File size: 44,043 Bytes
14f6f61 4088864 14f6f61 73fb27f 14f6f61 73fb27f 14f6f61 73fb27f 14f6f61 73fb27f 14f6f61 73fb27f 14f6f61 73fb27f 14f6f61 73fb27f 14f6f61 |
1 2 3 4 5 6 7 8 9 10 11 12 13 14 15 16 17 18 19 20 21 22 23 24 25 26 27 28 29 30 31 32 33 34 35 36 37 38 39 40 41 42 43 44 45 46 47 48 49 50 51 52 53 54 55 56 57 58 59 60 61 62 63 64 65 66 67 68 69 70 71 72 73 74 75 76 77 78 79 80 81 82 83 84 85 86 87 88 89 90 91 92 93 94 95 96 97 98 99 100 101 102 103 104 105 106 107 108 109 110 111 112 113 114 115 116 117 118 119 120 121 122 123 124 125 126 127 128 129 130 131 132 133 134 135 136 137 138 139 140 141 142 143 144 145 146 147 148 149 150 151 152 153 154 155 156 157 158 159 160 161 162 163 164 165 166 167 168 169 170 171 172 173 174 175 176 177 178 179 180 181 182 183 184 185 186 187 188 189 190 191 192 193 194 195 196 197 198 199 200 201 202 203 204 205 206 207 208 209 210 211 212 213 214 215 216 217 218 219 220 221 222 223 224 225 226 227 228 229 230 231 232 233 234 235 236 237 238 239 240 241 242 243 244 245 246 247 248 249 250 251 252 253 254 255 256 257 258 259 260 261 262 263 264 265 266 267 268 269 270 271 272 273 274 275 276 277 278 279 280 281 282 283 284 285 286 287 288 289 290 291 292 293 294 295 296 297 298 299 300 301 302 303 304 305 306 307 308 309 310 311 312 313 314 315 316 317 318 319 320 321 322 323 324 325 326 327 328 329 330 331 332 333 334 335 336 337 338 339 340 341 342 343 344 345 346 347 348 349 350 351 352 353 354 355 356 357 358 359 360 361 362 363 364 365 366 367 368 369 370 371 372 373 374 375 376 377 378 379 380 381 382 383 384 385 386 387 388 389 390 391 392 393 394 395 396 397 398 399 400 401 402 403 404 405 406 407 408 409 410 411 412 413 414 415 416 417 418 419 420 421 422 423 424 425 426 427 428 429 430 431 432 433 434 435 436 437 438 439 440 441 442 443 444 445 446 447 448 449 450 451 452 453 454 455 456 457 458 459 460 461 462 463 464 465 466 467 468 469 470 471 472 473 474 475 476 477 478 479 480 481 482 483 484 485 486 487 488 489 490 491 492 493 494 495 496 497 498 499 500 501 502 503 504 505 506 507 508 509 510 511 512 513 514 |
---
license: agpl-3.0
library_name: ultralytics
pipeline_tag: object-detection
---
<div align="center">
<p>
<a href="https://www.ultralytics.com/events/yolovision" target="_blank">
<img width="100%" src="https://raw.githubusercontent.com/ultralytics/assets/main/yolov8/banner-yolov8.png"></a>
</p>
[中文](https://docs.ultralytics.com/zh) | [한국어](https://docs.ultralytics.com/ko) | [日本語](https://docs.ultralytics.com/ja) | [Русский](https://docs.ultralytics.com/ru) | [Deutsch](https://docs.ultralytics.com/de) | [Français](https://docs.ultralytics.com/fr) | [Español](https://docs.ultralytics.com/es) | [Português](https://docs.ultralytics.com/pt) | [Türkçe](https://docs.ultralytics.com/tr) | [Tiếng Việt](https://docs.ultralytics.com/vi) | [العربية](https://docs.ultralytics.com/ar)
<div style="display: flex; justify-content: center; align-items: center; flex-wrap: wrap; gap: 20px; text-align: center; margin: 5px;">
<a href="https://github.com/ultralytics/yolov5/actions/workflows/ci-testing.yml">
<img src="https://github.com/ultralytics/yolov5/actions/workflows/ci-testing.yml/badge.svg" alt="YOLOv5 CI">
</a>
<a href="https://zenodo.org/badge/latestdoi/264818686">
<img src="https://zenodo.org/badge/264818686.svg" alt="YOLOv5 Citation">
</a>
<a href="https://hub.docker.com/r/ultralytics/yolov5">
<img src="https://img.shields.io/docker/pulls/ultralytics/yolov5?logo=docker" alt="Docker Pulls">
</a>
<a href="https://discord.com/invite/ultralytics">
<img alt="Discord" src="https://img.shields.io/discord/1089800235347353640?logo=discord&logoColor=white&label=Discord&color=blue">
</a>
<a href="https://community.ultralytics.com/">
<img alt="Ultralytics Forums" src="https://img.shields.io/discourse/users?server=https%3A%2F%2Fcommunity.ultralytics.com&logo=discourse&label=Forums&color=blue">
</a>
<a href="https://reddit.com/r/ultralytics">
<img alt="Ultralytics Reddit" src="https://img.shields.io/reddit/subreddit-subscribers/ultralytics?style=flat&logo=reddit&logoColor=white&label=Reddit&color=blue">
</a>
</div>
<div style="display: flex; justify-content: center; align-items: center; flex-wrap: wrap; gap: 20px; text-align: center; margin-top: 5px;">
<a href="https://bit.ly/yolov5-paperspace-notebook">
<img src="https://assets.paperspace.io/img/gradient-badge.svg" alt="Run on Gradient">
</a>
<a href="https://colab.research.google.com/github/ultralytics/yolov5/blob/master/tutorial.ipynb">
<img src="https://colab.research.google.com/assets/colab-badge.svg" alt="Open In Colab">
</a>
<a href="https://www.kaggle.com/models/ultralytics/yolov5">
<img src="https://kaggle.com/static/images/open-in-kaggle.svg" alt="Open In Kaggle">
</a>
</div>
YOLOv5 🚀 is the world's most loved vision AI, representing <a href="https://www.ultralytics.com/">Ultralytics</a> open-source research into future vision AI methods, incorporating lessons learned and best practices evolved over thousands of hours of research and development. We hope that the resources here will help you get the most out of YOLOv5. Please browse the YOLOv5 <a href="https://docs.ultralytics.com/yolov5/">Docs</a> for details, raise an issue on <a href="https://github.com/ultralytics/yolov5/issues/new/choose">GitHub</a> for support, and join our <a href="https://discord.com/invite/ultralytics">Discord</a> community for questions and discussions! To request an Enterprise License please complete the form at [Ultralytics Licensing](https://www.ultralytics.com/license).
<div style="display: flex; justify-content: center; align-items: center; gap: 10px; flex-wrap: wrap; margin-top: 20px;">
<a href="https://github.com/ultralytics">
<img src="https://github.com/ultralytics/assets/raw/main/social/logo-social-github.png" width="40px" alt="Ultralytics GitHub">
</a>
<img src="https://github.com/ultralytics/assets/raw/main/social/logo-transparent.png" width="40px">
<a href="https://www.linkedin.com/company/ultralytics/">
<img src="https://github.com/ultralytics/assets/raw/main/social/logo-social-linkedin.png" width="40px" alt="Ultralytics LinkedIn">
</a>
<img src="https://github.com/ultralytics/assets/raw/main/social/logo-transparent.png" width="40px">
<a href="https://twitter.com/ultralytics">
<img src="https://github.com/ultralytics/assets/raw/main/social/logo-social-twitter.png" width="40px" alt="Ultralytics Twitter">
</a>
<img src="https://github.com/ultralytics/assets/raw/main/social/logo-transparent.png" width="40px">
<a href="https://youtube.com/ultralytics?sub_confirmation=1">
<img src="https://github.com/ultralytics/assets/raw/main/social/logo-social-youtube.png" width="40px" alt="Ultralytics YouTube">
</a>
<img src="https://github.com/ultralytics/assets/raw/main/social/logo-transparent.png" width="40px">
<a href="https://www.tiktok.com/@ultralytics">
<img src="https://github.com/ultralytics/assets/raw/main/social/logo-social-tiktok.png" width="40px" alt="Ultralytics TikTok">
</a>
<img src="https://github.com/ultralytics/assets/raw/main/social/logo-transparent.png" width="40px">
<a href="https://ultralytics.com/bilibili">
<img src="https://github.com/ultralytics/assets/raw/main/social/logo-social-bilibili.png" width="40px" alt="Ultralytics BiliBili">
</a>
<img src="https://github.com/ultralytics/assets/raw/main/social/logo-transparent.png" width="40px">
<a href="https://discord.com/invite/ultralytics">
<img src="https://github.com/ultralytics/assets/raw/main/social/logo-social-discord.png" width="40px" alt="Ultralytics Discord">
</a>
</div>
<br>
<div align="center">
<h2>YOLO11 🚀 NEW</h2>
</div>
We are excited to unveil the launch of Ultralytics YOLO11 🚀, the latest advancement in our state-of-the-art (SOTA) vision models! Available now at **[GitHub](https://github.com/ultralytics/ultralytics)**, YOLO11 builds on our legacy of speed, precision, and ease of use. Whether you're tackling object detection, image segmentation, or image classification, YOLO11 delivers the performance and versatility needed to excel in diverse applications. Get started today and unlock the full potential of YOLO11! Visit the [Ultralytics Docs](https://docs.ultralytics.com/) for comprehensive guides and resources:
<div style="display: flex; justify-content: center; align-items: center; gap: 10px; margin-top: 20px;">
<a href="https://badge.fury.io/py/ultralytics">
<img src="https://badge.fury.io/py/ultralytics.svg" alt="PyPI version">
</a>
<a href="https://www.pepy.tech/projects/ultralytics">
<img src="https://static.pepy.tech/badge/ultralytics" alt="Downloads">
</a>
</div>
```bash
pip install ultralytics
```
<div align="center">
<a href="https://www.ultralytics.com/yolo" target="_blank">
<img width="100%" src="https://raw.githubusercontent.com/ultralytics/assets/refs/heads/main/yolo/performance-comparison.png"></a>
</div>
<div align="center">
<h2>Documentation</h2>
</div>
See the [YOLOv5 Docs](https://docs.ultralytics.com/yolov5/) for full documentation on training, testing and deployment. See below for quickstart examples.
<details open>
<summary>Install</summary>
Clone repo and install [requirements.txt](https://github.com/ultralytics/yolov5/blob/master/requirements.txt) in a [**Python>=3.8.0**](https://www.python.org/) environment, including [**PyTorch>=1.8**](https://pytorch.org/get-started/locally/).
```bash
git clone https://github.com/ultralytics/yolov5 # clone
cd yolov5
pip install -r requirements.txt # install
```
</details>
<details>
<summary>Inference</summary>
YOLOv5 [PyTorch Hub](https://docs.ultralytics.com/yolov5/tutorials/pytorch_hub_model_loading/) inference. [Models](https://github.com/ultralytics/yolov5/tree/master/models) download automatically from the latest YOLOv5 [release](https://github.com/ultralytics/yolov5/releases).
```python
import torch
# Model
model = torch.hub.load("ultralytics/yolov5", "yolov5s") # or yolov5n - yolov5x6, custom
# Images
img = "https://ultralytics.com/images/zidane.jpg" # or file, Path, PIL, OpenCV, numpy, list
# Inference
results = model(img)
# Results
results.print() # or .show(), .save(), .crop(), .pandas(), etc.
```
</details>
<details>
<summary>Inference with detect.py</summary>
`detect.py` runs inference on a variety of sources, downloading [models](https://github.com/ultralytics/yolov5/tree/master/models) automatically from the latest YOLOv5 [release](https://github.com/ultralytics/yolov5/releases) and saving results to `runs/detect`.
```bash
python detect.py --weights yolov5s.pt --source 0 # webcam
img.jpg # image
vid.mp4 # video
screen # screenshot
path/ # directory
list.txt # list of images
list.streams # list of streams
'path/*.jpg' # glob
'https://youtu.be/LNwODJXcvt4' # YouTube
'rtsp://example.com/media.mp4' # RTSP, RTMP, HTTP stream
```
</details>
<details>
<summary>Training</summary>
The commands below reproduce YOLOv5 [COCO](https://github.com/ultralytics/yolov5/blob/master/data/scripts/get_coco.sh) results. [Models](https://github.com/ultralytics/yolov5/tree/master/models) and [datasets](https://github.com/ultralytics/yolov5/tree/master/data) download automatically from the latest YOLOv5 [release](https://github.com/ultralytics/yolov5/releases). Training times for YOLOv5n/s/m/l/x are 1/2/4/6/8 days on a V100 GPU ([Multi-GPU](https://docs.ultralytics.com/yolov5/tutorials/multi_gpu_training/) times faster). Use the largest `--batch-size` possible, or pass `--batch-size -1` for YOLOv5 [AutoBatch](https://github.com/ultralytics/yolov5/pull/5092). Batch sizes shown for V100-16GB.
```bash
python train.py --data coco.yaml --epochs 300 --weights '' --cfg yolov5n.yaml --batch-size 128
yolov5s 64
yolov5m 40
yolov5l 24
yolov5x 16
```
<img width="800" src="https://user-images.githubusercontent.com/26833433/90222759-949d8800-ddc1-11ea-9fa1-1c97eed2b963.png">
</details>
<details open>
<summary>Tutorials</summary>
- [Train Custom Data](https://docs.ultralytics.com/yolov5/tutorials/train_custom_data/) 🚀 RECOMMENDED
- [Tips for Best Training Results](https://docs.ultralytics.com/guides/model-training-tips/) ☘️
- [Multi-GPU Training](https://docs.ultralytics.com/yolov5/tutorials/multi_gpu_training/)
- [PyTorch Hub](https://docs.ultralytics.com/yolov5/tutorials/pytorch_hub_model_loading/) 🌟 NEW
- [TFLite, ONNX, CoreML, TensorRT Export](https://docs.ultralytics.com/yolov5/tutorials/model_export/) 🚀
- [NVIDIA Jetson platform Deployment](https://docs.ultralytics.com/yolov5/tutorials/running_on_jetson_nano/) 🌟 NEW
- [Test-Time Augmentation (TTA)](https://docs.ultralytics.com/yolov5/tutorials/test_time_augmentation/)
- [Model Ensembling](https://docs.ultralytics.com/yolov5/tutorials/model_ensembling/)
- [Model Pruning/Sparsity](https://docs.ultralytics.com/yolov5/tutorials/model_pruning_and_sparsity/)
- [Hyperparameter Evolution](https://docs.ultralytics.com/yolov5/tutorials/hyperparameter_evolution/)
- [Transfer Learning with Frozen Layers](https://docs.ultralytics.com/yolov5/tutorials/transfer_learning_with_frozen_layers/)
- [Architecture Summary](https://docs.ultralytics.com/yolov5/tutorials/architecture_description/) 🌟 NEW
- [Ultralytics HUB to train and deploy YOLO](https://www.ultralytics.com/hub) 🚀 RECOMMENDED
- [ClearML Logging](https://docs.ultralytics.com/yolov5/tutorials/clearml_logging_integration/)
- [YOLOv5 with Neural Magic's Deepsparse](https://docs.ultralytics.com/yolov5/tutorials/neural_magic_pruning_quantization/)
- [Comet Logging](https://docs.ultralytics.com/yolov5/tutorials/comet_logging_integration/) 🌟 NEW
</details>
## <div align="center">Integrations</div>
Our key integrations with leading AI platforms extend the functionality of Ultralytics' offerings, enhancing tasks like dataset labeling, training, visualization, and model management. Discover how Ultralytics, in collaboration with [W&B](https://docs.wandb.ai/guides/integrations/ultralytics/), [Comet](https://bit.ly/yolov8-readme-comet), [Roboflow](https://roboflow.com/?ref=ultralytics) and [OpenVINO](https://docs.ultralytics.com/integrations/openvino/), can optimize your AI workflow.
<br>
<a href="https://www.ultralytics.com/hub" target="_blank">
<img width="100%" src="https://github.com/ultralytics/assets/raw/main/yolov8/banner-integrations.png" alt="Ultralytics active learning integrations"></a>
<br>
<br>
<div align="center">
<a href="https://www.ultralytics.com/hub">
<img src="https://github.com/ultralytics/assets/raw/main/partners/logo-ultralytics-hub.png" width="10%" alt="Ultralytics HUB logo"></a>
<img src="https://github.com/ultralytics/assets/raw/main/social/logo-transparent.png" width="15%" height="0" alt="space">
<a href="https://docs.wandb.ai/guides/integrations/ultralytics/">
<img src="https://github.com/ultralytics/assets/raw/main/partners/logo-wb.png" width="10%" alt="ClearML logo"></a>
<img src="https://github.com/ultralytics/assets/raw/main/social/logo-transparent.png" width="15%" height="0" alt="space">
<a href="https://bit.ly/yolov8-readme-comet">
<img src="https://github.com/ultralytics/assets/raw/main/partners/logo-comet.png" width="10%" alt="Comet ML logo"></a>
<img src="https://github.com/ultralytics/assets/raw/main/social/logo-transparent.png" width="15%" height="0" alt="space">
<a href="https://bit.ly/yolov5-neuralmagic">
<img src="https://github.com/ultralytics/assets/raw/main/partners/logo-neuralmagic.png" width="10%" alt="NeuralMagic logo"></a>
</div>
| Ultralytics HUB 🚀 | W&B | Comet ⭐ NEW | Neural Magic |
| :--------------------------------------------------------------------------------------------------------------------------------: | :-----------------------------------------------------------------------------------------------------------------------------: | :-------------------------------------------------------------------------------------------------------------------------------------------------------: | :----------------------------------------------------------------------------------------------------: |
| Streamline YOLO workflows: Label, train, and deploy effortlessly with [Ultralytics HUB](https://www.ultralytics.com/hub). Try now! | Track experiments, hyperparameters, and results with [Weights & Biases](https://docs.wandb.ai/guides/integrations/ultralytics/) | Free forever, [Comet](https://bit.ly/yolov5-readme-comet) lets you save YOLOv5 models, resume training, and interactively visualize and debug predictions | Run YOLO11 inference up to 6x faster with [Neural Magic DeepSparse](https://bit.ly/yolov5-neuralmagic) |
## <div align="center">Ultralytics HUB</div>
Experience seamless AI with [Ultralytics HUB](https://www.ultralytics.com/hub) ⭐, the all-in-one solution for data visualization, YOLOv5 and YOLOv8 🚀 model training and deployment, without any coding. Transform images into actionable insights and bring your AI visions to life with ease using our cutting-edge platform and user-friendly [Ultralytics App](https://www.ultralytics.com/app-install). Start your journey for **Free** now!
<a align="center" href="https://www.ultralytics.com/hub" target="_blank">
<img width="100%" src="https://github.com/ultralytics/assets/raw/main/im/ultralytics-hub.png"></a>
## <div align="center">Why YOLOv5</div>
YOLOv5 has been designed to be super easy to get started and simple to learn. We prioritize real-world results.
<p align="left"><img width="800" src="https://user-images.githubusercontent.com/26833433/155040763-93c22a27-347c-4e3c-847a-8094621d3f4e.png"></p>
<details>
<summary>YOLOv5-P5 640 Figure</summary>
<p align="left"><img width="800" src="https://user-images.githubusercontent.com/26833433/155040757-ce0934a3-06a6-43dc-a979-2edbbd69ea0e.png"></p>
</details>
<details>
<summary>Figure Notes</summary>
- **COCO AP val** denotes [email protected]:0.95 metric measured on the 5000-image [COCO val2017](http://cocodataset.org) dataset over various inference sizes from 256 to 1536.
- **GPU Speed** measures average inference time per image on [COCO val2017](http://cocodataset.org) dataset using a [AWS p3.2xlarge](https://aws.amazon.com/ec2/instance-types/p3/) V100 instance at batch-size 32.
- **EfficientDet** data from [google/automl](https://github.com/google/automl) at batch size 8.
- **Reproduce** by `python val.py --task study --data coco.yaml --iou 0.7 --weights yolov5n6.pt yolov5s6.pt yolov5m6.pt yolov5l6.pt yolov5x6.pt`
</details>
### Pretrained Checkpoints
| Model | size<br><sup>(pixels) | mAP<sup>val<br>50-95 | mAP<sup>val<br>50 | Speed<br><sup>CPU b1<br>(ms) | Speed<br><sup>V100 b1<br>(ms) | Speed<br><sup>V100 b32<br>(ms) | params<br><sup>(M) | FLOPs<br><sup>@640 (B) |
| ----------------------------------------------------------------------------------------------- | --------------------- | -------------------- | ----------------- | ---------------------------- | ----------------------------- | ------------------------------ | ------------------ | ---------------------- |
| [YOLOv5n](https://github.com/ultralytics/yolov5/releases/download/v7.0/yolov5n.pt) | 640 | 28.0 | 45.7 | **45** | **6.3** | **0.6** | **1.9** | **4.5** |
| [YOLOv5s](https://github.com/ultralytics/yolov5/releases/download/v7.0/yolov5s.pt) | 640 | 37.4 | 56.8 | 98 | 6.4 | 0.9 | 7.2 | 16.5 |
| [YOLOv5m](https://github.com/ultralytics/yolov5/releases/download/v7.0/yolov5m.pt) | 640 | 45.4 | 64.1 | 224 | 8.2 | 1.7 | 21.2 | 49.0 |
| [YOLOv5l](https://github.com/ultralytics/yolov5/releases/download/v7.0/yolov5l.pt) | 640 | 49.0 | 67.3 | 430 | 10.1 | 2.7 | 46.5 | 109.1 |
| [YOLOv5x](https://github.com/ultralytics/yolov5/releases/download/v7.0/yolov5x.pt) | 640 | 50.7 | 68.9 | 766 | 12.1 | 4.8 | 86.7 | 205.7 |
| | | | | | | | | |
| [YOLOv5n6](https://github.com/ultralytics/yolov5/releases/download/v7.0/yolov5n6.pt) | 1280 | 36.0 | 54.4 | 153 | 8.1 | 2.1 | 3.2 | 4.6 |
| [YOLOv5s6](https://github.com/ultralytics/yolov5/releases/download/v7.0/yolov5s6.pt) | 1280 | 44.8 | 63.7 | 385 | 8.2 | 3.6 | 12.6 | 16.8 |
| [YOLOv5m6](https://github.com/ultralytics/yolov5/releases/download/v7.0/yolov5m6.pt) | 1280 | 51.3 | 69.3 | 887 | 11.1 | 6.8 | 35.7 | 50.0 |
| [YOLOv5l6](https://github.com/ultralytics/yolov5/releases/download/v7.0/yolov5l6.pt) | 1280 | 53.7 | 71.3 | 1784 | 15.8 | 10.5 | 76.8 | 111.4 |
| [YOLOv5x6](https://github.com/ultralytics/yolov5/releases/download/v7.0/yolov5x6.pt)<br>+ [TTA] | 1280<br>1536 | 55.0<br>**55.8** | 72.7<br>**72.7** | 3136<br>- | 26.2<br>- | 19.4<br>- | 140.7<br>- | 209.8<br>- |
<details>
<summary>Table Notes</summary>
- All checkpoints are trained to 300 epochs with default settings. Nano and Small models use [hyp.scratch-low.yaml](https://github.com/ultralytics/yolov5/blob/master/data/hyps/hyp.scratch-low.yaml) hyps, all others use [hyp.scratch-high.yaml](https://github.com/ultralytics/yolov5/blob/master/data/hyps/hyp.scratch-high.yaml).
- **mAP<sup>val</sup>** values are for single-model single-scale on [COCO val2017](http://cocodataset.org) dataset.<br>Reproduce by `python val.py --data coco.yaml --img 640 --conf 0.001 --iou 0.65`
- **Speed** averaged over COCO val images using a [AWS p3.2xlarge](https://aws.amazon.com/ec2/instance-types/p3/) instance. NMS times (~1 ms/img) not included.<br>Reproduce by `python val.py --data coco.yaml --img 640 --task speed --batch 1`
- **TTA** [Test Time Augmentation](https://docs.ultralytics.com/yolov5/tutorials/test_time_augmentation/) includes reflection and scale augmentations.<br>Reproduce by `python val.py --data coco.yaml --img 1536 --iou 0.7 --augment`
</details>
## <div align="center">Segmentation</div>
Our new YOLOv5 [release v7.0](https://github.com/ultralytics/yolov5/releases/v7.0) instance segmentation models are the fastest and most accurate in the world, beating all current [SOTA benchmarks](https://paperswithcode.com/sota/real-time-instance-segmentation-on-mscoco). We've made them super simple to train, validate and deploy. See full details in our [Release Notes](https://github.com/ultralytics/yolov5/releases/v7.0) and visit our [YOLOv5 Segmentation Colab Notebook](https://github.com/ultralytics/yolov5/blob/master/segment/tutorial.ipynb) for quickstart tutorials.
<details>
<summary>Segmentation Checkpoints</summary>
<div align="center">
<a align="center" href="https://www.ultralytics.com/yolo" target="_blank">
<img width="800" src="https://user-images.githubusercontent.com/61612323/204180385-84f3aca9-a5e9-43d8-a617-dda7ca12e54a.png"></a>
</div>
We trained YOLOv5 segmentations models on COCO for 300 epochs at image size 640 using A100 GPUs. We exported all models to ONNX FP32 for CPU speed tests and to TensorRT FP16 for GPU speed tests. We ran all speed tests on Google [Colab Pro](https://colab.research.google.com/signup) notebooks for easy reproducibility.
| Model | size<br><sup>(pixels) | mAP<sup>box<br>50-95 | mAP<sup>mask<br>50-95 | Train time<br><sup>300 epochs<br>A100 (hours) | Speed<br><sup>ONNX CPU<br>(ms) | Speed<br><sup>TRT A100<br>(ms) | params<br><sup>(M) | FLOPs<br><sup>@640 (B) |
| ------------------------------------------------------------------------------------------ | --------------------- | -------------------- | --------------------- | --------------------------------------------- | ------------------------------ | ------------------------------ | ------------------ | ---------------------- |
| [YOLOv5n-seg](https://github.com/ultralytics/yolov5/releases/download/v7.0/yolov5n-seg.pt) | 640 | 27.6 | 23.4 | 80:17 | **62.7** | **1.2** | **2.0** | **7.1** |
| [YOLOv5s-seg](https://github.com/ultralytics/yolov5/releases/download/v7.0/yolov5s-seg.pt) | 640 | 37.6 | 31.7 | 88:16 | 173.3 | 1.4 | 7.6 | 26.4 |
| [YOLOv5m-seg](https://github.com/ultralytics/yolov5/releases/download/v7.0/yolov5m-seg.pt) | 640 | 45.0 | 37.1 | 108:36 | 427.0 | 2.2 | 22.0 | 70.8 |
| [YOLOv5l-seg](https://github.com/ultralytics/yolov5/releases/download/v7.0/yolov5l-seg.pt) | 640 | 49.0 | 39.9 | 66:43 (2x) | 857.4 | 2.9 | 47.9 | 147.7 |
| [YOLOv5x-seg](https://github.com/ultralytics/yolov5/releases/download/v7.0/yolov5x-seg.pt) | 640 | **50.7** | **41.4** | 62:56 (3x) | 1579.2 | 4.5 | 88.8 | 265.7 |
- All checkpoints are trained to 300 epochs with SGD optimizer with `lr0=0.01` and `weight_decay=5e-5` at image size 640 and all default settings.<br>Runs logged to https://wandb.ai/glenn-jocher/YOLOv5_v70_official
- **Accuracy** values are for single-model single-scale on COCO dataset.<br>Reproduce by `python segment/val.py --data coco.yaml --weights yolov5s-seg.pt`
- **Speed** averaged over 100 inference images using a [Colab Pro](https://colab.research.google.com/signup) A100 High-RAM instance. Values indicate inference speed only (NMS adds about 1ms per image). <br>Reproduce by `python segment/val.py --data coco.yaml --weights yolov5s-seg.pt --batch 1`
- **Export** to ONNX at FP32 and TensorRT at FP16 done with `export.py`. <br>Reproduce by `python export.py --weights yolov5s-seg.pt --include engine --device 0 --half`
</details>
<details>
<summary>Segmentation Usage Examples <a href="https://colab.research.google.com/github/ultralytics/yolov5/blob/master/segment/tutorial.ipynb"><img src="https://colab.research.google.com/assets/colab-badge.svg" alt="Open In Colab"></a></summary>
### Train
YOLOv5 segmentation training supports auto-download COCO128-seg segmentation dataset with `--data coco128-seg.yaml` argument and manual download of COCO-segments dataset with `bash data/scripts/get_coco.sh --train --val --segments` and then `python train.py --data coco.yaml`.
```bash
# Single-GPU
python segment/train.py --data coco128-seg.yaml --weights yolov5s-seg.pt --img 640
# Multi-GPU DDP
python -m torch.distributed.run --nproc_per_node 4 --master_port 1 segment/train.py --data coco128-seg.yaml --weights yolov5s-seg.pt --img 640 --device 0,1,2,3
```
### Val
Validate YOLOv5s-seg mask mAP on COCO dataset:
```bash
bash data/scripts/get_coco.sh --val --segments # download COCO val segments split (780MB, 5000 images)
python segment/val.py --weights yolov5s-seg.pt --data coco.yaml --img 640 # validate
```
### Predict
Use pretrained YOLOv5m-seg.pt to predict bus.jpg:
```bash
python segment/predict.py --weights yolov5m-seg.pt --source data/images/bus.jpg
```
```python
model = torch.hub.load(
"ultralytics/yolov5", "custom", "yolov5m-seg.pt"
) # load from PyTorch Hub (WARNING: inference not yet supported)
```
| 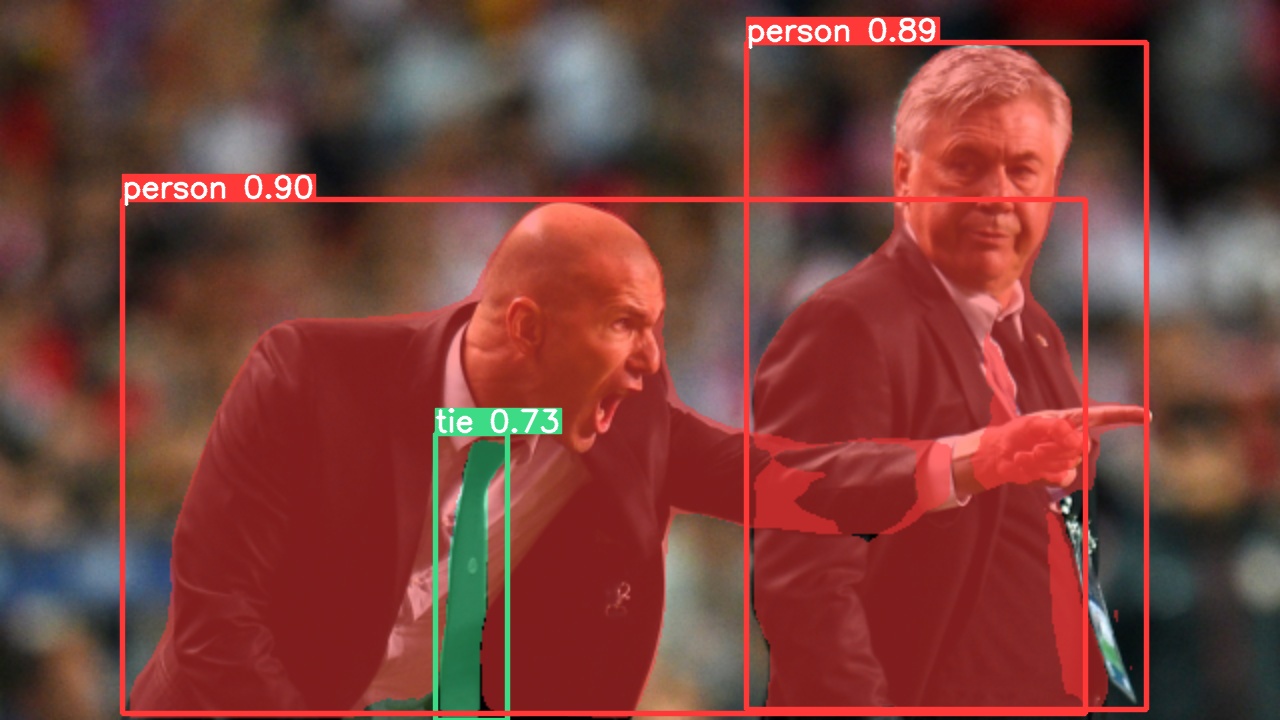 | 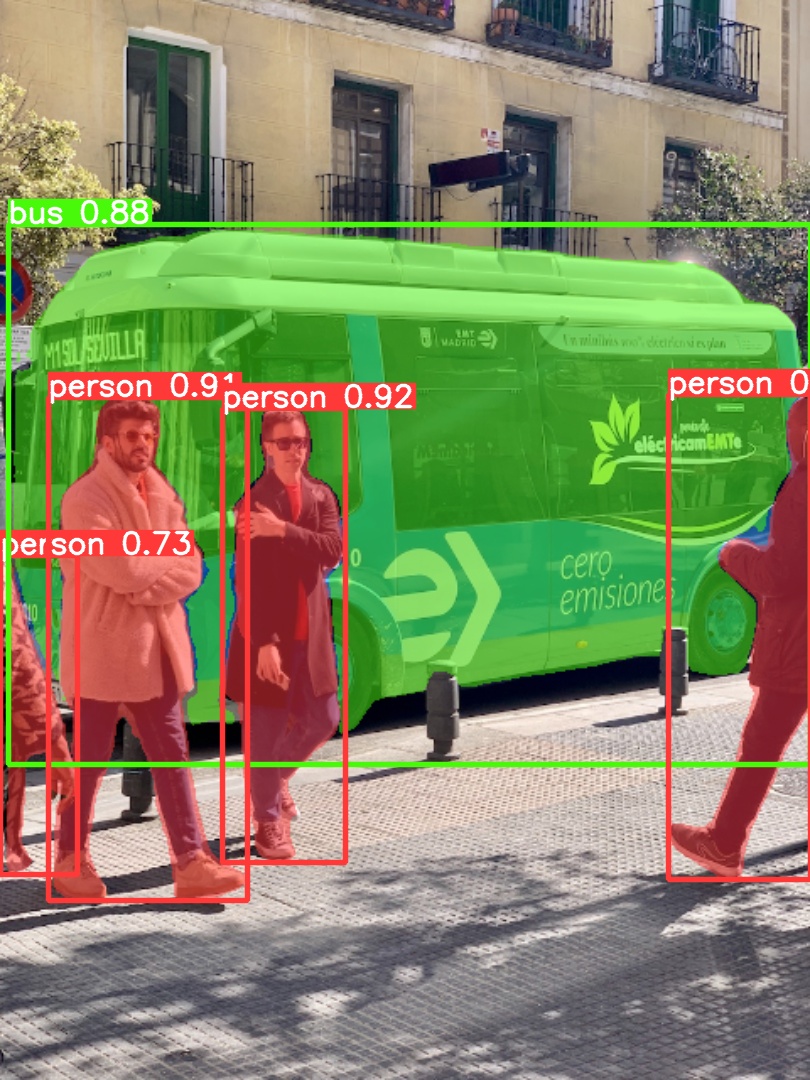 |
| ---------------------------------------------------------------------------------------------------------------- | ------------------------------------------------------------------------------------------------------------- |
### Export
Export YOLOv5s-seg model to ONNX and TensorRT:
```bash
python export.py --weights yolov5s-seg.pt --include onnx engine --img 640 --device 0
```
</details>
## <div align="center">Classification</div>
YOLOv5 [release v6.2](https://github.com/ultralytics/yolov5/releases) brings support for classification model training, validation and deployment! See full details in our [Release Notes](https://github.com/ultralytics/yolov5/releases/v6.2) and visit our [YOLOv5 Classification Colab Notebook](https://github.com/ultralytics/yolov5/blob/master/classify/tutorial.ipynb) for quickstart tutorials.
<details>
<summary>Classification Checkpoints</summary>
<br>
We trained YOLOv5-cls classification models on ImageNet for 90 epochs using a 4xA100 instance, and we trained ResNet and EfficientNet models alongside with the same default training settings to compare. We exported all models to ONNX FP32 for CPU speed tests and to TensorRT FP16 for GPU speed tests. We ran all speed tests on Google [Colab Pro](https://colab.research.google.com/signup) for easy reproducibility.
| Model | size<br><sup>(pixels) | acc<br><sup>top1 | acc<br><sup>top5 | Training<br><sup>90 epochs<br>4xA100 (hours) | Speed<br><sup>ONNX CPU<br>(ms) | Speed<br><sup>TensorRT V100<br>(ms) | params<br><sup>(M) | FLOPs<br><sup>@224 (B) |
| -------------------------------------------------------------------------------------------------- | --------------------- | ---------------- | ---------------- | -------------------------------------------- | ------------------------------ | ----------------------------------- | ------------------ | ---------------------- |
| [YOLOv5n-cls](https://github.com/ultralytics/yolov5/releases/download/v7.0/yolov5n-cls.pt) | 224 | 64.6 | 85.4 | 7:59 | **3.3** | **0.5** | **2.5** | **0.5** |
| [YOLOv5s-cls](https://github.com/ultralytics/yolov5/releases/download/v7.0/yolov5s-cls.pt) | 224 | 71.5 | 90.2 | 8:09 | 6.6 | 0.6 | 5.4 | 1.4 |
| [YOLOv5m-cls](https://github.com/ultralytics/yolov5/releases/download/v7.0/yolov5m-cls.pt) | 224 | 75.9 | 92.9 | 10:06 | 15.5 | 0.9 | 12.9 | 3.9 |
| [YOLOv5l-cls](https://github.com/ultralytics/yolov5/releases/download/v7.0/yolov5l-cls.pt) | 224 | 78.0 | 94.0 | 11:56 | 26.9 | 1.4 | 26.5 | 8.5 |
| [YOLOv5x-cls](https://github.com/ultralytics/yolov5/releases/download/v7.0/yolov5x-cls.pt) | 224 | **79.0** | **94.4** | 15:04 | 54.3 | 1.8 | 48.1 | 15.9 |
| | | | | | | | | |
| [ResNet18](https://github.com/ultralytics/yolov5/releases/download/v7.0/resnet18.pt) | 224 | 70.3 | 89.5 | **6:47** | 11.2 | 0.5 | 11.7 | 3.7 |
| [ResNet34](https://github.com/ultralytics/yolov5/releases/download/v7.0/resnet34.pt) | 224 | 73.9 | 91.8 | 8:33 | 20.6 | 0.9 | 21.8 | 7.4 |
| [ResNet50](https://github.com/ultralytics/yolov5/releases/download/v7.0/resnet50.pt) | 224 | 76.8 | 93.4 | 11:10 | 23.4 | 1.0 | 25.6 | 8.5 |
| [ResNet101](https://github.com/ultralytics/yolov5/releases/download/v7.0/resnet101.pt) | 224 | 78.5 | 94.3 | 17:10 | 42.1 | 1.9 | 44.5 | 15.9 |
| | | | | | | | | |
| [EfficientNet_b0](https://github.com/ultralytics/yolov5/releases/download/v7.0/efficientnet_b0.pt) | 224 | 75.1 | 92.4 | 13:03 | 12.5 | 1.3 | 5.3 | 1.0 |
| [EfficientNet_b1](https://github.com/ultralytics/yolov5/releases/download/v7.0/efficientnet_b1.pt) | 224 | 76.4 | 93.2 | 17:04 | 14.9 | 1.6 | 7.8 | 1.5 |
| [EfficientNet_b2](https://github.com/ultralytics/yolov5/releases/download/v7.0/efficientnet_b2.pt) | 224 | 76.6 | 93.4 | 17:10 | 15.9 | 1.6 | 9.1 | 1.7 |
| [EfficientNet_b3](https://github.com/ultralytics/yolov5/releases/download/v7.0/efficientnet_b3.pt) | 224 | 77.7 | 94.0 | 19:19 | 18.9 | 1.9 | 12.2 | 2.4 |
<details>
<summary>Table Notes (click to expand)</summary>
- All checkpoints are trained to 90 epochs with SGD optimizer with `lr0=0.001` and `weight_decay=5e-5` at image size 224 and all default settings.<br>Runs logged to https://wandb.ai/glenn-jocher/YOLOv5-Classifier-v6-2
- **Accuracy** values are for single-model single-scale on [ImageNet-1k](https://www.image-net.org/index.php) dataset.<br>Reproduce by `python classify/val.py --data ../datasets/imagenet --img 224`
- **Speed** averaged over 100 inference images using a Google [Colab Pro](https://colab.research.google.com/signup) V100 High-RAM instance.<br>Reproduce by `python classify/val.py --data ../datasets/imagenet --img 224 --batch 1`
- **Export** to ONNX at FP32 and TensorRT at FP16 done with `export.py`. <br>Reproduce by `python export.py --weights yolov5s-cls.pt --include engine onnx --imgsz 224`
</details>
</details>
<details>
<summary>Classification Usage Examples <a href="https://colab.research.google.com/github/ultralytics/yolov5/blob/master/classify/tutorial.ipynb"><img src="https://colab.research.google.com/assets/colab-badge.svg" alt="Open In Colab"></a></summary>
### Train
YOLOv5 classification training supports auto-download of MNIST, Fashion-MNIST, CIFAR10, CIFAR100, Imagenette, Imagewoof, and ImageNet datasets with the `--data` argument. To start training on MNIST for example use `--data mnist`.
```bash
# Single-GPU
python classify/train.py --model yolov5s-cls.pt --data cifar100 --epochs 5 --img 224 --batch 128
# Multi-GPU DDP
python -m torch.distributed.run --nproc_per_node 4 --master_port 1 classify/train.py --model yolov5s-cls.pt --data imagenet --epochs 5 --img 224 --device 0,1,2,3
```
### Val
Validate YOLOv5m-cls accuracy on ImageNet-1k dataset:
```bash
bash data/scripts/get_imagenet.sh --val # download ImageNet val split (6.3G, 50000 images)
python classify/val.py --weights yolov5m-cls.pt --data ../datasets/imagenet --img 224 # validate
```
### Predict
Use pretrained YOLOv5s-cls.pt to predict bus.jpg:
```bash
python classify/predict.py --weights yolov5s-cls.pt --source data/images/bus.jpg
```
```python
model = torch.hub.load("ultralytics/yolov5", "custom", "yolov5s-cls.pt") # load from PyTorch Hub
```
### Export
Export a group of trained YOLOv5s-cls, ResNet and EfficientNet models to ONNX and TensorRT:
```bash
python export.py --weights yolov5s-cls.pt resnet50.pt efficientnet_b0.pt --include onnx engine --img 224
```
</details>
## <div align="center">Environments</div>
Get started in seconds with our verified environments. Click each icon below for details.
<div align="center">
<a href="https://bit.ly/yolov5-paperspace-notebook">
<img src="https://github.com/ultralytics/assets/releases/download/v0.0.0/logo-gradient.png" width="10%" /></a>
<img src="https://github.com/ultralytics/assets/raw/main/social/logo-transparent.png" width="5%" alt="" />
<a href="https://colab.research.google.com/github/ultralytics/yolov5/blob/master/tutorial.ipynb">
<img src="https://github.com/ultralytics/assets/releases/download/v0.0.0/logo-colab-small.png" width="10%" /></a>
<img src="https://github.com/ultralytics/assets/raw/main/social/logo-transparent.png" width="5%" alt="" />
<a href="https://www.kaggle.com/models/ultralytics/yolov5">
<img src="https://github.com/ultralytics/assets/releases/download/v0.0.0/logo-kaggle-small.png" width="10%" /></a>
<img src="https://github.com/ultralytics/assets/raw/main/social/logo-transparent.png" width="5%" alt="" />
<a href="https://hub.docker.com/r/ultralytics/yolov5">
<img src="https://github.com/ultralytics/assets/releases/download/v0.0.0/logo-docker-small.png" width="10%" /></a>
<img src="https://github.com/ultralytics/assets/raw/main/social/logo-transparent.png" width="5%" alt="" />
<a href="https://docs.ultralytics.com/yolov5/environments/aws_quickstart_tutorial/">
<img src="https://github.com/ultralytics/assets/releases/download/v0.0.0/logo-aws-small.png" width="10%" /></a>
<img src="https://github.com/ultralytics/assets/raw/main/social/logo-transparent.png" width="5%" alt="" />
<a href="https://docs.ultralytics.com/yolov5/environments/google_cloud_quickstart_tutorial/">
<img src="https://github.com/ultralytics/assets/releases/download/v0.0.0/logo-gcp-small.png" width="10%" /></a>
</div>
## <div align="center">Contribute</div>
We love your input! We want to make contributing to YOLOv5 as easy and transparent as possible. Please see our [Contributing Guide](https://docs.ultralytics.com/help/contributing/) to get started, and fill out the [YOLOv5 Survey](https://www.ultralytics.com/survey?utm_source=github&utm_medium=social&utm_campaign=Survey) to send us feedback on your experiences. Thank you to all our contributors!
<!-- SVG image from https://opencollective.com/ultralytics/contributors.svg?width=990 -->
<a href="https://github.com/ultralytics/yolov5/graphs/contributors">
<img src="https://github.com/ultralytics/assets/raw/main/im/image-contributors.png" /></a>
## <div align="center">License</div>
Ultralytics offers two licensing options to accommodate diverse use cases:
- **AGPL-3.0 License**: This [OSI-approved](https://opensource.org/license) open-source license is ideal for students and enthusiasts, promoting open collaboration and knowledge sharing. See the [LICENSE](https://github.com/ultralytics/yolov5/blob/master/LICENSE) file for more details.
- **Enterprise License**: Designed for commercial use, this license permits seamless integration of Ultralytics software and AI models into commercial goods and services, bypassing the open-source requirements of AGPL-3.0. If your scenario involves embedding our solutions into a commercial offering, reach out through [Ultralytics Licensing](https://www.ultralytics.com/license).
## <div align="center">Contact</div>
For YOLOv5 bug reports and feature requests please visit [GitHub Issues](https://github.com/ultralytics/yolov5/issues), and join our [Discord](https://discord.com/invite/ultralytics) community for questions and discussions!
<br>
<div align="center">
<a href="https://github.com/ultralytics"><img src="https://github.com/ultralytics/assets/raw/main/social/logo-social-github.png" width="3%" alt="Ultralytics GitHub"></a>
<img src="https://github.com/ultralytics/assets/raw/main/social/logo-transparent.png" width="3%">
<a href="https://www.linkedin.com/company/ultralytics/"><img src="https://github.com/ultralytics/assets/raw/main/social/logo-social-linkedin.png" width="3%" alt="Ultralytics LinkedIn"></a>
<img src="https://github.com/ultralytics/assets/raw/main/social/logo-transparent.png" width="3%">
<a href="https://twitter.com/ultralytics"><img src="https://github.com/ultralytics/assets/raw/main/social/logo-social-twitter.png" width="3%" alt="Ultralytics Twitter"></a>
<img src="https://github.com/ultralytics/assets/raw/main/social/logo-transparent.png" width="3%">
<a href="https://youtube.com/ultralytics?sub_confirmation=1"><img src="https://github.com/ultralytics/assets/raw/main/social/logo-social-youtube.png" width="3%" alt="Ultralytics YouTube"></a>
<img src="https://github.com/ultralytics/assets/raw/main/social/logo-transparent.png" width="3%">
<a href="https://www.tiktok.com/@ultralytics"><img src="https://github.com/ultralytics/assets/raw/main/social/logo-social-tiktok.png" width="3%" alt="Ultralytics TikTok"></a>
<img src="https://github.com/ultralytics/assets/raw/main/social/logo-transparent.png" width="3%">
<a href="https://ultralytics.com/bilibili"><img src="https://github.com/ultralytics/assets/raw/main/social/logo-social-bilibili.png" width="3%" alt="Ultralytics BiliBili"></a>
<img src="https://github.com/ultralytics/assets/raw/main/social/logo-transparent.png" width="3%">
<a href="https://discord.com/invite/ultralytics"><img src="https://github.com/ultralytics/assets/raw/main/social/logo-social-discord.png" width="3%" alt="Ultralytics Discord"></a>
</div>
[tta]: https://docs.ultralytics.com/yolov5/tutorials/test_time_augmentation |