Update README.md
Browse files
README.md
CHANGED
@@ -1,203 +1,95 @@
|
|
1 |
---
|
2 |
library_name: peft
|
3 |
base_model: google/flan-t5-large
|
|
|
|
|
|
|
|
|
|
|
|
|
|
|
|
|
|
|
|
|
|
|
|
|
4 |
---
|
5 |
|
6 |
# Model Card for Model ID
|
7 |
|
8 |
-
|
9 |
|
|
|
|
|
10 |
|
|
|
11 |
|
12 |
-
|
13 |
-
|
14 |
-
### Model Description
|
15 |
|
16 |
-
|
17 |
|
|
|
18 |
|
|
|
19 |
|
20 |
-
|
21 |
-
- **Funded by [optional]:** [More Information Needed]
|
22 |
-
- **Shared by [optional]:** [More Information Needed]
|
23 |
-
- **Model type:** [More Information Needed]
|
24 |
-
- **Language(s) (NLP):** [More Information Needed]
|
25 |
-
- **License:** [More Information Needed]
|
26 |
-
- **Finetuned from model [optional]:** [More Information Needed]
|
27 |
-
|
28 |
-
### Model Sources [optional]
|
29 |
|
30 |
-
|
|
|
|
|
31 |
|
32 |
-
- **
|
33 |
-
- **
|
34 |
-
- **Demo [optional]:** [More Information Needed]
|
35 |
|
36 |
## Uses
|
37 |
|
38 |
-
<!-- Address questions around how the model is intended to be used, including the foreseeable users of the model and those affected by the model. -->
|
39 |
-
|
40 |
### Direct Use
|
41 |
|
42 |
-
|
43 |
-
|
44 |
-
[More Information Needed]
|
45 |
-
|
46 |
-
### Downstream Use [optional]
|
47 |
-
|
48 |
-
<!-- This section is for the model use when fine-tuned for a task, or when plugged into a larger ecosystem/app -->
|
49 |
|
50 |
-
[More Information Needed]
|
51 |
-
|
52 |
-
### Out-of-Scope Use
|
53 |
-
|
54 |
-
<!-- This section addresses misuse, malicious use, and uses that the model will not work well for. -->
|
55 |
-
|
56 |
-
[More Information Needed]
|
57 |
-
|
58 |
-
## Bias, Risks, and Limitations
|
59 |
-
|
60 |
-
<!-- This section is meant to convey both technical and sociotechnical limitations. -->
|
61 |
-
|
62 |
-
[More Information Needed]
|
63 |
-
|
64 |
-
### Recommendations
|
65 |
-
|
66 |
-
<!-- This section is meant to convey recommendations with respect to the bias, risk, and technical limitations. -->
|
67 |
-
|
68 |
-
Users (both direct and downstream) should be made aware of the risks, biases and limitations of the model. More information needed for further recommendations.
|
69 |
|
70 |
## How to Get Started with the Model
|
71 |
|
72 |
Use the code below to get started with the model.
|
73 |
|
74 |
-
|
|
|
|
|
|
|
|
|
|
|
|
|
|
|
|
|
|
|
|
|
|
|
|
|
|
|
|
|
|
|
|
|
|
|
|
|
|
|
|
|
|
|
|
|
|
|
|
|
|
|
|
|
|
|
|
|
|
|
|
|
75 |
|
76 |
## Training Details
|
77 |
|
78 |
### Training Data
|
79 |
|
80 |
-
|
81 |
-
|
82 |
-
[More Information Needed]
|
83 |
-
|
84 |
-
### Training Procedure
|
85 |
-
|
86 |
-
<!-- This relates heavily to the Technical Specifications. Content here should link to that section when it is relevant to the training procedure. -->
|
87 |
-
|
88 |
-
#### Preprocessing [optional]
|
89 |
-
|
90 |
-
[More Information Needed]
|
91 |
-
|
92 |
-
|
93 |
-
#### Training Hyperparameters
|
94 |
-
|
95 |
-
- **Training regime:** [More Information Needed] <!--fp32, fp16 mixed precision, bf16 mixed precision, bf16 non-mixed precision, fp16 non-mixed precision, fp8 mixed precision -->
|
96 |
-
|
97 |
-
#### Speeds, Sizes, Times [optional]
|
98 |
-
|
99 |
-
<!-- This section provides information about throughput, start/end time, checkpoint size if relevant, etc. -->
|
100 |
-
|
101 |
-
[More Information Needed]
|
102 |
-
|
103 |
-
## Evaluation
|
104 |
-
|
105 |
-
<!-- This section describes the evaluation protocols and provides the results. -->
|
106 |
-
|
107 |
-
### Testing Data, Factors & Metrics
|
108 |
-
|
109 |
-
#### Testing Data
|
110 |
-
|
111 |
-
<!-- This should link to a Dataset Card if possible. -->
|
112 |
-
|
113 |
-
[More Information Needed]
|
114 |
-
|
115 |
-
#### Factors
|
116 |
-
|
117 |
-
<!-- These are the things the evaluation is disaggregating by, e.g., subpopulations or domains. -->
|
118 |
-
|
119 |
-
[More Information Needed]
|
120 |
-
|
121 |
-
#### Metrics
|
122 |
-
|
123 |
-
<!-- These are the evaluation metrics being used, ideally with a description of why. -->
|
124 |
-
|
125 |
-
[More Information Needed]
|
126 |
-
|
127 |
-
### Results
|
128 |
-
|
129 |
-
[More Information Needed]
|
130 |
-
|
131 |
-
#### Summary
|
132 |
-
|
133 |
-
|
134 |
-
|
135 |
-
## Model Examination [optional]
|
136 |
-
|
137 |
-
<!-- Relevant interpretability work for the model goes here -->
|
138 |
-
|
139 |
-
[More Information Needed]
|
140 |
-
|
141 |
-
## Environmental Impact
|
142 |
-
|
143 |
-
<!-- Total emissions (in grams of CO2eq) and additional considerations, such as electricity usage, go here. Edit the suggested text below accordingly -->
|
144 |
-
|
145 |
-
Carbon emissions can be estimated using the [Machine Learning Impact calculator](https://mlco2.github.io/impact#compute) presented in [Lacoste et al. (2019)](https://arxiv.org/abs/1910.09700).
|
146 |
-
|
147 |
-
- **Hardware Type:** [More Information Needed]
|
148 |
-
- **Hours used:** [More Information Needed]
|
149 |
-
- **Cloud Provider:** [More Information Needed]
|
150 |
-
- **Compute Region:** [More Information Needed]
|
151 |
-
- **Carbon Emitted:** [More Information Needed]
|
152 |
-
|
153 |
-
## Technical Specifications [optional]
|
154 |
-
|
155 |
-
### Model Architecture and Objective
|
156 |
-
|
157 |
-
[More Information Needed]
|
158 |
-
|
159 |
-
### Compute Infrastructure
|
160 |
-
|
161 |
-
[More Information Needed]
|
162 |
-
|
163 |
-
#### Hardware
|
164 |
-
|
165 |
-
[More Information Needed]
|
166 |
-
|
167 |
-
#### Software
|
168 |
-
|
169 |
-
[More Information Needed]
|
170 |
-
|
171 |
-
## Citation [optional]
|
172 |
-
|
173 |
-
<!-- If there is a paper or blog post introducing the model, the APA and Bibtex information for that should go in this section. -->
|
174 |
-
|
175 |
-
**BibTeX:**
|
176 |
-
|
177 |
-
[More Information Needed]
|
178 |
-
|
179 |
-
**APA:**
|
180 |
-
|
181 |
-
[More Information Needed]
|
182 |
-
|
183 |
-
## Glossary [optional]
|
184 |
-
|
185 |
-
<!-- If relevant, include terms and calculations in this section that can help readers understand the model or model card. -->
|
186 |
-
|
187 |
-
[More Information Needed]
|
188 |
-
|
189 |
-
## More Information [optional]
|
190 |
-
|
191 |
-
[More Information Needed]
|
192 |
-
|
193 |
-
## Model Card Authors [optional]
|
194 |
-
|
195 |
-
[More Information Needed]
|
196 |
-
|
197 |
-
## Model Card Contact
|
198 |
-
|
199 |
-
[More Information Needed]
|
200 |
-
|
201 |
|
202 |
### Framework versions
|
203 |
|
|
|
1 |
---
|
2 |
library_name: peft
|
3 |
base_model: google/flan-t5-large
|
4 |
+
license: creativeml-openrail-m
|
5 |
+
datasets:
|
6 |
+
- MohamedRashad/ChatGPT-prompts
|
7 |
+
- Hello-SimpleAI/HC3
|
8 |
+
language:
|
9 |
+
- en
|
10 |
+
metrics:
|
11 |
+
- accuracy
|
12 |
+
- rouge
|
13 |
+
pipeline_tag: text2text-generation
|
14 |
+
tags:
|
15 |
+
- prompt
|
16 |
---
|
17 |
|
18 |
# Model Card for Model ID
|
19 |
|
20 |
+
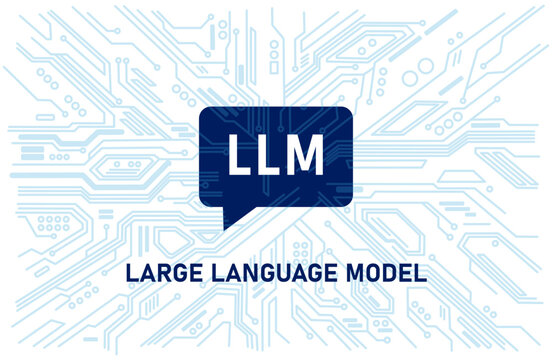
|
21 |
|
22 |
+
This exploration highlights the innovative use of the Learning Rate Annealing (LoRA) technique in the context of fine-tuning a T5 model. Based on the `google/flan-t5-large` architecture and utilizing the PEFT library, this approach aims to refine the model's capabilities specifically for question-answering (QA) tasks.
|
23 |
+
The entire fine-tuning code is available on Kaggle at the following link: [Kaggle code link](https://www.kaggle.com/code/yannicksteph/nlp-llm-fine-tuning-qa-lora-t5).
|
24 |
|
25 |
+
The exploration focuses on the fine-tuning methodology, leveraging LoRA to dynamically adjust the learning rate during the training process. This strategic choice aims to optimize the model's convergence and enhance its performance specifically for text generation tasks in response to questions.
|
26 |
|
27 |
+
The utilized datasets, such as `MohamedRashad/ChatGPT-prompts` and `Hello-SimpleAI/HC3`, contribute to enriching the diversity and complexity of linguistic interactions, thereby strengthening the model's ability to adapt to varied conversational contexts.
|
|
|
|
|
28 |
|
29 |
+
The resulting model, identified by the specified model ID, is intended for direct use in text generation scenarios while also offering the possibility of additional fine-tuning for specific tasks. Evaluation metrics, including accuracy and ROUGE score, provide an objective assessment of the model's performance.
|
30 |
|
31 |
+
To facilitate accessibility and usage, the entire fine-tuning code is available on Kaggle, serving as a practical and transparent resource for the natural language processing (NLP) practitioner community.
|
32 |
|
33 |
+
## Model Details
|
34 |
|
35 |
+
### Model Description
|
|
|
|
|
|
|
|
|
|
|
|
|
|
|
|
|
36 |
|
37 |
+
The model is based on the T5 architecture (google/flan-t5-large) and has undergone fine-tuning using the PEFT library.
|
38 |
+
It is designed to generate text responses in a question-answering format.
|
39 |
+
The model is available under the Creative Commons Attribution-ShareAlike 4.0 International License (creativeml-openrail-m).
|
40 |
|
41 |
+
- **Developed by:** [YanSte](https://github.com/YanSte)
|
42 |
+
- **Model type:** [flan-t5-large]
|
|
|
43 |
|
44 |
## Uses
|
45 |
|
|
|
|
|
46 |
### Direct Use
|
47 |
|
48 |
+
The model can be directly employed for text-to-text generation tasks, with a focus on generating responses to questions in a conversational format.
|
|
|
|
|
|
|
|
|
|
|
|
|
49 |
|
|
|
|
|
|
|
|
|
|
|
|
|
|
|
|
|
|
|
|
|
|
|
|
|
|
|
|
|
|
|
|
|
|
|
|
|
|
|
50 |
|
51 |
## How to Get Started with the Model
|
52 |
|
53 |
Use the code below to get started with the model.
|
54 |
|
55 |
+
```python
|
56 |
+
# Importing necessary libraries
|
57 |
+
from transformers import AutoTokenizer, T5ForConditionalGeneration
|
58 |
+
from transformers import pipeline
|
59 |
+
|
60 |
+
# Load the pre-trained tokenizer and fine-tuned model from the specified hub repository
|
61 |
+
tokenizer = AutoTokenizer.from_pretrained(hub_repo_name)
|
62 |
+
finetuned_model = T5ForConditionalGeneration.from_pretrained(hub_repo_name)
|
63 |
+
|
64 |
+
# Create a text generation pipeline using the fine-tuned model
|
65 |
+
text_generation_pipeline = pipeline(
|
66 |
+
task=pipeline_task,
|
67 |
+
model=finetuned_model,
|
68 |
+
tokenizer=tokenizer,
|
69 |
+
truncation=True,
|
70 |
+
max_length=pipeline_max_length,
|
71 |
+
min_length=pipeline_min_length,
|
72 |
+
temperature=pipeline_temperature,
|
73 |
+
device=0 # Set device to 0 for GPU, -1 for CPU
|
74 |
+
)
|
75 |
+
|
76 |
+
# Define a list of questions for text generation
|
77 |
+
questions = ["What is Sherlock Holmes' job?"]
|
78 |
+
|
79 |
+
# Prefix each question with the specified prefix for the task
|
80 |
+
prefix = "Answer this question: "
|
81 |
+
transformed_questions = [prefix + question for question in questions]
|
82 |
+
|
83 |
+
# Generate texts using the text generation pipeline with the transformed questions
|
84 |
+
generated_texts = text_generation_pipeline(transformed_questions, do_sample=True)
|
85 |
+
```
|
86 |
|
87 |
## Training Details
|
88 |
|
89 |
### Training Data
|
90 |
|
91 |
+
The model has been fine-tuned on datasets such as MohamedRashad/ChatGPT-prompts and Hello-SimpleAI/HC3.
|
92 |
+
More detailed information on the training data, including links to Dataset Cards and preprocessing details, is needed.
|
|
|
|
|
|
|
|
|
|
|
|
|
|
|
|
|
|
|
|
|
|
|
|
|
|
|
|
|
|
|
|
|
|
|
|
|
|
|
|
|
|
|
|
|
|
|
|
|
|
|
|
|
|
|
|
|
|
|
|
|
|
|
|
|
|
|
|
|
|
|
|
|
|
|
|
|
|
|
|
|
|
|
|
|
|
|
|
|
|
|
|
|
|
|
|
|
|
|
|
|
|
|
|
|
|
|
|
|
|
|
|
|
|
|
|
|
|
|
|
|
|
|
|
|
|
|
|
|
|
|
|
|
|
|
|
|
|
|
|
|
|
|
|
|
|
|
|
|
|
|
|
|
|
|
|
|
|
|
|
|
|
|
|
|
|
|
|
|
|
|
|
|
|
|
|
|
|
|
|
|
|
|
|
|
|
|
|
|
|
|
|
|
|
|
|
|
|
|
|
|
|
|
|
|
|
|
|
|
|
|
|
|
|
|
|
|
|
|
|
|
|
|
|
|
|
|
|
|
|
|
|
|
|
|
|
|
|
|
93 |
|
94 |
### Framework versions
|
95 |
|