File size: 7,596 Bytes
6712da4 627ebfc 6712da4 627ebfc 6712da4 627ebfc |
1 2 3 4 5 6 7 8 9 10 11 12 13 14 15 16 17 18 19 20 21 22 23 24 25 26 27 28 29 30 31 32 33 34 35 36 37 38 39 40 41 42 43 44 45 46 47 48 49 50 51 52 53 54 55 56 57 58 59 60 61 62 63 64 65 66 67 68 69 70 71 72 73 74 75 76 77 78 79 80 81 82 83 84 85 86 87 88 89 90 91 92 93 94 95 96 97 98 99 100 101 102 103 104 105 106 107 108 109 110 111 112 113 114 115 116 117 118 119 120 121 122 123 124 125 126 127 128 129 130 131 132 133 134 135 136 137 138 139 140 141 142 143 144 145 146 147 148 149 150 151 152 153 154 155 156 157 158 159 160 161 162 163 164 165 166 167 168 169 170 171 172 173 174 175 176 177 178 179 180 181 182 183 184 185 186 187 188 189 190 191 192 193 194 195 196 197 198 199 |
---
language:
- en
license: cc-by-4.0
pipeline_tag: text-generation
model-index:
- name: sqlcoder-34b-alpha
results:
- task:
type: text-generation
name: Text Generation
dataset:
name: AI2 Reasoning Challenge (25-Shot)
type: ai2_arc
config: ARC-Challenge
split: test
args:
num_few_shot: 25
metrics:
- type: acc_norm
value: 54.18
name: normalized accuracy
source:
url: https://huggingface.co/spaces/HuggingFaceH4/open_llm_leaderboard?query=defog/sqlcoder-34b-alpha
name: Open LLM Leaderboard
- task:
type: text-generation
name: Text Generation
dataset:
name: HellaSwag (10-Shot)
type: hellaswag
split: validation
args:
num_few_shot: 10
metrics:
- type: acc_norm
value: 75.93
name: normalized accuracy
source:
url: https://huggingface.co/spaces/HuggingFaceH4/open_llm_leaderboard?query=defog/sqlcoder-34b-alpha
name: Open LLM Leaderboard
- task:
type: text-generation
name: Text Generation
dataset:
name: MMLU (5-Shot)
type: cais/mmlu
config: all
split: test
args:
num_few_shot: 5
metrics:
- type: acc
value: 54.42
name: accuracy
source:
url: https://huggingface.co/spaces/HuggingFaceH4/open_llm_leaderboard?query=defog/sqlcoder-34b-alpha
name: Open LLM Leaderboard
- task:
type: text-generation
name: Text Generation
dataset:
name: TruthfulQA (0-shot)
type: truthful_qa
config: multiple_choice
split: validation
args:
num_few_shot: 0
metrics:
- type: mc2
value: 40.63
source:
url: https://huggingface.co/spaces/HuggingFaceH4/open_llm_leaderboard?query=defog/sqlcoder-34b-alpha
name: Open LLM Leaderboard
- task:
type: text-generation
name: Text Generation
dataset:
name: Winogrande (5-shot)
type: winogrande
config: winogrande_xl
split: validation
args:
num_few_shot: 5
metrics:
- type: acc
value: 73.48
name: accuracy
source:
url: https://huggingface.co/spaces/HuggingFaceH4/open_llm_leaderboard?query=defog/sqlcoder-34b-alpha
name: Open LLM Leaderboard
- task:
type: text-generation
name: Text Generation
dataset:
name: GSM8k (5-shot)
type: gsm8k
config: main
split: test
args:
num_few_shot: 5
metrics:
- type: acc
value: 34.87
name: accuracy
source:
url: https://huggingface.co/spaces/HuggingFaceH4/open_llm_leaderboard?query=defog/sqlcoder-34b-alpha
name: Open LLM Leaderboard
---
# Defog SQLCoder
**Updated on Nov 14 to reflect benchmarks for SQLCoder-34B**
Defog's SQLCoder is a state-of-the-art LLM for converting natural language questions to SQL queries.
[Interactive Demo](https://defog.ai/sqlcoder-demo/) | [🤗 HF Repo](https://huggingface.co/defog/sqlcoder-34b-alpha) | [♾️ Colab](https://colab.research.google.com/drive/1z4rmOEiFkxkMiecAWeTUlPl0OmKgfEu7?usp=sharing) | [🐦 Twitter](https://twitter.com/defogdata)
## TL;DR
SQLCoder-34B is a 34B parameter model that outperforms `gpt-4` and `gpt-4-turbo` for natural language to SQL generation tasks on our [sql-eval](https://github.com/defog-ai/sql-eval) framework, and significantly outperforms all popular open-source models.
SQLCoder-34B is fine-tuned on a base CodeLlama model.
## Results on novel datasets not seen in training
| model | perc_correct |
|-|-|
| defog-sqlcoder-34b | 84.0 |
| gpt4-turbo-2023-11-09 | 82.5 |
| gpt4-2023-11-09 | 82.5 |
| defog-sqlcoder2 | 77.5 |
| gpt4-2023-08-28 | 74.0 |
| defog-sqlcoder-7b | 71.0 |
| gpt-3.5-2023-10-04 | 66.0 |
| claude-2 | 64.5 |
| gpt-3.5-2023-08-28 | 61.0 |
| claude_instant_1 | 61.0 |
| text-davinci-003 | 52.5 |
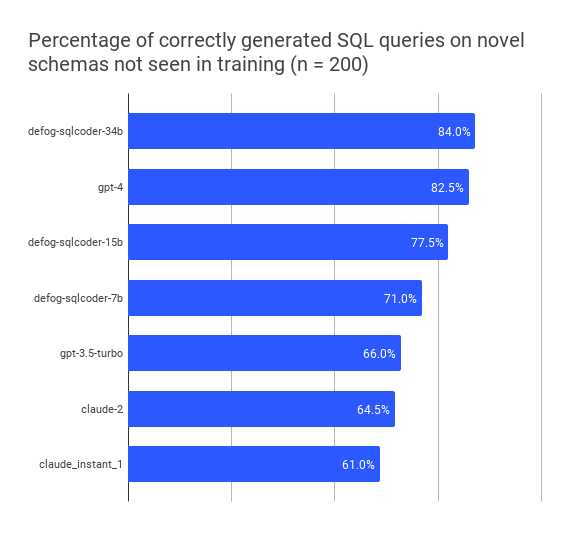
## License
The code in this repo (what little there is of it) is Apache-2 licensed. The model weights have a `CC BY-SA 4.0` license. The TL;DR is that you can use and modify the model for any purpose – including commercial use. However, if you modify the weights (for example, by fine-tuning), you must open-source your modified weights under the same license terms.
## Training
Defog was trained on more than 20,000 human-curated questions. These questions were based on 10 different schemas. None of the schemas in the training data were included in our evaluation framework.
You can read more about our [training approach](https://defog.ai/blog/open-sourcing-sqlcoder2-7b/) and [evaluation framework](https://defog.ai/blog/open-sourcing-sqleval/).
## Results by question category
We classified each generated question into one of 5 categories. The table displays the percentage of questions answered correctly by each model, broken down by category.
| | date | group_by | order_by | ratio | join | where |
| -------------- | ---- | -------- | -------- | ----- | ---- | ----- |
| sqlcoder-34b | 80 | 94.3 | 88.6 | 74.3 | 82.9 | 82.9 |
| gpt-4 | 68 | 94.3 | 85.7 | 77.1 | 85.7 | 80 |
| sqlcoder2-15b | 76 | 80 | 77.1 | 60 | 77.1 | 77.1 |
| sqlcoder-7b | 64 | 82.9 | 74.3 | 54.3 | 74.3 | 74.3 |
| gpt-3.5 | 68 | 77.1 | 68.6 | 37.1 | 71.4 | 74.3 |
| claude-2 | 52 | 71.4 | 74.3 | 57.1 | 65.7 | 62.9 |
| claude-instant | 48 | 71.4 | 74.3 | 45.7 | 62.9 | 60 |
| gpt-3 | 32 | 71.4 | 68.6 | 25.7 | 57.1 | 54.3 |
<img width="831" alt="image" src="https://github.com/defog-ai/sqlcoder/assets/5008293/79c5bdc8-373c-4abd-822e-e2c2569ed353">
## Using SQLCoder
You can use SQLCoder via the `transformers` library by downloading our model weights from the Hugging Face repo. We have added sample code for [inference](./inference.py) on a [sample database schema](./metadata.sql).
```bash
python inference.py -q "Question about the sample database goes here"
# Sample question:
# Do we get more revenue from customers in New York compared to customers in San Francisco? Give me the total revenue for each city, and the difference between the two.
```
You can also use a demo on our website [here](https://defog.ai/sqlcoder-demo)
## Hardware Requirements
SQLCoder-34B has been tested on a 4xA10 GPU with `float16` weights. You can also load an 8-bit and 4-bit quantized version of the model on consumer GPUs with 20GB or more of memory – like RTX 4090, RTX 3090, and Apple M2 Pro, M2 Max, or M2 Ultra Chips with 20GB or more of memory.
## Todo
- [x] Open-source the v1 model weights
- [x] Train the model on more data, with higher data variance
- [ ] Tune the model further with Reward Modelling and RLHF
- [ ] Pretrain a model from scratch that specializes in SQL analysis
# [Open LLM Leaderboard Evaluation Results](https://huggingface.co/spaces/HuggingFaceH4/open_llm_leaderboard)
Detailed results can be found [here](https://huggingface.co/datasets/open-llm-leaderboard/details_defog__sqlcoder-34b-alpha)
| Metric |Value|
|---------------------------------|----:|
|Avg. |55.59|
|AI2 Reasoning Challenge (25-Shot)|54.18|
|HellaSwag (10-Shot) |75.93|
|MMLU (5-Shot) |54.42|
|TruthfulQA (0-shot) |40.63|
|Winogrande (5-shot) |73.48|
|GSM8k (5-shot) |34.87|
|