|
--- |
|
license: other |
|
language: |
|
- en |
|
library_name: transformers |
|
inference: false |
|
thumbnail: https://h2o.ai/etc.clientlibs/h2o/clientlibs/clientlib-site/resources/images/favicon.ico |
|
tags: |
|
- gpt |
|
- llm |
|
- large language model |
|
- LLaMa |
|
datasets: |
|
- h2oai/h2ogpt-oig-oasst1-instruct-cleaned-v2 |
|
--- |
|
|
|
# h2ogpt-oasst1-512-30B-GGML |
|
|
|
These files are GGML format model files of [H2O.ai's h2ogpt-research-oig-oasst1-512-30b](https://huggingface.co/h2oai/h2ogpt-research-oig-oasst1-512-30b). |
|
|
|
GGML files are for CPU inference using [llama.cpp](https://github.com/ggerganov/llama.cpp). |
|
|
|
## Repositories available |
|
|
|
* [4bit GPTQ models for GPU inference](https://huggingface.co/TheBloke/h2ogpt-oasst1-512-30B-GPTQ). |
|
* [4bit and 5bit GGML models for CPU inference](https://huggingface.co/TheBloke/h2ogpt-oasst1-512-30B-GGML). |
|
* [float16 HF format unquantised model for GPU inference and further conversions](https://huggingface.co/TheBloke/h2ogpt-oasst1-512-30B-HF) |
|
|
|
## THE FILES IN MAIN BRANCH REQUIRES LATEST LLAMA.CPP (May 19th 2023 - commit 2d5db48)! |
|
|
|
llama.cpp recently made another breaking change to its quantisation methods - https://github.com/ggerganov/llama.cpp/pull/1508 |
|
|
|
I have quantised the GGML files in this repo with the latest version. Therefore you will require llama.cpp compiled on May 19th or later (commit `2d5db48` or later) to use them. |
|
|
|
For files compatible with the previous version of llama.cpp, please see branch `previous_llama_ggmlv2`. |
|
## Provided files |
|
| Name | Quant method | Bits | Size | RAM required | Use case | |
|
| ---- | ---- | ---- | ---- | ---- | ----- | |
|
`h2ogptq-oasst1-512-30B.ggmlv3.q4_0.bin` | q4_0 | 4bit | 20.3GB | 25GB | 4-bit. | |
|
`h2ogptq-oasst1-512-30B.ggmlv3.q4_1.bin` | q4_1 | 4bit | 24.4GB | 26GB | 4-bit. Higher accuracy than q4_0 but not as high as q5_0. However has quicker inference than q5 models. | |
|
`h2ogptq-oasst1-512-30B.ggmlv3.q5_0.bin` | q5_0 | 5bit | 22.4GB | 25GB | 5-bit. Higher accuracy, higher resource usage and slower inference. | |
|
`h2ogptq-oasst1-512-30B.ggmlv3.q5_1.bin` | q5_1 | 5bit | 24.4GB | 26GB | 5-bit. Even higher accuracy, and higher resource usage and slower inference.| |
|
`h2ogptq-oasst1-512-30B.ggmlv3.q8_0.bin` | q8_0 | 8bit | 36.6GB | 39GB | 8-bit. Almost indistinguishable from float16. Huge resource use and slow. Not recommended for normal use. | |
|
|
|
## How to run in `llama.cpp` |
|
|
|
I use the following command line; adjust for your tastes and needs: |
|
|
|
``` |
|
./main -t 8 -m h2ogptq-oasst1-512-30B.ggmlv3.q5_0.bin --color -c 2048 --temp 0.7 --repeat_penalty 1.1 -n -1 -p "Below is an instruction that describes a task. Write a response that appropriately completes the request. |
|
### Instruction: |
|
Write a story about llamas |
|
### Response:" |
|
``` |
|
Change `-t 12` to the number of physical CPU cores you have. For example if your system has 8 cores/16 threads, use `-t 8`. |
|
|
|
If you want to have a chat-style conversation, replace the `-p <PROMPT>` argument with `-i -ins` |
|
|
|
## How to run in `text-generation-webui` |
|
|
|
GGML models can be loaded into text-generation-webui by installing the llama.cpp module, then placing the ggml model file in a model folder as usual. |
|
|
|
Further instructions here: [text-generation-webui/docs/llama.cpp-models.md](https://github.com/oobabooga/text-generation-webui/blob/main/docs/llama.cpp-models.md). |
|
|
|
Note: at this time text-generation-webui may not support the new May 19th llama.cpp quantisation methods for q4_0, q4_1 and q8_0 files. |
|
|
|
# Original h2oGPT Model Card |
|
## Summary |
|
|
|
H2O.ai's `h2oai/h2ogpt-research-oig-oasst1-512-30b` is a 30 billion parameter instruction-following large language model for research use only. |
|
|
|
Due to the license attached to LLaMA models by Meta AI it is not possible to directly distribute LLaMA-based models. Instead we provide LORA weights. |
|
|
|
- Base model: [decapoda-research/llama-30b-hf](https://huggingface.co/decapoda-research/llama-30b-hf) |
|
- Fine-tuning dataset: [h2oai/h2ogpt-oig-oasst1-instruct-cleaned-v2](https://huggingface.co/datasets/h2oai/h2ogpt-oig-oasst1-instruct-cleaned-v2) |
|
- Data-prep and fine-tuning code: [H2O.ai GitHub](https://github.com/h2oai/h2ogpt) |
|
- Training logs: [zip](https://huggingface.co/h2oai/h2ogpt-research-oig-oasst1-512-30b/blob/main/llama-30b-hf.h2oaih2ogpt-oig-oasst1-instruct-cleaned-v2.2.0_epochs.131f6d098b43236b5f91e76fc074ad089d6df368.llama30b_17.zip) |
|
|
|
The model was trained using h2oGPT code as: |
|
|
|
```python |
|
torchrun --nproc_per_node=8 finetune.py --base_model=decapoda-research/llama-30b-hf --micro_batch_size=1 --batch_size=8 --cutoff_len=512 --num_epochs=2.0 --val_set_size=0 --eval_steps=100000 --save_steps=17000 --save_total_limit=20 --prompt_type=plain --save_code=True --train_8bit=False --run_id=llama30b_17 --llama_flash_attn=True --lora_r=64 --lora_target_modules=['q_proj', 'k_proj', 'v_proj', 'o_proj'] --learning_rate=2e-4 --lora_alpha=32 --drop_truncations=True --data_path=h2oai/h2ogpt-oig-oasst1-instruct-cleaned-v2 --data_mix_in_path=h2oai/openassistant_oasst1_h2ogpt --data_mix_in_factor=1.0 --data_mix_in_prompt_type=plain --data_mix_in_col_dict={'input': 'input'} |
|
``` |
|
On h2oGPT Hash: 131f6d098b43236b5f91e76fc074ad089d6df368 |
|
|
|
Only the last checkpoint at epoch 2.0 and step 137,846 is provided in this model repository because the LORA state is large enough and there are enough checkpoints to make total run 19GB. Feel free to request additional checkpoints and we can consider adding more. |
|
|
|
## Chatbot |
|
|
|
- Run your own chatbot: [H2O.ai GitHub](https://github.com/h2oai/h2ogpt) |
|
[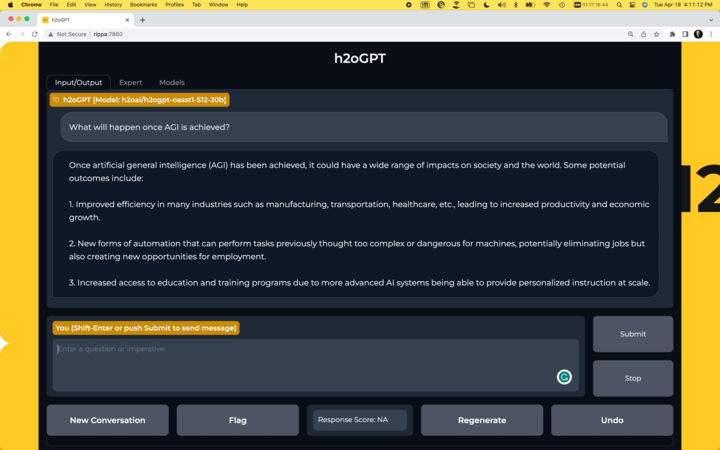](https://github.com/h2oai/h2ogpt) |
|
|
|
## Usage: |
|
|
|
### Usage as LORA: |
|
|
|
### Build HF model: |
|
|
|
Use: https://github.com/h2oai/h2ogpt/blob/main/export_hf_checkpoint.py and change: |
|
|
|
```python |
|
BASE_MODEL = 'decapoda-research/llama-30b-hf' |
|
LORA_WEIGHTS = '<lora_weights_path>' |
|
OUTPUT_NAME = "local_h2ogpt-research-oasst1-512-30b" |
|
``` |
|
where `<lora_weights_path>` is a directory of some name that contains the files in this HF model repository: |
|
|
|
* adapter_config.json |
|
* adapter_model.bin |
|
* special_tokens_map.json |
|
* tokenizer.model |
|
* tokenizer_config.json |
|
|
|
Once the HF model is built, to use the model with the `transformers` library on a machine with GPUs, first make sure you have the `transformers` and `accelerate` libraries installed. |
|
|
|
```bash |
|
pip install transformers==4.28.1 |
|
pip install accelerate==0.18.0 |
|
``` |
|
|
|
```python |
|
import torch |
|
from transformers import pipeline |
|
|
|
generate_text = pipeline(model="local_h2ogpt-research-oasst1-512-30b", torch_dtype=torch.bfloat16, trust_remote_code=True, device_map="auto") |
|
|
|
res = generate_text("Why is drinking water so healthy?", max_new_tokens=100) |
|
print(res[0]["generated_text"]) |
|
``` |
|
|
|
Alternatively, if you prefer to not use `trust_remote_code=True` you can download [instruct_pipeline.py](https://huggingface.co/h2oai/h2ogpt-oasst1-512-20b/blob/main/h2oai_pipeline.py), |
|
store it alongside your notebook, and construct the pipeline yourself from the loaded model and tokenizer: |
|
|
|
```python |
|
import torch |
|
from h2oai_pipeline import H2OTextGenerationPipeline |
|
from transformers import AutoModelForCausalLM, AutoTokenizer |
|
|
|
tokenizer = AutoTokenizer.from_pretrained("local_h2ogpt-research-oasst1-512-30b", padding_side="left") |
|
model = AutoModelForCausalLM.from_pretrained("local_h2ogpt-research-oasst1-512-30b", torch_dtype=torch.bfloat16, device_map="auto") |
|
generate_text = H2OTextGenerationPipeline(model=model, tokenizer=tokenizer) |
|
|
|
res = generate_text("Why is drinking water so healthy?", max_new_tokens=100) |
|
print(res[0]["generated_text"]) |
|
``` |
|
|
|
## Model Architecture with LORA and flash attention |
|
|
|
``` |
|
PeftModelForCausalLM( |
|
(base_model): LoraModel( |
|
(model): LlamaForCausalLM( |
|
(model): LlamaModel( |
|
(embed_tokens): Embedding(32000, 6656, padding_idx=31999) |
|
(layers): ModuleList( |
|
(0-59): 60 x LlamaDecoderLayer( |
|
(self_attn): LlamaAttention( |
|
(q_proj): Linear( |
|
in_features=6656, out_features=6656, bias=False |
|
(lora_dropout): ModuleDict( |
|
(default): Dropout(p=0.05, inplace=False) |
|
) |
|
(lora_A): ModuleDict( |
|
(default): Linear(in_features=6656, out_features=64, bias=False) |
|
) |
|
(lora_B): ModuleDict( |
|
(default): Linear(in_features=64, out_features=6656, bias=False) |
|
) |
|
) |
|
(k_proj): Linear( |
|
in_features=6656, out_features=6656, bias=False |
|
(lora_dropout): ModuleDict( |
|
(default): Dropout(p=0.05, inplace=False) |
|
) |
|
(lora_A): ModuleDict( |
|
(default): Linear(in_features=6656, out_features=64, bias=False) |
|
) |
|
(lora_B): ModuleDict( |
|
(default): Linear(in_features=64, out_features=6656, bias=False) |
|
) |
|
) |
|
(v_proj): Linear( |
|
in_features=6656, out_features=6656, bias=False |
|
(lora_dropout): ModuleDict( |
|
(default): Dropout(p=0.05, inplace=False) |
|
) |
|
(lora_A): ModuleDict( |
|
(default): Linear(in_features=6656, out_features=64, bias=False) |
|
) |
|
(lora_B): ModuleDict( |
|
(default): Linear(in_features=64, out_features=6656, bias=False) |
|
) |
|
) |
|
(o_proj): Linear( |
|
in_features=6656, out_features=6656, bias=False |
|
(lora_dropout): ModuleDict( |
|
(default): Dropout(p=0.05, inplace=False) |
|
) |
|
(lora_A): ModuleDict( |
|
(default): Linear(in_features=6656, out_features=64, bias=False) |
|
) |
|
(lora_B): ModuleDict( |
|
(default): Linear(in_features=64, out_features=6656, bias=False) |
|
) |
|
) |
|
(rotary_emb): LlamaRotaryEmbedding() |
|
) |
|
(mlp): LlamaMLP( |
|
(gate_proj): Linear(in_features=6656, out_features=17920, bias=False) |
|
(down_proj): Linear(in_features=17920, out_features=6656, bias=False) |
|
(up_proj): Linear(in_features=6656, out_features=17920, bias=False) |
|
(act_fn): SiLUActivation() |
|
) |
|
(input_layernorm): LlamaRMSNorm() |
|
(post_attention_layernorm): LlamaRMSNorm() |
|
) |
|
) |
|
(norm): LlamaRMSNorm() |
|
) |
|
(lm_head): Linear(in_features=6656, out_features=32000, bias=False) |
|
) |
|
) |
|
) |
|
trainable params: 204472320 || all params: 32733415936 || trainable%: 0.6246592790675496 |
|
``` |
|
|
|
## Model Configuration |
|
|
|
```json |
|
{ |
|
"base_model_name_or_path": "decapoda-research/llama-30b-hf", |
|
"bias": "none", |
|
"fan_in_fan_out": false, |
|
"inference_mode": true, |
|
"init_lora_weights": true, |
|
"lora_alpha": 32, |
|
"lora_dropout": 0.05, |
|
"modules_to_save": null, |
|
"peft_type": "LORA", |
|
"r": 64, |
|
"target_modules": [ |
|
"q_proj", |
|
"k_proj", |
|
"v_proj", |
|
"o_proj" |
|
], |
|
"task_type": "CAUSAL_LM" |
|
``` |
|
|
|
## Model Validation |
|
|
|
Classical benchmarks align with base LLaMa 30B model, but are not useful for conversational purposes. One could use GPT3.5 or GPT4 to evaluate responses, while here we use a [RLHF based reward model](OpenAssistant/reward-model-deberta-v3-large-v2). This is run using h2oGPT: |
|
|
|
```python |
|
python generate.py --base_model=decapoda-research/llama-30b-hf --gradio=False --infer_devices=False --eval_sharegpt_prompts_only=100 --eval_sharegpt_as_output=False --lora_weights=llama-30b-hf.h2oaih2ogpt-oig-oasst1-instruct-cleaned-v2.2.0_epochs.131f6d098b43236b5f91e76fc074ad089d6df368.llama30b_17 |
|
``` |
|
|
|
So the model gets a reward model score mean of 0.55 and median of 0.58. This compares to our [20B model](https://huggingface.co/h2oai/h2ogpt-oasst1-512-20b) that gets 0.49 mean and 0.48 median or [Dollyv2](https://huggingface.co/databricks/dolly-v2-12b) that gets 0.37 mean and 0.27 median. |
|
|
|
[Logs](https://huggingface.co/h2oai/h2ogpt-research-oig-oasst1-512-30b/blob/main/score_llama30b_jon17d.log) and [prompt-response pairs](https://huggingface.co/h2oai/h2ogpt-research-oig-oasst1-512-30b/blob/main/df_scores_100_100_1234_False_llama-30b-hf_llama-30b-hf.h2oaih2ogpt-oig-oasst1-instruct-cleaned-v2.2.0_epochs.131f6d098b43236b5f91e76fc074ad089d6df368.llama30b_17.parquet) |
|
|
|
The full distribution of scores is shown here: |
|
|
|
 |
|
|
|
Same plot for our h2oGPT 20B: |
|
|
|
 |
|
|
|
Same plot for DB Dollyv2: |
|
|
|
 |
|
|
|
|
|
|
|
## Disclaimer |
|
|
|
Please read this disclaimer carefully before using the large language model provided in this repository. Your use of the model signifies your agreement to the following terms and conditions. |
|
|
|
- The LORA contained in this repository is only for research (non-commercial) purposes. |
|
- Biases and Offensiveness: The large language model is trained on a diverse range of internet text data, which may contain biased, racist, offensive, or otherwise inappropriate content. By using this model, you acknowledge and accept that the generated content may sometimes exhibit biases or produce content that is offensive or inappropriate. The developers of this repository do not endorse, support, or promote any such content or viewpoints. |
|
- Limitations: The large language model is an AI-based tool and not a human. It may produce incorrect, nonsensical, or irrelevant responses. It is the user's responsibility to critically evaluate the generated content and use it at their discretion. |
|
- Use at Your Own Risk: Users of this large language model must assume full responsibility for any consequences that may arise from their use of the tool. The developers and contributors of this repository shall not be held liable for any damages, losses, or harm resulting from the use or misuse of the provided model. |
|
- Ethical Considerations: Users are encouraged to use the large language model responsibly and ethically. By using this model, you agree not to use it for purposes that promote hate speech, discrimination, harassment, or any form of illegal or harmful activities. |
|
- Reporting Issues: If you encounter any biased, offensive, or otherwise inappropriate content generated by the large language model, please report it to the repository maintainers through the provided channels. Your feedback will help improve the model and mitigate potential issues. |
|
- Changes to this Disclaimer: The developers of this repository reserve the right to modify or update this disclaimer at any time without prior notice. It is the user's responsibility to periodically review the disclaimer to stay informed about any changes. |
|
|
|
By using the large language model provided in this repository, you agree to accept and comply with the terms and conditions outlined in this disclaimer. If you do not agree with any part of this disclaimer, you should refrain from using the model and any content generated by it. |
|
|