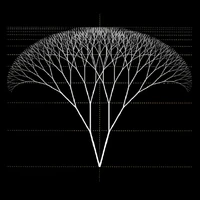
Marcus2112/pythia-160m-minipile_pico_density-proportioned
Updated
•
11
text
stringlengths 10
3.78M
| pile_idx
int64 2.62k
134M
|
---|---|
Q:
TCSH script full path
In bash shell I can get a full path of a script even if the script is called by source, link, ./..., etc.
These magic bash lines:
#Next lines just find the path of the file.
#Works for all scenarios including:
#when called via multiple soft links.
#when script called by command "source" aka . (dot) operator.
#when arg $0 is modified from caller.
#"./script" "/full/path/to/script" "/some/path/../../another/path/script" "./some/folder/script"
#SCRIPT_PATH is given in full path, no matter how it is called.
#Just make sure you locate this at start of the script.
SCRIPT_PATH="${BASH_SOURCE[0]}";
if [ -h "${SCRIPT_PATH}" ]; then
while [ -h "${SCRIPT_PATH}" ]; do SCRIPT_PATH=`readlink "${SCRIPT_PATH}"`; done
fi
pushd `dirname ${SCRIPT_PATH}` > /dev/null
SCRIPT_PATH=`pwd`;
popd > /dev/null
How can you get the script path under the same conditions in TCSH shell? What are these 'magic lines'?
P.S. It is not a duplicate of this and similar questions. I'm aware of $0.
A:
I don't use tcsh and do not claim guru status in it, or any other variant of C shell. I also firmly believe that Csh Programming Considered Harmful contains much truth; I use Korn shell or Bash.
However, I can look at manual pages, and I used the man page for tcsh (tcsh 6.17.00 (Astron) 2009-07-10 (x86_64-apple-darwin) on MacOS 10.7.1 Lion).
As far as I can see, there is no analogue to the variable ${BASH_SOURCE[0]} in tcsh, so the starting point for the script fragment in the question is missing. Thus, unless I missed something in the manual, or the manual is incomplete, there is no easy way to achieve the same result in tcsh.
The original script fragment has some problems, too, as noted in comments. If the script is invoked with current directory /home/user1 using the name /usr/local/bin/xyz, but that is a symlink containing ../libexec/someprog/executable, then the code snippet is going to produce the wrong answer (it will likely say /home/user1 because the directory /home/libexec/someprog does not exist).
Also, wrapping the while loop in an if is pointless; the code should simply contain the while loop.
SCRIPT_PATH="${BASH_SOURCE[0]}";
while [ -h "${SCRIPT_PATH}" ]; do SCRIPT_PATH=`readlink "${SCRIPT_PATH}"`; done
You should look up the realpath() function; there may even be a command that uses it already available. It certainly is not hard to write a command that does use realpath(). However, as far as I can tell, none of the standard Linux commands wrap the realpath() function, which is a pity as it would help you solve the problem. (The stat and readlink commands do not help, specifically.)
At its simplest, you could write a program that uses realpath() like this:
#include <stdio.h>
#include <stdlib.h>
#include <string.h>
#include <errno.h>
int main(int argc, char **argv)
{
int rc = EXIT_SUCCESS;
for (int i = 1; i < argc; i++)
{
char *rn = realpath(argv[i], 0);
if (rn != 0)
{
printf("%s\n", rn);
free(rn);
}
else
{
fprintf(stderr, "%s: failed to resolve the path for %s\n%d: %s\n",
argv[0], argv[i], errno, strerror(errno));
rc = EXIT_FAILURE;
}
}
return(rc);
}
If that program is called realpath, then the Bash script fragment reduces to:
SCRIPT_PATH=$(realpath ${BASH_SOURCE[0]})
| 50,331,746 |
Q:
Setting object style in InDesign
I'm trying to somehow set InDesign object style for images that I add into text frame directly so they flow along with text when I edit content. My document is of technical nature so it has a single text frame per page. It's more of technical document really.
This are my requirements:
images need to break text block apart, so text won't flow over/around them
images need to centrally aligned in the text frame regardless of their width
images have to move with text (hence they are pasted into)
images have a description that must never break to next page
images have to align to the last baseline gridline just above description so description always appears it's positioned exactly the same space after image (upper margin will therefore vary but should be at least one leading high) - By last baseline I mean if an images occupies few line-heights (leadings), bottom of the image frame should align exactly with the last line and not somewhere in between.
I'm heaving great difficulty creating my object style to accomplish this. The main problem being positioning images exactly on the last baseline grid.
A:
You can do this.
I've tested the following in CS5 and 5.5, but the technique should work at least as far back as CS3, when iirc Object Styles were first introduced.
So, in sequence:
To get started, set up a Paragraph Style of "Image", which is set to align to grid and centered. First line, all lines, doesn't matter. The paragraph will only contain one line.
Set up a paragraph style called "Description." In Keep Options, check "Keep With Previous."
Edit the "Image" paragraph style, to add "Next Style: Description." (This isn't essential, but it makes things quicker later.)
Paste your first image into its own paragraph, and set the style to "Image."
Set the anchored image options to "Inline or Above Line" and choose the "Inline" radio button. The Y offset should be 0. The bottom of the image will now be sitting on a baseline. The top of the image will be below the last line of the previous paragraph.
Select the image and Alt/Option-Click the new style icon in the Object Styles panel. Call this "Image" and give it a keyboard shortcut. Activate the Paragraph Styles checkbox, and "Use Next Style" in the associated Paragraph Styles dialog. Select the "Anchored Object Options" checkbox, and verify those settings.
You're all set. You can now paste an inline image into its own paragraph, assign the "Image" object style using the keyboard shortcut, and press Enter/Return to create the next paragraph, which will have a paragraph style of "Description" assigned automatically. (If you are working on existing text, select the image and the description and right-click the "Image" paragraph style in the Paragraph Styles panel, then choose "Apply Image then Next Style" from the context menu.)
At this point you have an image that won't have any text flowing around it, with a description that will never break to the next page without taking the image with it.
A:
I don't think you'll be able to accomplish this through styles alone with those last two bullets being a requirement.
For one, object styles don't have a setting for anything having to do with the grid except when working with a text frame, and even then relative-to-grid settings only deal with the top of the frame which won't be of much help. Second, you could place the image frame in the text flow and apply a paragraph, but then a paragraph style aligns to a grid by the first line or all lines, but nothing in between.
If dodging repetitive work is the goal here, then the only thing I can think of would be to create an object library that has the objects you need and some hooks for automation (like an applied label on the backend) and write up a script that will then go through the document, find the objects, and fix the layout "automagically" after you have finished populating your document. That's no small feat, and even with the most robust automatic layout systems, someone still has to go in a tweak layouts manually if layout quality is even a vague concern.
I suggest either relaxing your layout requirements or handle this manually as you do your layout. It's a drag no matter how I look at this but that's also why a lot of us have jobs.
| 50,331,774 |
A wonderful way Vine Street both shares its facilities while providing important ministry is through the Pastoral Counseling Center of Tennessee (PCCT). Vine Street founded PCCT in 1985 to provide affordable, professional counseling in middle Tennessee. PCCT has several locations in the Nashville area, but its main office is on Vine Street’s campus in the Fitzpatrick House. It has been our neighbor for the last 29 years.
The work at PCCT is unique in that the counselors are not only licensed clinicians in a mental health field, but they also have in-depth religious and theological training. The mission of PCCT is “to restore lives to wholeness—mentally, emotionally, and spiritually.” The staff provides individual, marital and family therapy, and services for Spanish-speaking clients are offered, too. Last year the Vine Street location served 400 clients, totaling 1,596 sessions of counseling and $125,000 of financial assistance. In addition, you may recall Vine Street donated $7,500 last year to PCCT, which was made possible by a generous gift left by Mrs. Hallie Warner. This gift provided 111 sessions to senior adult clients in need of financial assistance. PCCT is committed to helping all who are in need of counseling regardless of their financial situation, and it offers a sliding scale fee option for those who need financial assistance.
Pastoral Counseling Center of Tennessee is a major ministry Vine Street Christian Church supports right here on our campus.
Sundays: Communion in the chapel at 8:30 a.m. | Traditional Worship at 10:00 a.m. in the Sanctuary| Christian Education at 9 a.m.
About
CONNECT
I'M NEW
Vine Street Christian Church is a member of the Christian Church (Disciples of Christ). We are a movement for wholeness in a fragmented world. As part of the one body of Christ, we welcome all to the Lord's Table as God has welcomed us. | 50,331,898 |
Role of inhibition of uroporphyrinogen decarboxylase in PCB-induced porphyria in mice.
The oral administration of 3,4,5,3',4',5'-hexachlorobiphenyl for 3 weeks to mice caused a marked accumulation of porphyrins in the liver of C57BL/6 and C57Bl/10 mice but not in the liver of ddY mice. The time course of induction of delta-aminolevulinic acid synthetase (ALA-S), cytochrome P-450, and mixed function oxidases and inhibition of uroporphyrinogen decarboxylase (URO-D) in the liver of C57BL/6 mice and ddY mice fed a diet containing 500 ppm of a commercial PCB (Kanechlor-500) were investigated to clarify the sole factor in inducing porphyria. The activity of URO-D in the liver of C57BL/6 mice was depressed approximately 80% at 3 weeks when a large amount of uroporphyrin accumulated. Male ddY mice showed only a slight increase in uroporphyrin accumulation in the liver and a moderate decrease of URO-D activity even at the 10th week. ALA-S, cytochrome P-450, and mixed function oxidases were induced in both strains of mice, although the magnitude of these inductions in C57BL/6 mice was greater than that in ddY mice. No differences were detected between the two strains in the content and gas chromatographic pattern of PCB remaining in liver cytosol (6 weeks). In addition there was no relationship between the time of onset of porphyria and that of the maximal induction of drug-metabolizing function in C57BL/6 mice. These results indicate that the development of porphyria is causally related to the inhibition of URO-D rather than the induction of drug-metabolizing function. The hypothesis that porphyria first develops when the ratio of hepatic URO-D and ALA-S activities decreases to less than 1.0 is presented. | 50,333,255 |
[Establishment and evaluation of the SD rat allergic rhinitis model].
To investigate method established and system evaluated in the model of SD rat with AR. To establish AR model of SD rats by ovalbumin (OVA), 20 cases of SD rats were randomly divided into two groups, namely control group (10 cases) and AR group (10 cases). AR models were sensitized and challenged by OVA. Control group were used with normal saline instead of OVA. The score of pathology and praxiology were observed when the SD rats in AR group appeared typical symptom of allergic rhinitis, and levels of IL-4, IFN-γ, IgE in the serum were examined by ELISA. According to the behavioral score, nasal histology and content of IL-4, IFN-γ, IgE of serum, Rat allergic rhinitis model were judged successfully established or not. Behavioral scores were significantly increased in OVA-challenged rats compared with the control group, P<0.05. Nasal epithelial goblet cells, eosinophils and lymphocytes in nasal mucosa in the AR rats exhibited obvious increase relative to the control group. IL-4, IgE levels in the AR rat exhibited obvious increase relative to control group while INF-γ levels exhibited obvious reduction (P<0.05). The allergic rhinitis models in SD rat by OVA were successfully established. The levels of IgE, INF-γ and IL-4 in Serum can be used as objective evaluation of animal models of allergic rhinitis established successfully or not. | 50,333,327 |
The RNA-Seq data reported in this paper are available from the NCBI Sequence Read Archive under accession number GSE87194.
Introduction {#sec001}
============
Schizophrenia is a serious psychiatric disorder adversely affecting the quality of life of a significant number of people \[[@pone.0166944.ref001]\]. Schizophrenia arises from a complex and varied set of environmental and genetic factors, which has made it very difficult to come to a clear understanding of the etiology of the condition, despite intensive scientific work in the area. However, it seems that a disease arising from the interplay of genes and environment is likely to involve the super family of nuclear receptors which are known to control gene expression depending on context.
A group of 48 transcription factors play a key role in transducing extracellular (environmental, metabolic, endocrine) signals into intercellular signals, resulting in changes in expression of target genes. The nuclear receptors (NRs) are grouped into 6 functionally related sub-families (NR1---NR6) and include the estrogen and androgen receptors (NR3A1/ESR1 and NR3C4/AR), the glucocorticoid and mineralocorticoid receptors (NR3C1/GR and NR3C2/MR), the retinoid receptors (NR1B/RARs and NR2B/RXRs), the vitamin D receptor (NR1I1/VDR), the peroxisome proliferator-activated (fatty acid) receptors (NR1C/PPARs) and the orphan nuclear receptors (NR4A sub-family)\[[@pone.0166944.ref002]\]. A number of these genes/transcripts have been implicated in schizophrenia, including the estrogen \[[@pone.0166944.ref003], [@pone.0166944.ref004]\] and the glucocorticoid receptors \[[@pone.0166944.ref005]--[@pone.0166944.ref008]\], the retinoid (vitamin A) receptors \[[@pone.0166944.ref009]\] and the NR4A (orphan) receptors \[[@pone.0166944.ref010]\]. The nuclear receptors generally dimerize to form either homodimers or heterodimers with other nuclear receptors and may be activated by multiple ligands. They are therefore part of a complex network of molecules essential for development and adaptive responses in the adult.
To gain a more complete picture of alterations in nuclear receptor alterations, we have focused this study on the NR4A sub-family of nuclear receptors (NR4A1 (Nurr 77 or NGF1B), NR4A2 (Nurr1), NR4A3 (Nor1)), and their dimerization partners, the retinoid X receptors (RXRA, RXRB, RXRG) and the retinoic acid receptors (RARA, RARB, RARG).
The RAR proteins are activated by all-trans retinoic acid while the RXR proteins are activated by by 9-cis retinoic acid, and other ligands such as the omega 3 unsaturated fatty acids and various synthetic compounds \[[@pone.0166944.ref011], [@pone.0166944.ref012]\]. NR4A1 and NR4A2, but not NR4A3 \[[@pone.0166944.ref013], [@pone.0166944.ref014]\], form active heterodimers with RXRA and RXRG \[[@pone.0166944.ref015], [@pone.0166944.ref016]\] and in this form can bind to the retinoid acid response elements in genomic DNA \[[@pone.0166944.ref015]\]. Whilst NR4A3 does not heterodimerize with the RXRs, it can interfere with the signaling from either the NR4A1-RXR or NR4A2-RXR complexes \[[@pone.0166944.ref014]\]. RXR dimerizes with several nuclear receptors including the retinoid receptors (RARs sub-family), the vitamin D receptor (VDR), the thyroid hormone receptors (T3Rs) and the lipid activated nuclear receptors (PPARs).
While NR4A2 has an important role in the cell body of dopaminergic neurons, the action of NR4A1 is more pronounced at target areas of the dopaminergic neurons, such as the prefrontal cortex. The NR4A1- RXR complex is suggested to function as an adaptive homeostatic regulator of dopamine neurotransmission \[[@pone.0166944.ref017]\]. Blockers of dopamine transmission, antipsychotics, can impact the expression of NR4A genes \[[@pone.0166944.ref018], [@pone.0166944.ref019]\] and gene ablation studies have demonstrated changes in response to antipsychotic medication in NR4A1 null mice \[[@pone.0166944.ref020], [@pone.0166944.ref021]\]. Thus, it is important to consider if the levels of antipsychotic drug levels correlate with levels of these nuclear receptor mRNAs in the brains of people with schizophrenia.
In this study, we have quantified and compared the mRNA expression of genes encoding nuclear receptors with a focus on those in the NR4A and RXR/RAR families. This study aims to determine if altered levels of the mRNA expressions in orphan nuclear receptors and retinoid receptors exist in the brains of people with schizophrenia using next generation sequencing and by real time quantitative polymerase chain reaction (RT-qPCR) in the DLPFC.
Methods {#sec002}
=======
Post-mortem brain samples {#sec003}
-------------------------
Dorsal lateral prefrontal cortex (DLPFC) from thirty-seven schizophrenia/schizoaffective cases and thirty-seven controls was obtained from the New South Wales Tissue Resource Centre. Of the thirty-seven schizophrenia/schizoaffective cases, eight were on first generation antipsychotics only, twenty-two had predominantly received first generation antipsychotics, one was one second generation antipsychotics only, five had predominantly received second generation antipsychotics, and one received equal first and second generation antipsychotics. Cases were matched for sample pH, age, post-mortem interval (PMI), and RNA integrity number (RIN) ([Table 1](#pone.0166944.t001){ref-type="table"}). Details of tissue characterization have been previously described \[[@pone.0166944.ref022]\]. All research was approved by and conducted under the guidelines of the Human Research Ethics Committee at the University of New South Wales (HREC 12435- Investigation of schizophrenia pathogenesis using post-mortem brain tissue). 300 mg of DLPFC was weighed out for total RNA extraction using TRIzol® Reagent (Life Technologies Inc., Grand Island, N.Y., U.S.A., catalogue number: 15596--018), as previously described \[[@pone.0166944.ref023]\], The quantity and quality of RNA was determined using a spectrophotometer (Nanodrop ND-1000, Thermo Fisher Scientific) and Agilent Bioanalyzer 2100 (Agilent Technologies, Palo Alto, CA, USA).
10.1371/journal.pone.0166944.t001
###### Control and Schizophrenia Cohort Demographics.
{#pone.0166944.t001g}
Control Group Schizophrenia Group
---------------------------------------- -------------------------- ---------------------------
Number of Cases Healthy Controls = 37 SZ = 30, SA = 7
Age (years) 51.1 (18--78) 51.3 (27--75)
Gender F = 7, M = 30 F = 13, M = 24
Hemisphere L = 14, R = 23 L = 20, R = 17
pH 6.66 ± 0.29 (5.84--7.19) 6.61 ± 0.30 (5.69--7.09)
Post-Mortem Interval (hours) 24.8 ± 10.97 (6.5--50) 28.8 ± 14.07 (5--72)
RNA Integrity Number (RIN) 7.3 ± 0.57 (6.0--8.4) 7.3 ± 0.58 (6.2--8.4)
Manner of Death Natural = 37 Natural = 29, Suicide = 8
Age of onset (years) \- 23.7 ± 0.1
Duration of Illness (years) \- 27.6 ± 2.3
Daily Chlorpromazine Mean (mg) \- 692 ± 502
Last Recorded Chlorpromazine Dose (mg) \- 542 ± 374
Key: SZ = schizophrenia, SA = schizoaffective; F = Female, M = Male; L = left, R = Right; ± = Standard Deviation
cDNA derived from total RNA from the DLPFC tissue of a cohort of 20 schizophrenia/schizoaffective cases (referred to as schizophrenia) and 20 control samples was sequenced using the ABI SOLiD platform as previously described \[[@pone.0166944.ref024]\]. In this study, we took the raw data generated from 19 of the schizophrenia samples and 19 control samples. We excluded one schizophrenia sample as the raw data file had been damaged and it was not possible to use it in further mapping. We also excluded one control sample who was phenotypically male but putatively XXY. We mapped the 50 nucleotide reads to the human genome (hg19) using TopHat2 (v 2.0.4) \[[@pone.0166944.ref025]\], which calls the Bowtie aligner (v 0.12.8) \[[@pone.0166944.ref026]\], allowing up to 2 bp mismatches per read (default position). HTSeq-count (Python package HTSeq, python v 2.7.3) was used to generate counts of reads uniquely mapped to known and annotated genes (freeze date October 2011) using the Ensembl annotation file GRCh37.66_chr.gtf (mode = union,--t = exon,--i = gene_name). The count table of uniquely mapped reads was then used for differential expression analysis. Differential expression was tested using the Bioconductor package, edgeR (v 3.12.1) \[[@pone.0166944.ref027]\] and confirmed using DESeq2 (v 1.10.1) \[[@pone.0166944.ref028]\]. We used a generalized linear model (GLM) with batch as well as the diagnostic (schizophrenia versus control) as factors in the design matrix \[[@pone.0166944.ref029]\] in each of the analyses.
In carrying out this analysis, we have used read data (fastq files) obtained from RNA-Seq previously performed on the DLPFC of post-mortem brain. Tools for the analysis of RNA-Seq data have improved rapidly over recent years. Since the time when this data was first analyzed \[[@pone.0166944.ref024]\] commonly used analysis tools such as edgeR and DESeq have undergone important developments. They now allow covariates such as batch to be routinely incorporated in experimental analysis and provide a more sophisticated treatment of the variation in gene expression (dispersion estimation) \[[@pone.0166944.ref029], [@pone.0166944.ref030]\]. We have used these new methods in the work reported here.
In the edgeR analysis, low count transcripts were excluded and only those genes with at least 1 count per million (cpm), in at least 10 samples, were used for analysis. This filtering retained 17,483 of the original 42,358 transcripts. Normalization was performed using the trimmed mean of M values (TMM) \[[@pone.0166944.ref027]\]. The dispersion parameter for each gene was estimated with the Cox-Reid common dispersion method \[[@pone.0166944.ref029]\]. Testing for differential expression employed a negative binomial generalized linear model for each gene.
In the DESeq2 confirmatory analysis, normalization was performed using the median-of-ratios method \[[@pone.0166944.ref028]\]. Dispersions were estimated using a Cox-Reid adjusted profile likelihood and the Wald test for significance of GLM was used. DESeq2 invokes automatic filtering to optimize the number of genes that have an adjusted p value below the threshold (default 0.1). This resulted in the retention of 17,447 transcripts. In both workflows the Benjamini-Hochberg correction was used to correct for multiple comparisons with a false discovery rate of 0.10.
The differential expression between schizophrenia and control are distinct from those obtained in our earlier analysis \[[@pone.0166944.ref024]\] due to the alternative analysis streams employed. For this edgeR analysis, estimates of dispersion take into account the actual variation seen in counts for a gene across samples (tagwise dispersion) as well as the common dispersion, which is a value derived from the entire gene set. The tagwise dispersion for a gene is modulated towards the common dispersion by applying a weighting factor (prior.n). Earlier versions of edgeR set the prior.n value at 10, which moved the tagwise dispersions strongly towards the common dispersion value. This was based upon the assumption that RNA-Seq projects generally consisted of few samples and accordingly the small sample size could not alone provide a reliable estimate of dispersion. Later versions of edgeR including v 3.12.1 (used in this analysis) altered these general settings to allow greater sensitivity to the number of samples used in an experiment. The greater the number of samples, the more reliable the tagwise dispersion should be, thus reducing the modulation towards a common dispersion value. The weighting towards the common dispersion is now calculated taking account of the number of samples and groups being analysed. Under the current default settings in edgeR (v 3.12.1) the prior.df is set to 10 with a resulting prior.n of approximately 0.3 (prior.n = prior.df /residual.df). That is, for a data set of this size, with 19 individual samples, there is very little smoothing towards a common dispersion value. Instead, more weight is given to the actual variation in count numbers for a particular gene gleaned from the data for that gene. In a data set where there is a large divergence of count values for a particular gene or where there may be one or two samples with an extreme value, tagwise dispersion will make it less likely that such a gene will be called as differentially expressed. The fact that we see a reduction in differentially expressed genes using the current method is indicative of a spread of gene counts rather than a tighter clustering of values among individuals for a gene expression level.
Clustering functions available in the gplots package in the R environment (v 3.0.2) (<http://www.R-project.org>) \[[@pone.0166944.ref031]\] were used to generate heatmaps. The Metacore database was used for ascertaining interaction partners of particular genes. The Cytoscape software platform ([www.cytoscape.org](http://www.cytoscape.org/)) \[[@pone.0166944.ref032]\] was used for constructing protein interaction networks.
qPCR analysis {#sec004}
-------------
SuperScript® II/III First-Strand Synthesis System (Life Technologies, catalogue number: 11904-018/18080-400) was used for cDNA synthesis from 3 μg RNA. The protocol for SuperScript® II was followed by adding random hexamers, nucleoside triphosphate (dNTP), and RNase OUT to each sample. After incubating at 65°C, tris-hydrochloride (tris-HCl), potassium chloride (KCl), magnesium chloride (Mg2Cl), dithiothreitol (DTT) and SuperScript® II were added. All samples were incubated at room temperature for 10 min before heating it to 42°C for 50 min, followed by 70°C for 15 min. RNase H was added to all samples before incubating at 37°C. We followed the protocol for SuperScript® III First-Strand Synthesis System according to manufacturer's instructions.
cDNA was plated out with a seven-point serial diluted standard curve, followed by quantitative real time PCR, probing with various primers to amplify members of the nuclear receptor superfamily ([Table 2](#pone.0166944.t002){ref-type="table"}). Samples were measured in triplicates on the 7900HT Fast Real-Time PCR System. The quantity means obtained from the relative standard curve method from serial dilutions of cDNA (1:3, 1:9, 1:27 etc) of our genes of interest were normalized to the geometric mean of four housekeeping genes: β-actin, ubiquitin C, glyceraldehyde-3-phosphate dehydrogenase, and TATA box binding protein ([Table 2](#pone.0166944.t002){ref-type="table"}). There was no difference in the mRNA levels for housekeepers between the schizophrenia and control groups \[[@pone.0166944.ref022]\].
10.1371/journal.pone.0166944.t002
###### List of Taqman genes of interest.
{#pone.0166944.t002g}
Gene Gene Name Assay ID
-------------------------------------------- ------------------------------------------------- -------------------------------------------------
NR4A1/Nur77 Nuclear receptor subfamily 4, group A, member 1 Hs00374226_m1
NR4A2/Nurr1 Nuclear receptor subfamily 4, group A, member 2 Hs00428691_m1
NR4A3/Nor1 Nuclear receptor subfamily 4, group A, member 3 Hs00545009_g1
KLF4 Kruppel-like factor 4 Hs00358836_m1
VDR Vitamin D receptor Hs01045840_m1
RARA Retinoic Acid Receptor, alpha Hs00940446_m1
RARB Retinoic Acid Receptor, beta GCAGAGCGTGTAATTACCTTGAA/GTGAGATGCTAGGACTGTGCTCT
RARG Retinoic Acid Receptor, gamma Hs01559234_m1
RXRA Retinoid X Receptor, alpha Hs01067640_m1
RXRB Retinoid X Receptor, beta Hs00232774_m1
RXRG Retinoid X Receptor, gamma Hs00199455_m1
ACTβ[\*](#t002fn001){ref-type="table-fn"} Actin, beta Hs99999903_m1
UBC[\*](#t002fn001){ref-type="table-fn"} Ubiquitin C Hs00824723_m1
GAPDH[\*](#t002fn001){ref-type="table-fn"} Glyceraldehyde-3-phosphate dehydrogenase Hs99999905_m1
TBP[\*](#t002fn001){ref-type="table-fn"} TATA box binding protein Hs00427620_m1
\*Housekeeper genes
Statistical analysis of qPCR results {#sec005}
------------------------------------
Normalized data was analyzed using IBM SPSS Statistics 23.0. KLF4 and RXRB were log10 transformed for normal distribution within each diagnostic group. All data were tested for correlation with age, pH, PMI and RIN. The correlations between the gene expressions with each of these factors are listed in [S1 Table](#pone.0166944.s006){ref-type="supplementary-material"}. ANOVA and ANCOVA were performed when appropriate. The results were analyzed for diagnostic and gender differences. We performed Spearman's correlation in the schizophrenia group with chlorpromazine dosages, illness duration, and on target mRNAs.
Results {#sec006}
=======
Nuclear receptor family genes are expressed in three clusters {#sec007}
-------------------------------------------------------------
We sought to characterize nuclear receptor mRNA change in the context of the expression landscape of all nuclear receptor genes in the DLPFC. A cluster-based analysis revealed that the nuclear receptor genes expressed in the adult human prefrontal cortex fall into three main groups, highly expressed genes, moderately expressed genes and lowly expressed genes ([Fig 1](#pone.0166944.g001){ref-type="fig"}). Our analysis indicates that NR4A1 falls within the moderately expressed cluster and is expressed at similar abundance levels as the other members of this sub-family NR4A2 (Nurr1) and NR4A3 (Nor-1). Further, the expression levels of the NR4A genes is in a similar range to that of members of the retinoid receptors (RARs and RXRs, part of the NR1B and NR2B sub-families), and the sex steroid hormone receptors such as the estrogen and androgen receptors (ESR1 and AR). As we were interested in seeing the relative expression levels of the nuclear receptors in the DLPFC we adjusted for gene length and also clustered by gene name rather than by sample.
{#pone.0166944.g001}
Nuclear receptor NR4A1 is significantly downregulated in schizophrenia {#sec008}
----------------------------------------------------------------------
Using RNA-Seq, we analyzed gene expression in the DLPFC of 19 schizophrenia patients and compared that to 19 controls. The biological coefficient of variation (BCV) calculated using the methods available in edgeR produced a value of 0.3863 for the 19 control samples and 0.479 for the 19 schizophrenia samples. This does indicate a slightly greater degree of variability in samples from people with schizophrenia compared to samples from the controls; however it also reflects that there is also quite a bit of variability in samples from controls as well. Considerable gene expression variability was seen between individuals, which was unsurprising for human brain and patient-derived samples. This could be due to the uncontrolled factors in case-control studies (such as age at death, time of death, or gender) and also due to the heterogeneous nature of schizophrenia. Consequently, we did not see a strong distinction between the two diagnostic categories when examining global gene expression via a multidimensional scaling (MDS) plot ([S1 Fig](#pone.0166944.s001){ref-type="supplementary-material"}). However, a small group of genes, which had not been previously reported by us to be associated with schizophrenia, was revealed with these novel analysis parameters. The top 20 differentially expressed genes (FDR\<0.1) found using edgeR are given in [Table 3](#pone.0166944.t003){ref-type="table"}. [S2 Fig](#pone.0166944.s002){ref-type="supplementary-material"} in the Supplementary material shows the sensitivity of the number of differentially expressed genes (found at an FDR\<0.1) to changes in prior.n from 0.3 to 2, 5 and 10. Full details of the DEGs found using these different values of prior.n are included as [S2 Table](#pone.0166944.s007){ref-type="supplementary-material"}.
10.1371/journal.pone.0166944.t003
###### Significant differentially expressed genes in schizophrenia compared to controls (FDR \< 0.1) from use of edgeR.
{#pone.0166944.t003g}
Gene Protein name log2FC[^a^](#t003fn001){ref-type="table-fn"} p-value[^a^](#t003fn001){ref-type="table-fn"} FDR[^a^](#t003fn001){ref-type="table-fn"}
--------------- ---------------------------------------------------------- ---------------------------------------------- ----------------------------------------------- -------------------------------------------
NR4A1 Nuclear receptor subfamily 4, group A member 1 -1.13 1 x 10^−6^ 0.019
KLF4 Kruppel-like factor 4 -0.99 6.49 x 10^−6^ 0.062
EIF2AP4 Eukaryotic translation initiation factor 2A pseudogene 4 1.08 2.26 x 10^−5^ \<0.1
RTN4R Reticulon 4 receptor -0.69 2.49 x 10^−5^ \<0.1
COL5A3 Collagen, type V, alpha 3 -0.55 3.44 x 10^−5^ \<0.1
ARRDC3 Arrestin domain containing 3 0.64 3.52 x 10^−5^ \<0.1
ADAMTS9-AS2 ADAMTS9 antisense RNA 2 0.53 3.74 x 10^−5^ \<0.1
GAREML/FAM59B GRB2 associated, regulator of MAPK1-like -0.47 4.52 x 10^−5^ \<0.1
MMD2 Monocyte to macrophage differentiation-associated 2 -0.49 5.26 x 10^−5^ \<0.1
DUSP1 Dual specificity phosphatase 1 -0.7 5.6 x 10^−5^ \<0.1
OAS2 2\'-5\'-oligoadenylate synthetase 2, 69/71kDa -0.67 6.98 x 10^−5^ \<0.1
ALDH1L2 Aldehyde dehydrogenase 1 family, member L2 0.37 7.82 x 10^−5^ \<0.1
ZNF385A Zinc finger protein 385A -0.49 8.26 x 10^−5^ \<0.1
ZNF610 Zinc finger protein 610 0.4 8.71 x 10^−5^ \<0.1
PAPOLB Poly(A) polymerase beta (testis specific) 0.89 8.98 x 10^−5^ \<0.1
PPP1R3B Protein phosphatase 1, regulatory subunit 3B 0.66 8.99 x 10^−5^ \<0.1
SNORD116-24 Small nucleolar RNA, C/D box 116--24 0.64 9.02 x 10^−5^ \<0.1
ALDH3A2 Aldehyde dehydrogenase 3 family, member A2 0.3 9.64 x 10^−5^ \<0.1
GABRE Gamma-aminobutyric acid (GABA) A receptor, epsilon 1.2 9.79 x 10^−5^ \<0.1
GBAP1 Glucosidase, beta, acid pseudogene 1 -0.57 1 x 10^−4^ \<0.1
^a^Calculated in edgeR as described in Methods
In this analysis, the most significant differentially expressed gene was the nuclear receptor NR4A1 (Nur77), for which expression is reduced in schizophrenia (54%, p\<0.01, FDR\<0.1). The next most significant gene expression change was KLF4 (kruppel-like factor 4), which is reduced by a similar amount in schizophrenia (50% p\<0.01, FDR\<0.1). We confirmed the edgeR results using a different analysis program, DESeq2, which also found NR4A1 and KLF4 as the most significantly differentially expressed genes with decreases in schizophrenia similar to that found with edgeR (51% and 47%, respectively) ([S2 Table](#pone.0166944.s007){ref-type="supplementary-material"}).
To further validate these changes, quantitative PCR was used to measure the expression of these genes in the complete cohort of 74 individuals (37 schizophrenia and 37 controls). This established that both genes were significantly decreased in schizophrenia, (KLF4: F(1,69) = 8.101, p\<0.01); NR4A1: F(1,68) = 6.912, p = 0.01; [Fig 2](#pone.0166944.g002){ref-type="fig"}, [Table 4](#pone.0166944.t004){ref-type="table"}).
{#pone.0166944.g002}
10.1371/journal.pone.0166944.t004
###### Results from RT-qPCR analysis.
{#pone.0166944.t004g}
Gene F-value p-value df Mean Normalized Expression for Control Group Mean Normalized Expression for Schizophrenia Group Percentage Change (%)
------------------------------------------- ------------------- --------- ---- ---------------------------------------------- ---------------------------------------------------- ----------------------- ---------- --------
NR4A1/ Nur77 F(1, 68) = 6.912 0.011 1 4.634 (n = 36) 3.661 (n = 35) -20.99
NR4A2/ Nurr1 F(1, 66) = 4.655 0.035 1 11.624 (n = 36) 10.285 (n = 35) -11.52
NR4A3/ Nor1 F(1, 68) = 1.030 0.314 1 16.204 (n = 35) 14.994 (n = 36) -7.47
KLF4[\*](#t004fn001){ref-type="table-fn"} F(1, 69) = 8.101 0.006 1 0.864 (n = 36) 0.609 (n = 35) -37.36
VDR F(1, 70) = 0.209 0.649 1 26.381 (n = 37) 25.372 (n = 35) -3.83
RARA F(1, 66) = 0.400 0.529 1 2.26 (n = 35) 2.324 (n = 35) 2.83
RARB F(1, 66) = 0.046 0.5 1 11.038 (n = 33) 11.405 (n = 35) 3.32
RARG F(1, 62) = 2.824 0.098 1 8.457 (n = 32) 7.254 (n = 34) -14.23
RXRA F(1, 66) = 0.744 0.391 1 17.36 (n = 35) 19.049 (n = 34) 9.73
RXRB[\*](#t004fn001){ref-type="table-fn"} F(1, 66) = 10.256 0.002 1 1.772 (n = 35) 1.647 (n = 34) -24.99
RXRG F(1, 66) = 1.669 0.201 1 8.953 (n = 35) 8.035 (n = 35) -10.25
\*Log~10~ transformed data
qPCR analysis of the other NR4A sub-family and retinoid receptors {#sec009}
-----------------------------------------------------------------
*U*sing qPCR in the expanded cohort (37 schizophrenia and 37 controls), we confirmed decreased expression of NR4A1 and also found a significant decrease in NR4A2 expression in schizophrenia (NR4A2: F(1,66) = 4.655, p\<0.05) and RXRB: F(1,66) = 10.256, p\<0.01; [Fig 2](#pone.0166944.g002){ref-type="fig"}, [Table 4](#pone.0166944.t004){ref-type="table"}). We found no significant difference for NR4A3 (F(1,68) = 1.03, p\>0.05) or the RARs (A, B, and G), RXRA or RXRG (all F\<2.8, p\>0.05, [Table 4](#pone.0166944.t004){ref-type="table"}). Percentage differences in gene expression in all 10 targets examined here in the whole sample by qPCR are shown in [Fig 3](#pone.0166944.g003){ref-type="fig"}, and a comparison between control and schizophrenia groups of each of the gene expressions are shown in [S3 Fig](#pone.0166944.s003){ref-type="supplementary-material"}.
{#pone.0166944.g003}
Correlation of gene expression among transcription factors {#sec010}
----------------------------------------------------------
We performed Pearson's correlations of the mRNA expression found by qPCR for NR4 sub-family and the retinoid receptors in our extended cohort. Correlations were performed across the combined group of controls and schizophrenia patients. We found that NR4A1 mRNA was significantly correlated with the two other closely related mRNAs NR4A2 and NR4A3. RXRG mRNA was significantly correlated with the other two RXRs (A and B). RXRB mRNA was also correlated with RARG mRNA and NR4A3 mRNA was correlated with RARA mRNA ([Table 5](#pone.0166944.t005){ref-type="table"}).
10.1371/journal.pone.0166944.t005
###### Correlation of gene expression for nuclear receptors.
{#pone.0166944.t005g}
Gene Gene N p-value FDR Adjusted
------- ------- ---- ---------------- ----------------
NR4A1 NR4A2 68 1.05 x 10^−4^ 0.002
NR4A1 NR4A3 69 1.89 x 10^−15^ 1.04 x 10^−13^
NR4A3 RARA 67 0.003 0.025
RXRA RXRG 66 0.003 0.026
RXRB RARG 63 4.62 x 10^−10^ 1.27 x 10^−8^
RXRB RXRG 66 7.9 x 10^−4^ 0.011
Correlation between gene expression and age {#sec011}
-------------------------------------------
We found a negative correlation between expression of the NR4A family genes and age (NR4A1: r(71) = -0.419, p = 0.0003; NR4A2: r(71) = -0.515, p = 0.000004; NR4A3: r(71) = -0.330, p = 0.005; [S4 Fig](#pone.0166944.s004){ref-type="supplementary-material"}). This correlation in age was found in both control and schizophrenia groups, with the correlation effect stronger in the control group ([S3 Table](#pone.0166944.s008){ref-type="supplementary-material"}). There was no significant correlation between age and KLF4 or any of the retinoid receptors mRNAs.
Correlation between gene expression, and chlorpromazine dosage {#sec012}
--------------------------------------------------------------
We found a significant negative correlation between daily chlorpromazine dosage with NR4A1 and NR4A3 (NR4A1: rho(35) = -0.594, p = 0.0002; NR4A3: rho(36) = -0.438, p = 0.008) and no significant correlations between the last recorded chlorpromazine dosage to any of the mRNAs measured. There was a significant negative correlation between estimated lifetime chlorpromazine dosage and expression of NR4A1, NR4A2 and NR4A3 mRNAs (NR4A1: rho(35) = -0.601, p = 0.0001; NR4A2: rho(35) = -0.383, p = 0.023; NR4A3: rho(36) = -0.403, p = 0.015; [Fig 4](#pone.0166944.g004){ref-type="fig"}). We did not find a significant correlation with any measure of lifetime chlorpromazine and the retinoid receptor mRNAs measured (rho\<0.182, p\>0.05).
{#pone.0166944.g004}
We also found significant negative correlations between NR4A1 and NR4A2 mRNAs with duration of illness, and a trend between NR4A3 mRNA levels and duration of illness (NR4A1: rho(35) = -0.461, p = 0.005; NR4A2: rho(35) = -0.372, p = 0.028; NR4A3: rho(36) = -0.310, p = 0.067). We did not find a significant correlation between duration of illness and any retinoid receptors mRNAs measured (rho\<0.240, p\>0.05).
Because there were significant correlations between the NR4A genes with age, we re-analyzed correlations between the NR4A mRNA expressions with illness duration and lifetime dosage in a partial correlation, factoring for age. We found NR4A1 mRNA expression remained significantly correlated with the estimated lifetime antipsychotic dosage (r(32) = -0.401, p = 0.019) and a trend in NR4A3 (r(33) = -0.311, p = 0.069).
Two-way ANCOVA of diagnosis with gender {#sec013}
---------------------------------------
We found there was a decrease of RXRG mRNA in females with schizophrenia (F(1,64) = 4.97, p = 0.029) and of RARG mRNA in females with schizophrenia (F(1,60) = 3.942, p = 0.05; [S5 Fig](#pone.0166944.s005){ref-type="supplementary-material"}). There was no significant change in all other gene targets when we analyzed by two-way ANCOVA of diagnosis and gender.
Interaction network {#sec014}
-------------------
To investigate the biological effect of NR4A1, NR4A2 and RXRB down-regulation, we generated a network representation of the genes annotated as being transcriptionally regulated by the NR4 sub-family or RXRB ([Fig 5](#pone.0166944.g005){ref-type="fig"}). The downstream genes presented in [Fig 5](#pone.0166944.g005){ref-type="fig"} are involved in a broad range of cellular functions, some of which are listed in [S4 Table](#pone.0166944.s009){ref-type="supplementary-material"}.
{#pone.0166944.g005}
Generally, the annotated interactions involve transcriptional activation, although in some cases there is an inhibitory effect of the transcription factor on the target gene. We see some indication of decreased expression in genes activated by NR4A1, for instance PYGM (phosphorylase, glycogen, muscle) (26% reduction, p\<0.001, FDR\<0.2), consistent with a decrease in NR4A1 activity while genes thought to be inhibited by NR4A1, such as PPARG (peroxisome proliferator-activated receptor gamma) (30% increase, p\<0.005 FDR\<0.4), have higher levels in schizophrenia. However, the overall picture is complicated with examples of the converse also being demonstrated. This leads us to suggest that complex mechanisms are involved in the transcriptional regulation of these genes and that down-regulation of the NR4A genes and RXRB alone may not be sufficient to induce all the expected changes in downstream gene expression.
Discussion {#sec015}
==========
This study presents evidence for down-regulation of the nuclear receptors NR4A1, NR4A2, RXRB, and KLF4 mRNAs in the DLPFC in schizophrenia and evidence of reduced RARG and RXRG expression in females with schizophrenia. This study also found a negative correlation between the expression of NR4A genes and estimated levels of antipsychotic exposure. To our knowledge, this is the first study to report a correlation between clinical lifetime chlorpromazine dose and decreased expression of NR4A genes in the DLPFC. Our finding of decreased expression of NR4A1 and NR4A2 mRNA in a large cohort confirms and expands upon a previous finding in post-mortem schizophrenia in the same area of the brain \[[@pone.0166944.ref010]\], whereas decrease in KLF4 mRNA overall, and RARG and RXRG mRNAs in females with schizophrenia are reported for the first time.
The therapeutic efficacy of various antipsychotic drugs depends upon antagonism of the D2 dopamine receptors \[[@pone.0166944.ref033]\]. Schizophrenia patients who are being treated with antipsychotic medication are subjected to constant perturbation of dopamine signaling pathways. In this study, we see a highly significant diagnostic decrease in NR4A1 mRNA in the DLPFC and a lesser decrease in NR4A2 mRNA. We also find a significant negative correlation between the estimated lifetime dose of chlorpromazine and the expression of NR4A genes in the DLPFC. This is consistent with the proposition that NR4 family genes play an important role in the frontal cortex and may be regulated via cortical dopaminergic neurotransmission.
It has previously been reported that NR4A1 and NR4A3 expression increases in the murine prefrontal cortex upon a single administration of chlorpromazine \[[@pone.0166944.ref019]\]. Maheux et al. studied the effect of a number of typical and atypical neuroleptics on the expression of NR4A1 and NR4A3 in different brain regions. Overall, they found that typical antipsychotics, as distinct from atypical antipsychotics, strongly induce the expression of NR4A1 and NR4A3 in striatal areas associated with control of locomotor functions with strength of induction being correlated with the affinity of the neuroleptic drug for the D2 receptor \[[@pone.0166944.ref019]\]. Our finding of negative correlation between the mRNA of NR4A1, NR4A2 and NR4A3 with lifetime chlorpromazine dose may appear contrary to this result. However, it also suggests that chronic administration of this medication may have a different effect to acute administration. This is in line with other studies which have reported different effects on NR4A gene expression for acute versus ongoing treatment with antipsychotics. For example, the atypical antipsychotic, Clozapine, has previously been found to affect NR4A1 expression, with acute administration resulting in an increase in NR4A1 mRNA and chronic treatment (treatment over 21 days) resulting in a decrease in NR4A1 expression \[[@pone.0166944.ref018]\]. Haloperidol also increased NR4A1 expression in the dorsolateral striatum on acute treatment only \[[@pone.0166944.ref018]\]. To date, there is little evidence that antipsychotic drugs affect RXR gene expression. We found no correlation between RXR expression and chlorpromazine medication. Langlois et al. report that haloperidol had modest effects on RXRG expression in the dorsolateral portion of the striatum without any effect in other regions \[[@pone.0166944.ref034]\]. Although we found no correlation between retinoid gene expression and chlorpromazine it is possible that medication has an effect on other genes or proteins which interact with or affect the retinoid receptors and contribute to the gene expression changes reported here.
It has been proposed that NR4A1 and RXR work together as adaptive homeostatic regulators of dopamine function by reducing the effect of alterations in dopamine neurotransmission \[[@pone.0166944.ref017]\]. Given the link between NR4A genes and response to antipsychotic medication it is difficult to say whether NR4A genes are dysregulated in schizophrenia prior to commencement of antipsychotic medication. However, if dopamine signaling dysfunction is involved in the etiology of schizophrenia it is plausible that NR4A genes are vulnerable to changed expression in the development of the condition. Up or down regulation of NR4A genes is likely to have some effect on genes transcriptionally regulated by these factors. A change in NR4A can result in switching the transcriptional pathway between retinoic acid initiated (RARs) and 9-cis retinoic acid (RXRs) initiated programs \[[@pone.0166944.ref035]\] with potential perturbations in the dopaminergic pathways and in gene expression affected by dopamine.
Functions ascribed to NR4A1 in neuronal differentiation and neurite outgrowth \[[@pone.0166944.ref036], [@pone.0166944.ref037]\], learning and memory and immunity are all potentially relevant to schizophrenia. Notably, neurotrophic factors that promote neuronal survival and growth mediate their effect through receptors such as NR4A1 \[[@pone.0166944.ref038]\]. NR4A1 was first recognized through its response to nerve growth factor (NGF), which induces neuronal differentiation and neurite outgrowth \[[@pone.0166944.ref037]\]. Another neurotrophin which has also been found to be reduced in schizophrenia is brain-derived neurotrophic factor \[[@pone.0166944.ref039]\]. A potential effect on neural plasticity is also consistent with recent work indicating that the NR4A sub-family is involved in the processes of learning and memory \[[@pone.0166944.ref040], [@pone.0166944.ref041]\].
In our analysis, we found all three NR4A receptor mRNA levels to be decreased with age. The role of NR4A receptors in brain aging is currently unknown. With human brain aging, increased DNA damage is found and since NR4A receptors may protect against DNA damage \[[@pone.0166944.ref042], [@pone.0166944.ref043]\] our finding of decreased NR4A receptors with age may contribute to loss of DNA repair in brain cells, as has been observed in damaged skin cells \[[@pone.0166944.ref044]\]. Another prominent event that occurs as humans age is a decrease in metabolic rate particularly in brain \[[@pone.0166944.ref045], [@pone.0166944.ref046]\] and the down regulation of NR4A synthesis may also play a role in down-regulating cellular metabolism. In support of this, an increase in metabolism is observed in muscle cells overexpressing NR4A receptors \[[@pone.0166944.ref047]--[@pone.0166944.ref049]\]. Thus, our findings support the hypothesis that increasing NR4A transcription or function could be a potential angle to counteract some of the effects associated with human brain aging as previously proposed \[[@pone.0166944.ref042]\].
The decrease in RXRB in schizophrenia is interesting as the genetic locus of RXRB (6p21.3) has been linked to schizophrenia \[[@pone.0166944.ref009]\]. Ablation of RXRB leads to lethality in 50% of embryos indicating an important role for this gene in early development. However, surviving embryos only display mild defects, primarily male infertility related to lipid metabolism defects in Sertoli cells \[[@pone.0166944.ref050]\]. Mutant mice, with ablation of RXRB-RXRG, RARB-RXRB or RARB-RXRG have shown locomotor defects and decrease of dopamine receptors DR1 and DR2 in the ventral striatum but not in the dorsal striatum \[[@pone.0166944.ref051]\]. There is evidence that suggests DRD2 is dysregulated in schizophrenia brains \[[@pone.0166944.ref052]--[@pone.0166944.ref054]\]. Krezel et al. suggest that RXRB and RXRG may be functionally redundant in locomotion control \[[@pone.0166944.ref051]\], which is considered a functional readout of dopamine activity in the brain.
Our finding of decreased RARG and RXRG mRNA levels in females with schizophrenia may be related to changes found in estrogen and/or estrogen receptors (ER) signaling. Indeed, direct protein interaction between the retinoid receptors and the ERs via their ligand binding domain has been documented \[[@pone.0166944.ref055], [@pone.0166944.ref056]\]. Further, retinoid receptors have been shown to be regulated by estrogen/ER \[[@pone.0166944.ref057]\] through an estrogen response element (ERE) on the RAR gene promoter \[[@pone.0166944.ref058]--[@pone.0166944.ref061]\]. RARs and ER can bind to the overlapping DNA sites \[[@pone.0166944.ref062]\], which may cause antagonism. However, rather than simple antagonism, there can also be cooperation between RARs and ER in the control of gene expression \[[@pone.0166944.ref063], [@pone.0166944.ref064]\]. More work needs to be done to determine the role the retinoid receptors and ER proteins in brain neuropathology and how they may be individually or reciprocally altered in schizophrenia particularly in females.
We have also found KLF4 to be differentially expressed in schizophrenia compared to controls. KLF4 is a transcription factor in the kruppel-like factor family which regulates multiple biological functions and is involved in neurogenesis, neuronal differentiation and neurite outgrowth \[[@pone.0166944.ref065]\]. KLF4 is regulated by RARA \[[@pone.0166944.ref066]\], and it can also inhibit RARA \[[@pone.0166944.ref067]\] in vascular smooth muscle cells in a feedback-loop fashion. KLF4 mRNA and protein expression is found to be increased in skin and breast cancer \[[@pone.0166944.ref068]--[@pone.0166944.ref071]\]. In skin, RARG and RXRA are found to be antagonists of KLF4 \[[@pone.0166944.ref072]\]. Interestingly, KLF4 inhibits cell proliferation in the brain \[[@pone.0166944.ref065]\] and is down-regulated in neurogenesis \[[@pone.0166944.ref073]\]. It has been found there may be a decreased rate of cell proliferation and decreased neurogenesis in the hippocampus in schizophrenia \[[@pone.0166944.ref074]--[@pone.0166944.ref076]\]. However, the role of KLF4 in differentiated cells in the cerebral cortex is not well understood.
Transcriptional regulation by the nuclear receptors is complicated by heterodimerization and their activation by multiple ligands. Furthermore, recent genome-wide studies reveal that NR binding regions are enriched for sequence motifs of other transcription factors, such as Sp1, AP-1, and C/EBP motifs, suggesting that NRs interact with other transcription factors to regulate target gene expression \[[@pone.0166944.ref077], [@pone.0166944.ref078]\]. The NRs thus operate in a complex environment that may be tuned to provide specificity in particular tissues, cell types or environments. Quantifying and comparing the mRNA of nuclear receptors contributes to our understanding of their activity. However, a thorough investigation will also require study at the protein level. We and others have previously noted differences between protein and RNA abundance in the nuclear receptors highlighting the role of post-transcriptional regulation in these genes \[[@pone.0166944.ref006], [@pone.0166944.ref079]\]. The task of teasing out the functions of the nuclear receptors in normal and schizophrenia brains and their use as biomarkers in blook could be an area of future research. Another important research question is what cell types are expressing these nuclear receptors.
Conclusion {#sec016}
==========
This study reports significant changes in the nuclear receptors NR4A1, NR4A2, and RXRB and KLF4 in schizophrenia and provides further evidence of a role for the nuclear receptors in the disease process. Evidence is growing in support of an important role for NR4A1 and NR4A2 in neurogenesis, learning and memory, which may be associated with the role of NR4A family genes in dopaminergic pathways. Cognitive defects and changes to dopamine signaling are well known effects of schizophrenia and of current treatment protocols. These genes also play a role in immune function which is emerging as an important focus in schizophrenia research \[[@pone.0166944.ref024], [@pone.0166944.ref080], [@pone.0166944.ref081]\]. More generally this work highlights the role of a subset of nuclear receptors that link environmental cues to the genetic landscape in this complex disease.
Supporting Information {#sec017}
======================
###### MDS plot and Venn diagram of DEGs found using edgeR and DESeq2.
Multidimensional scaling (MDS) plot of all samples, SCZ samples in batch 1 (orange), Control samples in batch 1 (cyan), SCZ samples in batch 2 (red), Control samples in batch 2 (blue). (B) DEGs were identified using a glm model in both the edger and DESeq2 tools taking account of batch and SCZ, using the default settings for edgeR and DESeq2.
(TIF)
######
Click here for additional data file.
###### Venn diagram of DEGs found using edgeR with different values of prior.df.
The differentially expressed genes calculated using edgeR but varying the parameter prior.df., using the current default setting (prior.df = 10) and increasing this to prior.df = 70 (equivalent to prior.n = 2), and prior.df = 175 (equivalent to prior.n = 5) and prior.df = 350 (equivalent to prior.n = 10).
(TIF)
######
Click here for additional data file.
###### Normalized expressions of genes by diagnosis.
Overview of the normalized expressions of NR4A1, NR4A2, NR4A3, KLF4, RARA, RARB, RARG, RXRA, RXRB, and RXRG. Blue bars indicate control group and red bars indicate schizophrenia group, all showing standard error of mean (SEM). \* represents significance.
(TIF)
######
Click here for additional data file.
###### Correlations with age.
Normalized expressions of a) NR4A1 b) NR4A2 and c) NR4A3 correlated against age.
(TIF)
######
Click here for additional data file.
###### Diagnostic and Gender Differences.
Two-way ANCOVA analysis of the normalized expression of diagnosis and gender of a) RARG and b) RXRG.
(TIF)
######
Click here for additional data file.
###### Correlations between gene expressions and correlation factors.
(XLSX)
######
Click here for additional data file.
######
a\. EdgeR DE analysis using only common dispersion. b. EdgeR DE analysis using df = 350 equating to prion.n = 10. c. EdgeR DE analysis using df = 175 equating to prion.n = 5. d. EdgeR DE analysis using df = 70 equating to prion.n = 2. e. EdgeR DE analysis using current defaults (df = 10).
(ZIP)
######
Click here for additional data file.
###### Correlation between NR4A gene expressions and Age.
(XLSX)
######
Click here for additional data file.
###### Functions associated with genes downstream of NR4A family and RXRB.
(XLSX)
######
Click here for additional data file.
MRW and SC acknowledge support from the Australian Federal Government's Super Science and NCRIS Schemes, from the New South Wales State Government Science Leveraging Fund and Research Attraction and Acceleration Program and from the University of New South Wales.
This work was supported by Schizophrenia Research Institute (utilizing infrastructure funding from the NSW Ministry of Health and the Macquarie Group Foundation), the University of New South Wales, and Neuroscience Research Australia. CSW is a recipient of a National Health and Medical Research Council (Australia) Senior Research Fellowship (\#1021970). Shan-Yuan Tsai is supported by The Cowled Postgraduate Research Scholarship in Brain Research. Tissues were received from the New South Wales Brain Tissue Resource Centre at the University of Sydney which is supported by the Schizophrenia Research Institute and National Institute of Alcohol Abuse and Alcoholism (NIH (NIAAA) R28AA012725).
[^1]: **Competing Interests:**CSW is a panel member of Lundbeck Australia Advisory Board and in collaboration with Astellas Pharma Inc., Japan. This does not alter our adherence to PLOS ONE policies on sharing data and materials.
[^2]: **Conceptualization:** CSW MRW.**Data curation:** MRW SMC.**Formal analysis:** SMC SYT.**Funding acquisition:** CSW MRW.**Investigation:** SMC SYT.**Methodology:** CSW MRW SMC SYT.**Project administration:** CSW MRW.**Resources:** CSW MRW SMC SYT.**Software:** SMC MRW.**Supervision:** CSW MRW.**Validation:** CSW MRW SMC SYT.**Visualization:** SMC SYT.**Writing -- original draft:** SMC SYT MRW CSW.**Writing -- review & editing:** SMC SYT MRW CSW.
[^3]: ‡ These authors are co-first authors on this work.
| 50,334,125 |
---
abstract: |
Recommender systems are tools that support online users by pointing them to potential items of interest in situations of information overload. In recent years, the class of session-based recommendation algorithms received more attention in the research literature. These algorithms base their recommendations solely on the observed interactions with the user in an ongoing session and do not require the existence of long-term preference profiles. Most recently, a number of deep learning based (“neural”) approaches to session-based recommendations were proposed. However, previous research indicates that today’s complex neural recommendation methods are not always better than comparably simple algorithms in terms of prediction accuracy.
With this work, our goal is to shed light on the state-of-the-art in the area of session-based recommendation and on the progress that is made with neural approaches. For this purpose, we compare twelve algorithmic approaches, among them six recent neural methods, under identical conditions on various datasets. We find that the progress in terms of prediction accuracy that is achieved with neural methods is still limited. In most cases, our experiments show that simple heuristic methods based on nearest-neighbors schemes are preferable over conceptually and computationally more complex methods. Observations from a user study furthermore indicate that recommendations based on heuristic methods were also well accepted by the study participants. To support future progress and reproducibility in this area, we publicly share the <span style="font-variant:small-caps;"></span> evaluation framework that was used in our research.[^1]
author:
- Malte Ludewig
- Noemi Mauro
- Sara Latifi
- Dietmar Jannach
bibliography:
- 'article.bib'
subtitle: 'A Comparison of Neural and Non-Neural Approaches'
title: 'Empirical Analysis of Session-Based Recommendation Algorithms'
---
<ccs2012> <concept> <concept\_id>10002951.10003317.10003347.10003350</concept\_id> <concept\_desc>Information systems Recommender systems</concept\_desc> <concept\_significance>500</concept\_significance> </concept> <concept> <concept\_id>10002944.10011123.10011130</concept\_id> <concept\_desc>General and reference Evaluation</concept\_desc> <concept\_significance>500</concept\_significance> </concept> <concept> <concept\_id>10010147.10010257.10010293.10010294</concept\_id> <concept\_desc>Computing methodologies Neural networks</concept\_desc> <concept\_significance>300</concept\_significance> </concept> </ccs2012>
Introduction {#sec:introduction}
============
Recommender systems (RS) are software applications that help users in situations of information overload and they have become a common feature on many modern online services. Collaborative filtering (CF) techniques, which are based on behavioral data collected from larger user communities, are among the most successful technical approaches in practice. Historically, these approaches mostly rely on the assumption that information about longer-term preferences of the individual users are available, e.g., in the form of a user-item rating matrix [@Resnick:1994:GOA:192844.192905]. In many real-world applications, however, such longer-term information is often not available, because users are not logged in or because they are first-time users. In such cases, techniques that leverage behavioral patterns in a community can still be applied [@JannachZankerCF2018]. The difference is that instead of the long-term preference profiles only the observed interactions with the user in the ongoing session can be used to adapt the recommendations to the assumed needs, preferences, or intents of the user. Such a setting is usually termed a *session-based recommendation* problem [@QuadranaetalCSUR2018].
Interestingly, research on session-based recommendation was very scarce for many years despite the high practical relevance of the problem setting. Only in recent years, we can observe an increased interest in the topic in academia [@DBLP:journals/corr/abs-1902-04864], which is at least partially caused by the recent availability of public datasets in particular from the e-commerce domain. This increased interest in session-based recommendations coincides with the recent boom of deep learning (neural) methods in various application areas. Accordingly, it is not surprising that several neural session-based recommendation approaches were proposed in recent years, with [<span style="font-variant:small-caps;">gru4rec</span>]{}being one of the pioneering and most cited works in this context [@Hidasi2016GRU]. From the perspective of the evaluation of session-based algorithms, the research community—at the time when the first neural techniques were proposed—had not yet established a level of maturity as is the case for problem setups that are based on the traditional user-item rating matrix. This led to challenges that concerned both the question what represents the state-of-the-art in terms of algorithms and the question of the evaluation protocol when time-ordered user interaction logs are the input instead of a rating matrix. Partly due to this unclear situation, it soon turned out that in some cases comparably simple non-neural techniques, in particular ones based on nearest-neighbors approaches, can lead to very competitive or even better results than neural techniques [@JannachLudewig2017RecSys; @Ludewig2018]. Besides being competitive in terms of accuracy, such more simple approaches often have the advantage that their recommendations are more transparent and can more easily be explained to the users. Furthermore, these simpler methods can often be updated online when new data becomes available, without requiring expensive model retraining.
However, during the last few years after the publication of [<span style="font-variant:small-caps;">gru4rec</span>]{}, we have mostly observed new proposals in the area of complex models. With this work, our aim is to assess the progress that was made in the last few years in a reproducible way. To that purpose, we have conducted an extensive set of experiments in which we compared twelve session-based recommendation techniques under identical conditions on a number of datasets. Among the examined techniques, there are six recent neural approaches, which were published at highly-ranked publication outlets such as KDD, AAAI, or SIGIR after the publication of the first version of [<span style="font-variant:small-caps;">gru4rec</span>]{}in 2015.[^2]
The main outcome of our offline experiments is that the progress that is achieved with neural approaches to session-based recommendation is still limited. In most experiment configurations, one of the simple techniques outperforms all the neural approaches. In some cases, we could also not confirm that a more recently proposed neural method consistently outperforms the much earlier [<span style="font-variant:small-caps;">gru4rec</span>]{}method. Generally, our analyses point to certain underlying methodological issues, which were also observed in other application areas of applied machine learning. Similar observations regarding the competitiveness of established and often more simple approaches were made before, e.g., for the domains of information retrieval, time-series forecasting, and recommender systems, [@Yang:2019:CEH:3331184.3331340; @Ferraridacremaetal2019; @Makridakis2018; @Armstrong:2009:IDA:1645953.1646031], and it is important to note that these phenomena are not tied to deep learning approaches.
To help overcome some of these problems for the domain of session-based recommendation, we share our evaluation framework <span style="font-variant:small-caps;"></span> online[^3]. The framework not only includes the algorithms that are compared in this paper, it also supports different evaluation procedures, implements a number of metrics, and provides pointers to the public datasets that were used in our experiments.
Since offline experiments cannot inform us about the quality of the recommendation as *perceived* by users, we have furthermore conducted a user study. In this study, we compared heuristic methods with a neural approach and the recommendations produced by a commercial system (<span style="font-variant:small-caps;"></span>) in the context of an online radio station. The main outcomes of this study are that heuristic methods also lead to recommendations—playlists in this case—that are well accepted by users. The study furthermore sheds some light on the importance of other quality factors in the particular domain, i.e., the capability of an algorithm to help users discover new items.
The paper is organized as follows. Next, in Section \[sec:algorithms\], we provide an overview of the algorithms that were used in our experiments. Section \[sec:methodology\] describes our offline evaluation methodology in more detail and Section \[sec:results\] presents the outcomes of the experiments. In Section \[sec:user-study\], we report the results of our user study. Finally, we summarize our findings and their implications in Section \[sec:discussion\].
Algorithms {#sec:algorithms}
==========
Algorithms of various types were proposed over the years for session-based recommendation problems. A detailed overview of the more general family of *sequence-aware recommender systems*, where session-based ones are a part of, can be found in [@QuadranaetalCSUR2018]. In the context of this work, we limit ourselves to a brief summary of parts of the historical development and how we selected algorithms for inclusion in our evaluations.
Historical Development and Algorithm Selection
----------------------------------------------
Nowadays, different forms of session-based recommendations can be found in practical applications. The recommendation of *related items* for a given reference object can, for example, be seen as a basic and very typical form of session-based recommendations in practice. In such settings, the selection of the recommendations is usually based solely on the very last item viewed by the user. Common examples are the recommendation of additional articles on news web sites or recommendations of the form “Customers who bought …also bought” on e-commerce sites. Another common application scenario is the creation of automated playlists, e.g., on YouTube, Spotify, or last.fm. Here, the system creates a virtually endless list of next-item recommendations based on some seed item and additional observations, e.g., skips or likes, while the media is played. These application domains—web page and news recommendation, e-commerce, music playlists—also represent the main driving scenarios in academic research.
For the recommendation of *web pages* to visit, Mobasher et al. proposed one of the earliest session-based approaches based on frequent pattern mining in 2002 [@Mobasher2002]. In 2005, Shani et al. [@shani05mdp] investigated the use of an MDP-based (Markov Decision Process) approach for session-based recommendations in *e-commerce* and also demonstrated its value from a business perspective. Alternative technical approaches based on Markov processes were later on proposed in 2012 and 2013 for the *news* domain in [@DBLP:conf/recsys/GarcinDF13] and [@DBLP:conf/webi/GarcinZFS12].
A early approach to *music playlist generation* was proposed in 2005 [@Ragno:2005:ISM:1101826.1101840], where the selection of items was based on the similarity with a seed song. The music domain was however also very important for collaborative approaches. In 2012, the authors of [@hariri12context] used a session-based nearest-neighbors technique as part of their approach for playlist generation. This nearest-neighbors method and improved versions thereof later on turned out to be highly competitive with today’s neural methods [@Ludewig2018]. More complex methods were also proposed for the music domain, e.g., an approach based on Latent Markov Embeddings [@Chen:2012:PPV:2339530.2339643] from 2012. Some novel technical proposals in the years 2014 and 2015 were based on a non-public *e-commerce* dataset from a European fashion retailer and either used Markov processes and side information [@tavakol14fmdp] or a simple re-ranking scheme based on short-term intents [@Jannach2015]. More importantly, however, in the year 2015, the ACM RecSys conference hosted a challenge, where the problem was to predict if a consumer will make a purchase in a given session, and if so, to predict which item will be purchased. A corresponding dataset (YOOCHOOSE) was released by an industrial partner, which is very frequently used today for benchmarking session-based algorithms. Technically, the winning team used a two-stage classification approach and invested a lot of effort into feature engineering to make accurate predictions [@Romov:2015:RCE:2813448.2813510].
In late 2015, Hidasi et al. [@Hidasi2016GRU] then published the probably first deep learning based method for session-based recommendation called [<span style="font-variant:small-caps;">gru4rec</span>]{}, a method which was continuously improved later on, e.g., in [@Hidasi:2018:RNN:3269206.3271761] or [@Tan2016GruPlus]. In their work, they also use the mentioned YOOCHOOSE dataset for evaluation, although with the slightly different optimization goal, i.e., to predict the immediate next item click event. As one of their baselines, they used an item-based nearest-neighbors technique. They found that their neural method is significantly better than this technique in terms of prediction accuracy. The proposal of their method and the booming interest in neural approaches subsequently led to a still ongoing wave of new proposals that apply deep learning approaches to session-based recommendation problems.
In this present work, we consider a selection of algorithms that reflects these historical developments. We consider basic algorithms based on item co-occurrences, sequential patterns and Markov processes as well as methods that implement session-based nearest-neighbors techniques. Looking at neural approaches, we benchmark the latest versions of [<span style="font-variant:small-caps;">gru4rec</span>]{}as well as five other methods that were published later and which state that they outperform at least the initial version of [<span style="font-variant:small-caps;">gru4rec</span>]{}to a significant extent. Regarding the selected neural approaches, we limit ourselves to methods that do not use side information about the items in order to make our work easily reproducible and not dependent on such meta-data. Another constraint for the inclusion in our comparison is that the work was published in one of the major conferences, i.e., one that is rated A or A\* according to the Australian CORE scheme. Finally, while in theory algorithms should be reproducible based on the technical descriptions in the paper, there are usually many small implementation details that can influence the outcome of the measurement. Therefore, like in [@Ferraridacremaetal2019], we only considered approaches where the source code was available and could be integrated in our evaluation framework with reasonable effort.
Considered Algorithms
---------------------
In total, we considered 12 algorithms in our comparison. Table \[tab:non-neural-baselines\] provides an overview of the *non-neural* methods. Table \[tab:neural-methods\] correspondingly shows the neural methods considered in our analysis, ordered by their publication date.
[1]{}[@p[1.1cm]{}X@]{} [<span style="font-variant:small-caps;">ar</span>]{}& This simple “Association Rules” method counts pairwise item co-occurrences in the training sessions. Recommendations for an ongoing session are generated by this method by returning those items that most frequently co-occurred with the last item of the current session in the past. For a formal definition, see [@Ludewig2018].\
[<span style="font-variant:small-caps;">sr</span>]{}& This method called “Sequential Rules” was proposed in [@Ludewig2018]. It is similar to [<span style="font-variant:small-caps;">ar</span>]{}in that it counts pairwise item co-occurrences in the training sessions. In addition to [<span style="font-variant:small-caps;">ar</span>]{}, however, it considers the order of the items in a session and the distance between them using a decay function. The method often led to competitive results in particular in terms of the Mean Reciprocal Rank in the analysis in [@Ludewig2018].\
[<span style="font-variant:small-caps;">sknn</span>]{}/[<span style="font-variant:small-caps;">v-sknn</span>]{}& The analysis in [@JannachLudewig2017RecSys] showed that a simple session-based nearest-neighbors method similar to the one from [@Hariri2015] was competitive with the first version for [<span style="font-variant:small-caps;">gru4rec</span>]{}. Conceptually, the idea is to find past sessions that contain the same elements as the ongoing session. The recommendations are then based by selecting items that appeared in the most similar past session. Since the sequence in which items are consumed in the ongoing user session might be of importance in the recommendation process, a number of “sequential extensions” to the [<span style="font-variant:small-caps;">sknn</span>]{}method were proposed in [@Ludewig2018]. Here, the order of the items in a session proved to be helpful, both when calculating the similarities as well as in the item scoring process. Furthermore, according to [@Ludewig2018rsc] it can be beneficial to put more emphasis on less popular items by applying an Inverse-Document-Frequency(IDF) weighting scheme. In this paper, all those extensions are implemented in the [<span style="font-variant:small-caps;">v-sknn</span>]{}method.\
[<span style="font-variant:small-caps;">stan</span>]{}& This method called “Sequence and Time Aware Neighborhood” was presented at SIGIR ’19 [@Garg:2019]. [<span style="font-variant:small-caps;">stan</span>]{}is based on [<span style="font-variant:small-caps;">sknn</span>]{}[@JannachLudewig2017RecSys], but it additionally takes into account the following factors for making recommendations: i) the position of an item in the current session, ii) the recency of a past session w.r.t. to the current session, and iii) the position of a recommendable item in a neighboring session. Their results show that [<span style="font-variant:small-caps;">stan</span>]{}significantly improves over [<span style="font-variant:small-caps;">sknn</span>]{}, and is even comparable to recently proposed state-of-the-art deep learning approaches.\
[<span style="font-variant:small-caps;">vstan</span>]{}& This method, which we propose in this present paper, combines the ideas from [<span style="font-variant:small-caps;">stan</span>]{}and [<span style="font-variant:small-caps;">v-sknn</span>]{}in a single approach. It incorporates all three previously mentioned particularities of [<span style="font-variant:small-caps;">stan</span>]{}, which already share some similarities with the [<span style="font-variant:small-caps;">v-sknn</span>]{}method. Furthermore, we add a sequence-aware item scoring procedure as well as the IDF weighting scheme from [<span style="font-variant:small-caps;">v-sknn</span>]{}.\
[<span style="font-variant:small-caps;">ct</span>]{}& This technique is based on Context Trees, which were originally proposed for lossless data compression. It is a non-parametric method and based on variable-order Markov models. The method was proposed in [@Mi2018ct], where it showed promising results.\
[1]{}[@p[1.7cm]{}X@]{} [<span style="font-variant:small-caps;">gru4rec</span>]{}& [<span style="font-variant:small-caps;">gru4rec</span>]{}[@Hidasi2016GRU] was the first neural approach that employed RNNs for session-based recommendation. This technique uses Gated Recurrent Units (GRU) [@DBLP:journals/corr/ChoMBB14] to deal with the vanishing gradient problem. The technique was later on improved using more effective loss functions [@Hidasi:2018:RNN:3269206.3271761].\
[<span style="font-variant:small-caps;">narm</span>]{}& This model [@Li2017narm] extends [<span style="font-variant:small-caps;">gru4rec</span>]{}and improves its session modeling with the introduction of a hybrid encoder with an attention mechanism. The attention mechanism is in particular used to consider items that appeared earlier in the session and which are similar to the last clicked one. The recommendation scores for each candidate item are computed with a bilinear matching scheme based on the unified session representation.\
[<span style="font-variant:small-caps;">stamp</span>]{}& In contrast to [<span style="font-variant:small-caps;">narm</span>]{}, this model [@Liu2018stamp] does not rely on an RNN. A short-term attention/memory priority model is proposed, which is (a) capable of capturing the users’ general interests from the long-term memory of a session context, and which (b) also takes the users’ most recent interests from the short-term memory into account. The users’ general interests are captured by an external memory built from all the historical clicks in a session prefix (including the last click). The attention mechanism is built on top of the embedding of the last click that represents the user’s current interests.\
[<span style="font-variant:small-caps;">nextitnet</span>]{}& This recent model [@Yuan2019nextitnet] also discards RNNs to model user sessions. In contrast to [<span style="font-variant:small-caps;">stamp</span>]{}, convolutional neural networks are adopted with a few domain-specific enhancements. The generative model is designed to explicitly encode item inter-dependencies, which allows to directly estimate the distribution of the output sequence (rather than the desired item) over the raw item sequence. Moreover, to ease the optimization of the deep generative architecture, the authors propose to use residual networks to wrap convolutional layer(s) by residual block.\
[<span style="font-variant:small-caps;">sr</span><span style="font-variant:small-caps;">gnn</span>]{}& This method [@DBLP:journals/corr/abs-1811-00855] models session sequences as graph structured data (i.e., directed graphs). Based on the session graph, [<span style="font-variant:small-caps;">sr</span><span style="font-variant:small-caps;">gnn</span>]{}is capable of capturing transitions of items and generating item embedding vectors correspondingly, which are difficult to be revealed by conventional sequential methods like MC-based and RNN-based methods. With the help of item embedding vectors, [<span style="font-variant:small-caps;">sr</span><span style="font-variant:small-caps;">gnn</span>]{}furthermore aims to construct reliable session representations from which the next-click item can be inferred.\
[<span style="font-variant:small-caps;">csrm</span>]{}& This method [@Wang:2019:CSR:3331184.3331210] is a hybrid framework that uses collaborative neighborhood information in session-based recommendations. [<span style="font-variant:small-caps;">csrm</span>]{}consists of two parallel modules: an Inner Memory Encoder (IME) and an Outer Memory Encoder (OME). The IME models a user’s own information in the current session with the help of Recurrent Neural Networks (RNNs) and an attention mechanism. The OME exploits collaborative information to better predict the intent of current sessions by investigating neighborhood sessions. Then, a fusion gating mechanism is used to selectively combine information from the IME and OME to obtain the final representation of the current session. Finally, [<span style="font-variant:small-caps;">csrm</span>]{}obtains a recommendation score for each candidate item by computing a bi-linear match with the final representation of the current session.\
Except for the [<span style="font-variant:small-caps;">ct</span>]{}method, the non-neural methods from Table \[tab:non-neural-baselines\] are conceptually very simple or almost trivial. As mentioned above, this can lead to a number of potential practical advantages compared to more complex models, e.g., regarding online updates and explainability. From the perspective of the computational costs, the time needed to “train” the simple methods is often low, as this phase often reduces to counting item co-occurrences in the training data or to preparing some in-memory data structures. To make the nearest-neighbors technique scalable, we implemented the internal data structures and data sampling strategies proposed in [@JannachLudewig2017RecSys]. As a result, the [<span style="font-variant:small-caps;">ct</span>]{}method is the only one from the set of non-neural methods for which we encountered scalability issues in the form of memory consumption and prediction time when the set of recommendable items is huge.
Regarding alternative non-neural approaches, note that in the evaluation in [@Ludewig2018], a number of additional methods were considered. We do not include these methods ([<span style="font-variant:small-caps;">iknn</span>]{}, [<span style="font-variant:small-caps;">fpmc</span>]{}, [<span style="font-variant:small-caps;">mc</span>]{}, [<span style="font-variant:small-caps;">smf</span>]{}, [<span style="font-variant:small-caps;">bpr-mf</span>]{}, [<span style="font-variant:small-caps;">fism</span>]{}, [<span style="font-variant:small-caps;">fossil</span>]{}) in our present analysis, because previous research showed that these methods either are generally not competitive or only lead to competitive results in a few special cases.
[1]{}[@Xlcccccccc@]{} Method & Publication & [<span style="font-variant:small-caps;">iknn</span>]{}& [<span style="font-variant:small-caps;">sknn</span>]{}& [<span style="font-variant:small-caps;">bpr-mf</span>]{}& [<span style="font-variant:small-caps;">fpmc</span>]{}& [<span style="font-variant:small-caps;">gru4rec</span>]{}& [<span style="font-variant:small-caps;">narm</span>]{}& [<span style="font-variant:small-caps;">stamp</span>]{}\
[<span style="font-variant:small-caps;">gru4rec</span>]{}& ICLR (05/16) & & & & & & &\
[<span style="font-variant:small-caps;">gru4rec+</span>]{}& RecSys (09/16) & & & & & & & &\
[<span style="font-variant:small-caps;">narm</span>]{}& CIKM (11/17) & & & & & & &\
[<span style="font-variant:small-caps;">stamp</span>]{}& KDD (08/18) & & & & & & &\
[<span style="font-variant:small-caps;">gru4rec2</span>]{}& CIKM (10/18) & & & & & & &\
[<span style="font-variant:small-caps;">nextitnet</span>]{}& WSDM (02/19) & & & & & & &\
[<span style="font-variant:small-caps;">sr</span><span style="font-variant:small-caps;">gnn</span>]{}& AAAI (02/19) & & & & & & &\
[<span style="font-variant:small-caps;">csrm</span>]{}& SIGIR (07/19) & & & & & & &\
The development over time regarding the *neural* approaches is summarized in Table \[tab:used-baselines-neural-approaches\]. The table also indicates which baselines were used in the original papers. The analysis shows that [<span style="font-variant:small-caps;">gru4rec</span>]{}was considered as a baseline in all papers. Most papers refer to the original [<span style="font-variant:small-caps;">gru4rec</span>]{}publication from 2016 or an early improved version that was proposed shortly afterwards (which we term [<span style="font-variant:small-caps;">gru4rec+</span>]{}here, see [@Tan2016GruPlus]). Most papers, however, do not refer to the improved version ([<span style="font-variant:small-caps;">gru4rec2</span>]{}) discussed in [@Hidasi:2018:RNN:3269206.3271761]. Since the public code for [<span style="font-variant:small-caps;">gru4rec</span>]{}was constantly updated, we however assume that the authors ran benchmarks against the updated versions. [<span style="font-variant:small-caps;">narm</span>]{}, as one of the earlier neural techniques, is the only neural method other than [<span style="font-variant:small-caps;">gru4rec</span>]{}that is considered quite frequently by more recent works.
The analysis of the used baselines furthermore showed that only one of the more recent papers proposing a neural method considers, i.e., [@Wang:2019:CSR:3331184.3331210], session-based nearest-neighbors techniques as a baseline, even though their competitiveness was documented in a publication at the ACM Recommender Systems conference in 2017 [@JannachLudewig2017RecSys]. The authors of [@Wang:2019:CSR:3331184.3331210] however only consider the original proposal and not the improved versions from 2018 [@Ludewig2018]. The only other papers in our analysis, which consider session-based nearest-neighbors techniques as baselines, are about non-neural techniques ([<span style="font-variant:small-caps;">ct</span>]{}and [<span style="font-variant:small-caps;">stan</span>]{}). The paper proposing [<span style="font-variant:small-caps;">stan</span>]{}furthermore is an exception in that since it considers quite a number of neural approaches ([<span style="font-variant:small-caps;">gru4rec2</span>]{}, [<span style="font-variant:small-caps;">stamp</span>]{}, [<span style="font-variant:small-caps;">narm</span>]{}, [<span style="font-variant:small-caps;">sr</span><span style="font-variant:small-caps;">gnn</span>]{}) in its comparison.
Evaluation Methodology {#sec:methodology}
======================
We benchmarked all methods under the same conditions, using the evaluation framework that we share online to ensure reproducibility of our results.
Datasets {#subsec.datasets}
--------
We considered eight datasets from two domains for our evaluation, e-commerce and music. Six of them are public and several of them were previously used to benchmark session-based recommendation algorithms. Table \[tab:datasets\] briefly describes the datasets.
\[h!t\]
[1]{}[@lX@]{} [RSC15]{}& E-commerce dataset used in the 2015 ACM RecSys Challenge.\
[RETAIL]{}& An e-commerce dataset from the company Retail Rocket.\
[DIGI]{}& An e-commerce dataset shared by the company Diginetica.\
[ZALANDO]{}& A non-public dataset consisting of interaction logs from the European fashion retailer Zalando.\
[30MU]{}& Music listening logs obtained from Last.fm.\
[NOWP]{}& Music listening logs obtained from Twitter.\
[AOTM]{}& A public music dataset containing music playlists.\
[8TRACKS]{}& A private music dataset with hand-crafted playlists.\
We pre-processed the original datasets in a way that all sessions with only one interaction were removed. As done in previous works, we also removed from sessions items that appeared less than 5 times in the dataset. Furthermore, we use an evaluation procedure where we run repeated measurements on several subsets (splits) of the original data, see Section \[subsec:evaluation-procedure\]. The average characteristics of the subsets for each dataset are shown in Table \[tab:dataset-characteristics\]. We share all datasets except [ZALANDO]{}and [8TRACKS]{}online.
[1]{}[@Xrrrrrrrr@]{} Dataset & [RSC15]{}& [RETAIL]{}& [DIGI]{}& [ZALANDO]{}& [30MU]{}& [NOWP]{}& [AOTM]{}& [8TRACKS]{}\
Actions & 5.4M & 210k & 264k & 4.5M & 640k & 271k & 307k & 1.5M\
Sessions & 1.4M & 60k & 55k & 365k & 37k & 27k & 22k & 132k\
Items & 29k & 32k & 32k & 189k & 91k & 75k & 91k & 376k\
Days cov. & 31 & 27 & 31 & 90 & 90 & 90 & 90 & 90\
Actions/Sess. & 3.95 & 3.54 & 4.78 & 12.43 & 17.11 & 10.04 & 14.02 & 11.32\
Items/Sess. & 3.17 & 2.56 & 4.01 & 8.39 & 14.47 & 9.38 & 14.01 & 11.31\
Actions/Day & 175k & 8k & 8.5k & 50k & 7k & 3.0k & 3.4k & 16.6k\
Sessions/Day & 44k & 2.2k & 1.7k & 4k & 300 & 243 & 243 & 1.4k\
Evaluation Procedure and Metrics {#subsec:evaluation-procedure}
--------------------------------
#### Data Splitting Approach.
We apply the following procedure to create train-test splits. Since most datasets consist of time-ordered events, usual cross-validation procedures with the randomized allocation of events across data splits cannot be applied. Several authors only use one single time-ordered training-test split for their measurements. This, however, can lead to undesired random effects. We therefore rely on a protocol where we create five non-overlapping and contiguous subsets (splits) of the datasets. As done in previous works, we use the last *n* days of each split for evaluation (testing) and the other days for training the models.[^4] The reported measurements correspond to the averaged results obtained for each split.
The playlist datasets ([AOTM]{}and [8TRACKS]{}) are exceptions here as they do not have timestamps. For these datasets, we therefore randomly generated timestamps, which allows us to use the same procedure as for the other datasets.
#### Hyper-parameter Optimization.
Proper hyper-parameter tuning is essential when comparing machine learning approaches. We therefore tuned all hyper-parameters for all methods and datasets in a systematic approach, using MRR@20 as an optimization target as done in previous works. Technically, we created subsets from the training data for validation. The size of the validation set was chosen in a way that it covered the same number of days that was used in the final test set. We applied a random hyper-parameter optimization approach with 100 iterations as done in [@Hidasi:2018:RNN:3269206.3271761; @Liu2018stamp; @Li2017narm]. Since [<span style="font-variant:small-caps;">narm</span>]{}and [<span style="font-variant:small-caps;">csrm</span>]{}only have a smaller set of hyper-parameters, we only had to do 50 iterations for these methods. For the [<span style="font-variant:small-caps;">sr</span><span style="font-variant:small-caps;">gnn</span>]{}method, we had to limit the number of iterations for the [ZALANDO]{}dataset to 40, because tuning was particularly time-consuming. The final hyper-parameter values for each method and dataset can be found online, along with a description of the investigated ranges.
#### Accuracy Measures.
For each session in the test set, we incrementally reveal one event of a session after the other, as was proposed in [@Hidasi2016GRU]. The task of the recommendation algorithm is to generate a prediction for the next event(s) in the session in the form of a ranked list of items. The resulting list can then be used to apply standard accuracy measures from information retrieval. The measurement can be done in two different ways.
- As in [@Hidasi2016GRU] and other works, we can measure if the immediate next item is part of the resulting list and at which position it is ranked. The corresponding measures are the Hit Rate and the Mean Reciprocal Rank.
- In typical information retrieval scenarios, however, one is usually not interested in having one item right (e.g., the first search result), but in having as many predictions as possible right in a longer list that is displayed to the user. For session-based recommendation scenarios, this applies as well, as usually, e.g., on music and e-commerce sites, more than one recommendation is displayed. Therefore, we measure Precision and Recall in the usual way, by comparing the objects of the returned list with the entire remaining session, assuming that not only the immediate next item is relevant for the user. In addition to Precision and Recall, we also report the Mean Average Precision metric.
The most common cut-off threshold in the literature is 20, probably because this was the chosen threshold by the authors of [<span style="font-variant:small-caps;">gru4rec</span>]{}[@Hidasi2016GRU]. We have made measurements for alternative list lengths as well, but will only report the results when using 20 as a list length in this paper. We report additional results for cut-off thresholds of 5 and 10 in an online appendix.[^5]
#### Coverage and Popularity.
Depending on the application domain, factors other than prediction accuracy might be relevant as well, including coverage, novelty, diversity, or serendipity [@Shani2011]. Since we do not have information about item characteristics, we focus on questions of coverage and novelty in this work.
With *coverage*, we here refer to what is sometimes called “aggregate diversity” [@Adomavicius:2012:IAR:2197072.2197127]. Specifically, we measure the fraction of items of the catalog that ever appears in any top-n list presented to the users in the test set. This coverage measure in some ways also measures the level of context adaptation, i.e., if an algorithm tends to recommend the same set of items to everyone or specifically varies the recommendations for a given session.
We approximate the *novelty* level of an algorithm by measuring how popular the recommended items are on average. The underlying assumption is that recommending more unpopular items leads to higher novelty and discovery effects. Algorithms that mostly focus on the recommendation of popular items might be undesirable from a business perspective, e.g., when the goal is to leverage the potential of the long tail in e-commerce settings. Technically, we measure the *popularity* level of an algorithm as follows. First, we compute min-max normalized popularity values of each item in the training set. Then, during evaluation, we compute the popularity level of an algorithm by determining the average popularity value of each item that appears in its top-n recommendation list. Higher values correspondingly mean that an algorithm has a tendency to recommend rather popular items.
#### Running Times.
Complex neural models can need substantial computational resources to be trained. Training a “model”, i.e., calculating the statistics, for co-occurrence based approaches like [<span style="font-variant:small-caps;">sr</span>]{}or [<span style="font-variant:small-caps;">ar</span>]{}can, in contrast, be done very efficiently. For nearest-neighbors based approaches, actually no model is learned at all. Instead, some of our nearest-neighbors implementations need some time to create internal data structures that allow for efficient recommendation at prediction time. In the context of this paper, we will report running times for some selected datasets from e-commerce.
We executed all experiments on the same physical machine. The running times for the neural methods were determined using a GPU; the non-neural methods used a CPU. In theory, running times should be compared on the same hardware. Thererfore, since the running times of the neural methods are much longer even when a GPU can be used, we can assume that the true difference in computational complexity is in fact even higher than we can see in our measurements.
#### Stability with Respect to New Data
In some application domains, e.g., news recommendation or e-commerce, new user-item interaction data can come in at a high rate. Since retraining the models to accommodate the new data can be costly, a desirable characteristic of an algorithm can be that the performance of the model does not degenerate too quickly before the retraining happens. To put it differently, it is desirable that the models do not overfit too much to the training data.
To investigate this particular form of model stability, we proceeded as follows. First, we trained a model on the training data $T_0$ of a given train-test split[^6]. Then, we made measurements using two different protocols, which we term *retraining* and *no-retraining*, respectively.
- In the *retraining* configuration, we first evaluated the model that was trained on $T_0$ using the data of the first day of the test set. Then, we added this first day of the test set to $T_0$ and retrained the model on this extended dataset, which we name $T_1$. Then, we continued with the evaluation with the data from the second day of the test data, using the model trained on $T_1$. This process of adding more data to the training set, retraining the full model, and evaluating on the next day of the test set was done for all days of the test set.
- In the *no-retraining* configuration, we also evaluated the performance day by day on the test data, but did not retrain the models, i.e., we used the model trained on $T_0$ for all days in the test data.
To enable a fair comparison in both configurations, we only considered items in the evaluation phase that appeared at least once in the original training data $T_0$.
Note that the absolute accuracy values for a given test day depends on the characteristics of the recorded data on that day. In some cases, the accuracy for the second test day can therefore even be higher than for the first test day, even if there was no retraining. An exact comparison of absolute values is therefore not too meaningful. However, we consider the *relative* accuracy drop when using the initial model $T_0$ for a number of consecutive days as an indicator of the generalizability or stability of the learned models, provided that the investigated algorithms start from a comparable accuracy level.
\[tab:results-ec\]
[1]{}[@Xrrr|rr|rr@]{} Metrics & MAP@20 & P@20 & R@20 & HR@20 & MRR@20 & COV@20 & POP@20\
\
[<span style="font-variant:small-caps;">stan</span>]{}& [**0.0285**]{} & [**0.0543**]{} & [**0.4748**]{} & [**0.5938**]{} & [$*$**0.3638**]{} & 0.5929 & 0.0518\
[<span style="font-variant:small-caps;">vstan</span>]{}& 0.0284 & 0.0542 & 0.4741 & 0.5932 & 0.3636 & & 0.0488\
[<span style="font-variant:small-caps;">sknn</span>]{}& 0.0283 & 0.0532 & 0.4707 & 0.5788 & 0.3370 & 0.5709 & 0.0540\
[<span style="font-variant:small-caps;">v-sknn</span>]{}& 0.0278 & 0.0531 & 0.4632 & 0.5745 & 0.3395 & 0.5562 & 0.0598\
*[<span style="font-variant:small-caps;">gru4rec</span>]{}* & & & & & 0.3237 & [$*$**0.7973**]{} & [**0.0347**]{}\
*[<span style="font-variant:small-caps;">narm</span>]{}* & 0.0270 & 0.0501 & 0.4526 & 0.5549 & 0.3196 & 0.6472 & 0.0569\
*[<span style="font-variant:small-caps;">csrm</span>]{}* & 0.0252 & 0.0467 & 0.4246 & 0.5169 & 0.2955 & 0.6049 & 0.0496\
*[<span style="font-variant:small-caps;">sr</span><span style="font-variant:small-caps;">gnn</span>]{}* & 0.0241 & 0.0441 & 0.4125 & 0.4998 & & 0.5521 & 0.0743\
*[<span style="font-variant:small-caps;">stamp</span>]{}* & 0.0223 & 0.0420 & 0.3806 & 0.4620 & 0.2527 & 0.4865 & 0.0677\
[<span style="font-variant:small-caps;">ar</span>]{}& 0.0205 & 0.0387 & 0.3533 & 0.4367 & 0.2407 & 0.5444 & 0.0527\
[<span style="font-variant:small-caps;">sr</span>]{}& 0.0194 & 0.0362 & 0.3359 & 0.4174 & 0.2453 & 0.5185 &\
*[<span style="font-variant:small-caps;">nextitnet</span>]{}* & 0.0173 & 0.0320 & 0.3051 & 0.3779 & 0.2038 & 0.5737 & 0.0703\
[<span style="font-variant:small-caps;">ct</span>]{}& 0.0162 & 0.0308 & 0.2902 & 0.3632 & 0.2305 & 0.4026 & 0.3740\
\
[<span style="font-variant:small-caps;">sknn</span>]{}& [**0.0255**]{} & [**0.0596**]{} & 0.3715 & 0.4748 & 0.1714 & 0.8701 & 0.1026\
[<span style="font-variant:small-caps;">vstan</span>]{}& 0.0252 & 0.0588 & [**0.3723**]{} & [$*$**0.4803**]{} & [$*$**0.1837**]{} & 0.9384 & 0.0858\
[<span style="font-variant:small-caps;">stan</span>]{}& 0.0252 & 0.0589 & 0.3720 & 0.4800 & 0.1828 & 0.9161 & 0.0964\
[<span style="font-variant:small-caps;">v-sknn</span>]{}& 0.0249 & 0.0584 & 0.3668 & 0.4729 & 0.1784 & & 0.0840\
*[<span style="font-variant:small-caps;">gru4rec</span>]{}* & & & & & & [**0.9498**]{} & [**0.0567**]{}\
*[<span style="font-variant:small-caps;">csrm</span>]{}* & 0.0227 & 0.0544 & 0.3335 & 0.4258 & 0.1421 & 0.7337 & 0.0833\
*[<span style="font-variant:small-caps;">narm</span>]{}* & 0.0218 & 0.0528 & 0.3254 & 0.4188 & 0.1392 & 0.8696 & 0.0832\
*[<span style="font-variant:small-caps;">stamp</span>]{}* & 0.0201 & 0.0489 & 0.3040 & 0.3917 & 0.1314 & 0.9188 & 0.0799\
[<span style="font-variant:small-caps;">ar</span>]{}& 0.0189 & 0.0463 & 0.2872 & 0.3720 & 0.1280 & 0.8892 & 0.0863\
*[<span style="font-variant:small-caps;">sr</span><span style="font-variant:small-caps;">gnn</span>]{}* & 0.0186 & 0.0451 & 0.2840 & 0.3638 & 0.1564 & 0.8593 & 0.1092\
[<span style="font-variant:small-caps;">sr</span>]{}& 0.0161 & 0.0401 & 0.2489 & 0.3277 & 0.1216 & 0.8736 &\
*[<span style="font-variant:small-caps;">nextitnet</span>]{}* & 0.0149 & 0.0380 & 0.2416 & 0.2922 & 0.1424 & 0.7935 & 0.0947\
[<span style="font-variant:small-caps;">ct</span>]{}& 0.0115 & 0.0294 & 0.1860 & 0.2494 & 0.1075 & 0.7554 & 0.4262\
\
[<span style="font-variant:small-caps;">vstan</span>]{}& [**0.0168**]{} & [$*$**0.0777**]{} & [$*$**0.2073**]{} & [$*$**0.5362**]{} & 0.2488 & 0.5497 &\
[<span style="font-variant:small-caps;">stan</span>]{}& 0.0167 & 0.0774 & 0.2062 & 0.5328 & 0.2468 & 0.4918 & 0.0734\
[<span style="font-variant:small-caps;">v-sknn</span>]{}& 0.0158 & 0.0740 & 0.1956 & 0.5162 & 0.2487 & & 0.0680\
[<span style="font-variant:small-caps;">sknn</span>]{}& 0.0157 & 0.0738 & 0.1891 & 0.4352 & 0.1724 & 0.3316 & 0.0843\
*[<span style="font-variant:small-caps;">sr</span><span style="font-variant:small-caps;">gnn</span>]{}* & & & & 0.4755 & 0.2804 & 0.3845 & 0.0865\
*[<span style="font-variant:small-caps;">narm</span>]{}* & 0.0144 & 0.0692 & 0.1795 & 0.4598 & 0.2248 & 0.3695 & 0.0837\
*[<span style="font-variant:small-caps;">csrm</span>]{}* & 0.0143 & 0.0695 & 0.1764 & 0.4500 & 0.2347 & 0.2767 & 0.0789\
*[<span style="font-variant:small-caps;">gru4rec</span>]{}* & 0.0143 & 0.0666 & 0.1797 & & [**0.3069**]{} & [**0.6365**]{} & [$*$**0.0403**]{}\
[<span style="font-variant:small-caps;">sr</span>]{}& 0.0136 & 0.0638 & 0.1739 & 0.4824 & & 0.5849 & 0.0696\
[<span style="font-variant:small-caps;">ar</span>]{}& 0.0133 & 0.0631 & 0.1690 & 0.4665 & 0.2579 & 0.4672 & 0.0886\
[<span style="font-variant:small-caps;">ct</span>]{}& 0.0118 & 0.0564 & 0.1573 & 0.4561 & 0.2993 & 0.4653 & 0.2564\
*[<span style="font-variant:small-caps;">stamp</span>]{}* & 0.0104 & 0.0515 & 0.1359 & 0.3687 & 0.2065 & 0.2234 & 0.0868\
\
*[<span style="font-variant:small-caps;">narm</span>]{}* & [**0.0357**]{} & [**0.0735**]{} & [**0.5109**]{} & & 0.3047 & 0.6399 & 0.0638\
*[<span style="font-variant:small-caps;">sr</span><span style="font-variant:small-caps;">gnn</span>]{}* & 0.0351 & 0.0725 & 0.5060 & 0.6713 & [**0.3142**]{} & 0.5105 & 0.0720\
[<span style="font-variant:small-caps;">vstan</span>]{}& & & & [**0.6761**]{} & 0.2943 & 0.6762 &\
*[<span style="font-variant:small-caps;">csrm</span>]{}* & 0.0346 & 0.0714 & 0.4952 & 0.6566 & 0.2961 & 0.5929 & 0.0626\
*[<span style="font-variant:small-caps;">stamp</span>]{}* & 0.0344 & 0.0713 & 0.4979 & 0.6654 & 0.3033 & 0.5803 & 0.0655\
[<span style="font-variant:small-caps;">stan</span>]{}& 0.0342 & 0.0701 & 0.4986 & 0.6656 & 0.2933 & & 0.0773\
[<span style="font-variant:small-caps;">v-sknn</span>]{}& 0.0341 & 0.0707 & 0.4937 & 0.6512 & 0.2872 & 0.6333 & 0.0777\
*[<span style="font-variant:small-caps;">gru4rec</span>]{}* & 0.0334 & 0.0682 & 0.4837 & 0.6480 & 0.2826 & [**0.7482**]{} & [**0.0294**]{}\
[<span style="font-variant:small-caps;">sr</span>]{}& 0.0332 & 0.0684 & 0.4853 & 0.6506 & 0.3010 & 0.6674 & 0.0716\
[<span style="font-variant:small-caps;">ar</span>]{}& 0.0325 & 0.0673 & 0.4760 & 0.6361 & 0.2894 & 0.6297 & 0.0926\
[<span style="font-variant:small-caps;">sknn</span>]{}& 0.0318 & 0.0657 & 0.4658 & 0.5996 & 0.2620 & 0.6099 & 0.0796\
[<span style="font-variant:small-caps;">ct</span>]{}& 0.0316 & 0.0654 & 0.4710 & 0.6359 & & 0.6270 & 0.1446\
Results {#sec:results}
=======
In this section, we report the results of our offline evaluation. We will first focus on accuracy, then look at alternative quality measures, and finally discuss aspects of scalability and the stability of different models over time.
Accuracy Results
----------------
#### E-Commerce Datasets.
Table \[tab:results-ec\] shows the results for the e-commerce datasets. The highest value across all techniques is printed in bold; the highest value obtained by the other family of algorithms—neural or non-neural—is underlined. Stars indicate significant differences (p$<$0.05) according to a Kruskal–Wallis test between all the models and a Wilcoxon signed-rank test between the best-performing techniques from each category. The results for the individual datasets can be summarized as follows.
- On the [RETAIL]{}dataset, the nearest-neighbors methods consistently lead to the highest accuracy results on all the accuracy measures. Among the complex models, the best results were obtained by [<span style="font-variant:small-caps;">gru4rec</span>]{}on all the measures except for MRR, where [<span style="font-variant:small-caps;">sr</span><span style="font-variant:small-caps;">gnn</span>]{}led to the best value. The results for [<span style="font-variant:small-caps;">narm</span>]{}and [<span style="font-variant:small-caps;">gru4rec</span>]{}are almost identical on most measures.
- The results for the [DIGI]{}dataset are comparable, with the neighborhood methods leading to the best accuracy results. [<span style="font-variant:small-caps;">gru4rec</span>]{}is again the best method across the complex models on all the measures.
- For the [ZALANDO]{}dataset, the neighborhood methods dominate all accuracy measures, except for the MRR. Here, [<span style="font-variant:small-caps;">gru4rec</span>]{}is minimally better than the simple [<span style="font-variant:small-caps;">sr</span>]{}method. Among the complex models, [<span style="font-variant:small-caps;">gru4rec</span>]{}achieves the best HR value, and the recent [<span style="font-variant:small-caps;">sr</span><span style="font-variant:small-caps;">gnn</span>]{}method is the best one on the other accuracy measures.
- Only for the [RSC15]{}dataset, we can observe that a neural method ([<span style="font-variant:small-caps;">narm</span>]{}) is able to slightly outperform our best simple baseline [<span style="font-variant:small-caps;">vstan</span>]{}in terms of MAP, Precision and Recall. Interestingly, however, [<span style="font-variant:small-caps;">narm</span>]{}is one of the earlier neural methods in this comparison. The best Hit Rate is achieved by [<span style="font-variant:small-caps;">vstan</span>]{}; the best MRR by [<span style="font-variant:small-caps;">sr</span><span style="font-variant:small-caps;">gnn</span>]{}. The differences between the best neural and non-neural methods are often tiny, in most cases around or less than 1%.
Looking at the results across the different datasets, we can make the following additional observations.
- Across all e-commerce datasets, the [<span style="font-variant:small-caps;">vstan</span>]{}method proposed in this paper is, for most measures, the best neighborhood-based method. This suggests that it is reasonable to include it as a baseline in future performance comparisons.
- The ranking of the *neural* methods varies largely across the datasets and does not follow the order in which the methods were proposed. Like for the non-neural methods, the specific ranking therefore seems to be strongly depending on the dataset characteristics. This makes it particularly difficult to judge the progress that is made when only one or two datasets are used for the evaluation.
- The results for the [RSC15]{}dataset are generally different from the other results. Specifically, we found that some neural methods are competitive and slightly outperform our baselines. [<span style="font-variant:small-caps;">stamp</span>]{}is not among the top performers except for this dataset. Unlike for other e-commerce datasets, [<span style="font-variant:small-caps;">ct</span>]{}works particularly well for this dataset in terms of the MRR. Given these observations, it seems that the [RSC15]{}dataset has some unique characteristics that are different from the other e-commerce datasets. Therefore, it seems advisable to consider multiple datasets with different characteristics in future evaluations.
- We did not include measurements for [<span style="font-variant:small-caps;">nextitnet</span>]{}, one of the most recent methods, for the larger [ZALANDO]{}and [RSC15]{}datasets. We found that this method does not scale well and we could not complete the hyper-parameter tuning process within weeks on our machines (also for two music datasets).
[1]{}[@Xrrr|rr|rr@]{} Metrics & MAP@20 & P@20 & R@20 & HR@20 & MRR@20 & COV@20 & POP@20\
\
& [$*$**0.0193**]{} & [**0.0664**]{} & [$*$**0.1828**]{} & 0.2534 & 0.0810 & 0.4661 & 0.0582\
[<span style="font-variant:small-caps;">sknn</span>]{}& 0.0186 & 0.0655 & 0.1809 & 0.2450 & 0.0687 & 0.3150 & 0.0619\
[<span style="font-variant:small-caps;">stan</span>]{}& 0.0175 & 0.0585 & 0.1696 & 0.2414 & 0.0871 & & 0.0473\
[<span style="font-variant:small-caps;">vstan</span>]{}& 0.0174 & 0.0609 & 0.1795 & [$*$**0.2597**]{} & 0.0853 & 0.4299 & 0.0505\
[<span style="font-variant:small-caps;">ar</span>]{}& 0.0166 & 0.0564 & 0.1544 & 0.2076 & 0.0710 & 0.4531 & 0.0511\
[<span style="font-variant:small-caps;">sr</span>]{}& 0.0133 & 0.0466 & 0.1366 & 0.2002 & 0.1052 & 0.4661 &\
*[<span style="font-variant:small-caps;">sr</span><span style="font-variant:small-caps;">gnn</span>]{}* & & & & 0.2113 & 0.0935 & 0.3265 & 0.0576\
*[<span style="font-variant:small-caps;">narm</span>]{}* & 0.0118 & 0.0463 & 0.1274 & 0.1849 & 0.0894 & 0.4715 & 0.0488\
*[<span style="font-variant:small-caps;">gru4rec</span>]{}* & 0.0116 & 0.0449 & 0.1361 & & & [$*$**0.5795**]{} & [**0.0286**]{}\
*[<span style="font-variant:small-caps;">stamp</span>]{}* & 0.0111 & 0.0456 & 0.1244 & 0.1954 & 0.0921 & 0.2148 & 0.0714\
*[<span style="font-variant:small-caps;">csrm</span>]{}* & 0.0095 & 0.0388 & 0.1065 & 0.1508 & 0.0594 & 0.2445 & 0.0494\
[<span style="font-variant:small-caps;">ct</span>]{}& 0.0065 & 0.0287 & 0.0893 & 0.1679 & [**0.1094**]{} & 0.2714 & 0.2984\
\
& [$*$**0.0309**]{} & [$*$**0.1090**]{} & [$*$**0.2347**]{} & 0.3830 & 0.1162 & 0.3667 & 0.0485\
[<span style="font-variant:small-caps;">vstan</span>]{}& 0.0296 & 0.1003 & 0.2306 & [$*$**0.3904**]{} & 0.1564 & &\
[<span style="font-variant:small-caps;">sknn</span>]{}& 0.0290 & 0.1073 & 0.2217 & 0.3443 & 0.0898 & 0.1913 & 0.0574\
[<span style="font-variant:small-caps;">stan</span>]{}& 0.0278 & 0.0949 & 0.2227 & 0.3830 & 0.1533 & 0.4315 & 0.0347\
[<span style="font-variant:small-caps;">ar</span>]{}& 0.0254 & 0.0886 & 0.1930 & 0.3088 & 0.0960 & 0.3524 & 0.0393\
[<span style="font-variant:small-caps;">sr</span>]{}& 0.0240 & 0.0816 & 0.1937 & 0.3327 & 0.2410 & 0.4131 & 0.0317\
*[<span style="font-variant:small-caps;">narm</span>]{}* & & & 0.1486 & 0.2956 & 0.1945 & 0.3858 & 0.0425\
*[<span style="font-variant:small-caps;">gru4rec</span>]{}* & 0.0150 & 0.0617 & & & & [**0.4881**]{} & [**0.0255**]{}\
*[<span style="font-variant:small-caps;">csrm</span>]{}* & 0.0118 & 0.0536 & 0.1236 & 0.2652 & 0.1503 & 0.2290 & 0.0390\
*[<span style="font-variant:small-caps;">sr</span><span style="font-variant:small-caps;">gnn</span>]{}* & 0.0108 & 0.0482 & 0.1151 & 0.2883 & 0.1894 & 0.3965 & 0.0412\
*[<span style="font-variant:small-caps;">stamp</span>]{}* & 0.0093 & 0.0411 & 0.0875 & 0.1539 & 0.0819 & 0.0852 & 0.0491\
[<span style="font-variant:small-caps;">ct</span>]{}& 0.0058 & 0.0308 & 0.0885 & 0.2882 & [$*$**0.2502**]{} & 0.1932 & 0.4255\
\
[<span style="font-variant:small-caps;">sknn</span>]{}& [$*$**0.0037**]{} & [$*$**0.0139**]{} & [$*$**0.0390**]{} & [$*$**0.0417**]{} & 0.0054 & 0.2937 & 0.1467\
& 0.0032 & 0.0116 & 0.0312 & 0.0352 & 0.0057 & 0.5886 & 0.1199\
[<span style="font-variant:small-caps;">stan</span>]{}& 0.0031 & 0.0126 & 0.0357 & 0.0402 & 0.0054 & 0.2979 & 0.1667\
[<span style="font-variant:small-caps;">vstan</span>]{}& 0.0024 & 0.0083 & 0.0231 & 0.0271 & 0.0060 & [$*$**0.6907**]{} & [**0.0566**]{}\
[<span style="font-variant:small-caps;">ar</span>]{}& 0.0018 & 0.0076 & 0.0200 & 0.0233 & 0.0059 & 0.5532 & 0.1049\
[<span style="font-variant:small-caps;">sr</span>]{}& 0.0010 & 0.0047 & 0.0134 & 0.0186 & 0.0074 & 0.5669 & 0.0711\
*[<span style="font-variant:small-caps;">narm</span>]{}* & & & & & & 0.4816 & 0.1119\
[<span style="font-variant:small-caps;">ct</span>]{}& 0.0006 & 0.0043 & 0.0126 & 0.0191 & [$*$**0.0111**]{} & 0.3357 & 0.4680\
*[<span style="font-variant:small-caps;">sr</span><span style="font-variant:small-caps;">gnn</span>]{}* & 0.0006 & 0.0032 & 0.0096 & 0.0148 & 0.0082 & 0.4283 & 0.0812\
*[<span style="font-variant:small-caps;">csrm</span>]{}* & 0.0005 & 0.0040 & 0.0109 & 0.0100 & 0.0021 & 0.0056 & 0.6478\
*[<span style="font-variant:small-caps;">nextitnet</span>]{}* & 0.0004 & 0.0024 & 0.0071 & 0.0139 & 0.0065 & 0.4851 & 0.0960\
*[<span style="font-variant:small-caps;">stamp</span>]{}* & 0.0003 & 0.0020 & 0.0063 & 0.0128 & & 0.5168 & 0.0872\
*[<span style="font-variant:small-caps;">gru4rec</span>]{}* & 0.0003 & 0.0020 & 0.0063 & 0.0130 & 0.0074 & &\
\
[<span style="font-variant:small-caps;">sknn</span>]{}& [$*$**0.0024**]{} & & [$*$**0.0343**]{} & [$*$**0.0377**]{} & 0.0054 & 0.2352 & 0.1622\
[<span style="font-variant:small-caps;">stan</span>]{}& 0.0022 & 0.0119 & 0.0313 & 0.0357 & 0.0052 & 0.2971 & 0.1382\
& 0.0021 & 0.0110 & 0.0276 & 0.0312 & 0.0056 & 0.4572 & 0.1064\
[<span style="font-variant:small-caps;">vstan</span>]{}& 0.0018 & 0.0086 & 0.0227 & 0.0265 & 0.0056 & [$*$**0.5192**]{} & 0.0757\
*[<span style="font-variant:small-caps;">narm</span>]{}* & & [**0.0131**]{} & & & [$*$**0.0083**]{} & 0.0788 & 0.1589\
*[<span style="font-variant:small-caps;">sr</span><span style="font-variant:small-caps;">gnn</span>]{}* & 0.0017 & 0.0123 & 0.0301 & 0.0330 & 0.0077 & 0.0211 & 0.1833\
[<span style="font-variant:small-caps;">ar</span>]{}& 0.0016 & 0.0088 & 0.0219 & 0.0255 & & 0.4529 & 0.0912\
*[<span style="font-variant:small-caps;">stamp</span>]{}* & 0.0015 & 0.0114 & 0.0256 & 0.0272 & 0.0061 & 0.0405 & 0.1374\
[<span style="font-variant:small-caps;">sr</span>]{}& 0.0012 & 0.0067 & 0.0166 & 0.0201 & & 0.4897 & [$*$**0.0657**]{}\
*[<span style="font-variant:small-caps;">csrm</span>]{}* & 0.0011 & 0.0087 & 0.0189 & 0.0204 & 0.0048 & 0.0417 & 0.1587\
*[<span style="font-variant:small-caps;">gru4rec</span>]{}* & 0.0007 & 0.0060 & 0.0132 & 0.0161 & 0.0051 & &\
[<span style="font-variant:small-caps;">ct</span>]{}& 0.0007 & 0.0054 & 0.0127 & 0.0170 & & 0.2732 & 0.2685\
#### Music Domain
In Table \[tab:results-music\] we present the results for the music datasets. In general, the observations are in line with what we observed for the e-commerce domain regarding the competitiveness of the simple methods.
- Across all datasets excluding the [8TRACKS]{}dataset, the nearest-neighbors methods are consistently favorable in terms of Precision, Recall, MAP and the Hit Rate, and the [<span style="font-variant:small-caps;">ct</span>]{}method leads to the best MRR. Moreover, the simple [<span style="font-variant:small-caps;">sr</span>]{}technique often leads to very good MRR values.
- For [8TRACKS]{}dataset, the best Recall, MAP and the Hit Rate values are again achieved by neighborhood methods. The best Precision and the MRR values are, however, achieved by a neural method ([<span style="font-variant:small-caps;">narm</span>]{}).
- Again, no consistent ranking of the algorithms can be found across the datasets. In particular the neural approaches take largely varying positions in the rankings across the datasets. Generally, [<span style="font-variant:small-caps;">narm</span>]{}seems to be a technique which performs consistently well on most datasets and measures.
Coverage and Popularity
-----------------------
Table \[tab:results-ec\] and Table \[tab:results-music\] also contain information about the popularity bias of the individual algorithms and coverage information. Remember that we described in Section \[subsec:evaluation-procedure\] how the numbers were calculated. From the results, we can identify the following trends regarding individual algorithms and the different algorithm families.
#### Popularity Bias.
- The [<span style="font-variant:small-caps;">ct</span>]{}method is very different from all other methods in terms of its *popularity bias*, which is much higher than for any other method.
- The [<span style="font-variant:small-caps;">gru4rec</span>]{}method, on the other hand, is the method that almost consistently recommends the most unpopular (or: novel) items to the users.
- The neighborhood-based methods are often somewhere in the middle. There are, however, also neural methods, in particular [<span style="font-variant:small-caps;">sr</span><span style="font-variant:small-caps;">gnn</span>]{}, which seem to have a similar or sometimes even stronger popularity bias than the nearest-neighbors approaches. The assumption that nearest-neighbors methods are in general more focusing on popular items than neural methods can therefore not be confirmed through our experiments.
#### Coverage.
- In terms of *coverage*, we found that [<span style="font-variant:small-caps;">gru4rec</span>]{}often leads to the highest values.
- The coverage of the neighborhood-based methods varies quite a lot, depending on the specific algorithm variant. In some configurations, their coverage is almost as high as for [<span style="font-variant:small-caps;">gru4rec</span>]{}, while in others the coverage can be low.
- The coverage values of the other neural methods also do not show a clear ranking, and they are often in the range of the neighborhood-based methods and sometimes even very low.
Scalability
-----------
We present selected results regarding the running times of the algorithms for two e-commerce datasets and one music dataset in Table \[tab:running-times\]. The reported times were measured for training and predicting for one data split. The numbers reported for predicting correspond to the average time needed to generate a recommendation for a session beginning in the test set. For this measurement, we used a workstation computer with an Intel Core i7-4790k processor and an Nvidia Geforce GTX 1080 Ti graphics card (Cuda 10.1/CuDNN 7.5).
[1]{}[@Xrrrrrr@]{} & &\
Algorithm & [RSC15]{}& [ZALANDO]{}& [8TRACKS]{}& [RSC15]{}& [ZALANDO]{}& [8TRACKS]{}\
[<span style="font-variant:small-caps;">gru4rec2</span>]{}& 0.72h & 0.66h & 0.21h & 7.72 & 25.97 & 278.23\
[<span style="font-variant:small-caps;">stamp</span>]{}& 0.54h & 2.22h & 1.87h & 14.94 & 55.45 & 423.94\
[<span style="font-variant:small-caps;">narm</span>]{}& 3.76h & 13.30h & 10.40h & 7.83 & 25.00 & 211.35\
[<span style="font-variant:small-caps;">sr</span><span style="font-variant:small-caps;">gnn</span>]{}& 13.79h & 25.45h & 8.04h & 27.67 & 120.15 & 797.97\
[<span style="font-variant:small-caps;">csrm</span>]{}& 2.61h & 3.39h & 1.61h & 24.98 & 66.93 & 250.23\
[<span style="font-variant:small-caps;">nextitnet</span>]{}& 26.29h & – & – & 8.98 & – & –\
[<span style="font-variant:small-caps;">ar</span>]{}& 23.70s & 60.04s & 20.41s & 4.66 & 12.00 & 105.43\
[<span style="font-variant:small-caps;">sr</span>]{}& 24.74s & 31.82s & 15.14s & 4.66 & 11.77 & 101.98\
[<span style="font-variant:small-caps;">sknn</span>]{}& 10.81s & 7.52s & 3.29s & 37.82 & 27.77 & 291.26\
[<span style="font-variant:small-caps;">v-sknn</span>]{}& 11.24s & 8.03s & 3.26s & 18.75 & 30.56 & 278.51\
[<span style="font-variant:small-caps;">stan</span>]{}& 10.57s & 11.76s & 3.16s & 36.78 & 33.26 & 317.23\
[<span style="font-variant:small-caps;">vstan</span>]{}& 10.80s & 7.75s & 3.46s & 21.33 & 55.58 & 288.40\
[<span style="font-variant:small-caps;">ct</span>]{}& 0.18h & 0.26h & 0.07h & 73.34 & 484.87 & 1452.71\
The results generally show that the computational complexity of neural methods is, as expected, much higher than for the non-neural approaches. In some cases, researchers therefore only use a smaller fraction of the original datasets, e.g., or of the [RSC15]{}dataset. Several algorithms—both neural ones and the [<span style="font-variant:small-caps;">ct</span>]{}method—exhibit major scalability issues when the number of recommendable items increases. For the [<span style="font-variant:small-caps;">nextitnet</span>]{}method, for example, training on the [ZALANDO]{}dataset with its almost 190k items and its particularly long sessions did not complete within a reasonable time frame in our experiments.
In some cases, like for [<span style="font-variant:small-caps;">ct</span>]{}or [<span style="font-variant:small-caps;">sr</span><span style="font-variant:small-caps;">gnn</span>]{}, not only the training time increases, but also the prediction times. In particular the prediction times can, however, be subject to strict time constraints in production settings. The prediction times for the nearest-neighbors methods are often slightly higher than those measured for methods like [<span style="font-variant:small-caps;">gru4rec</span>]{}, but usually lie within the time constraints of real-time recommendation (e.g., requiring about 30ms for one prediction for the [ZALANDO]{}dataset).
Since datasets in real-world environments can be even larger, this leaves us with questions regarding the practicability of some of the approaches. In general, even in case where a complex neural method would slightly outperform one of the more simple ones in an offline evaluation, it remains open if it is worth the effort to put such complex methods into production. For the [ZALANDO]{}dataset, for example, the best neural method ([<span style="font-variant:small-caps;">sr</span><span style="font-variant:small-caps;">gnn</span>]{}) needs several orders of magnitude[^7] more time to train than the best non-neural method [<span style="font-variant:small-caps;">vstan</span>]{}, which also only needs half the time for recommending.
Stability With Respect to New Data
----------------------------------
We report the stability results for the examined neural and non-neural algorithms on two datasets in Table \[tab:stability\_completed\]. We used two months of training data and 10 days of test data for both datasets, [DIGI]{}and [NOWP]{}. The reported values show how much the accuracy results of each algorithm degrades (in percent), averaged across the test days when there is no daily retraining.
[1]{}[@Xrrrr@]{} & &\
Metrics & HR@20 & MRR@20 & HR@20 & MRR@20\
[<span style="font-variant:small-caps;">sknn</span>]{}& & & & **-14.29%**\
[<span style="font-variant:small-caps;">v-sknn</span>]{}& -2.28% & -0.64% & -27.20% & -14.36%\
[<span style="font-variant:small-caps;">vstan</span>]{}& -2.53% & -0.64% & -28.53% & -28.22%\
[<span style="font-variant:small-caps;">stan</span>]{}& -2.97% & -0.29% & -27.21% & -27.92%\
[<span style="font-variant:small-caps;">ar</span>]{}& -4.83% & -5.33% & -29.76% & -33.94%\
[<span style="font-variant:small-caps;">sr</span>]{}& -6.22% & -6.14% & -32.38% & -70.05%\
[<span style="font-variant:small-caps;">ct</span>]{}& -7.98% & -6.94% & -50.49% & -85.97%\
[<span style="font-variant:small-caps;">narm</span>]{}& **-1.84%** & **0.30%** & -35.10% & -70.28%\
[<span style="font-variant:small-caps;">gru4rec</span>]{}& -2.79% & -1.84% & -46.03% & -74.11%\
[<span style="font-variant:small-caps;">nextitnet</span>]{}& -3.75% & -4.69% & - & -\
[<span style="font-variant:small-caps;">sr</span><span style="font-variant:small-caps;">gnn</span>]{}& -3.76% & -2.14% & -46.05% & -75.74%\
[<span style="font-variant:small-caps;">csrm</span>]{}& -4.20% & -4.68% & **-17.84%** &\
[<span style="font-variant:small-caps;">stamp</span>]{}& -7.80% & -7.28% & -46.48% & -45.78%\
We can see from the results that the drop in accuracy without retraining can vary a lot across datasets (domains). For the [DIGI]{}dataset, the decrease in performance ranges between 0 and 10 percent across the different algorithms and performance measures. The [NOWP]{}dataset from the music domain seems to be more short-lived, with more recent trends that have to be considered. Here, the decrease in performance ranges from about 15 to 50 percent in terms of HR and from about 15 to 85 percent in terms of MRR.[^8]
Looking at the detailed results, we see that in both families of algorithms, i.e., neural and non-neural ones, some algorithms are much more stable than others when new data are added to a given dataset. For the family of non-neural approaches, we see that nearest-neighbor approaches are generally better than the other baselines techniques based on association rules or context trees.
Among the neural methods, [<span style="font-variant:small-caps;">narm</span>]{}is the most stable one on the [DIGI]{}dataset, but often falls behind the other deep learning methods on the [NOWP]{}dataset.[^9] On this latter dataset, the [<span style="font-variant:small-caps;">csrm</span>]{}method leads to the most stable results. In general, however, no clear pattern across the datasets can be found regarding the performance of the neural methods when new data comes in and no retraining is done.
Overall, given that the computational costs of training complex models can be high, it can be advisable to look at the stability of algorithms with respect to new data when choosing a method for production. According to our analysis, there can be strong differences across the algorithms. Furthermore, the nearest-neighbors methods appear to be quite stable in this comparison.
Observations From a User Study {#sec:user-study}
==============================
Offline evaluations, while predominant in the literature, can have certain limitations, in particular when it comes to the question how the quality of the provided recommendations is *perceived* by users. We therefore conducted a controlled experiment, in which we compared different algorithmic approaches for session-based recommendation in the context of an online radio station. In the following sections, we report the main insights of this experiment. While the study did not include all algorithms from our offline analysis, we consider it helpful to obtain a more comprehensive picture regarding performance of session-based recommenders. More details about the study cam be found in [@ludewigjannach2019radio].
Research Questions and Study Setup
----------------------------------
#### Research Questions.
Our offline analysis indicated that simple methods are often competitive than the more complex ones. Our main research question therefore was how the recommendations generated by such simple methods are perceived by its users in different dimensions, in particular compared to recommendations by a complex method. Furthermore, we were interested how users perceive the recommendations of a commercial music streaming service, in our case <span style="font-variant:small-caps;">Spotify</span>, in the same situation.
#### Study Setup.
An online music listening application in the form of an “automated radio station” was developed for the purpose of the study. Similar to existing commercial services, users of the application could select a track they like (called a “seed track”), based on which the application creates a playlist of subsequent tracks that are played automatically. While the music was played, the users could listen it to the end before moving to the next track, skip the track if they did not like the it, or press a “like” button. In case of a *like* action, the list of upcoming tracks was updated. Users were visually hinted that such an update takes place.
Besides recording skips and like actions, additional feedback was collected from the study participants. Before going to the next track, they had to answer for each listened track (i) if they already knew the track, (ii) to what extent the track matched the previously played track, and (iii) to what extent they liked the track (independent of the playlist), see Figure \[fig:radio2\].
![Track Rating Interface of the Application[]{data-label="fig:radio2"}](radio2.pdf){width="88.00000%"}
Once the participants had listened to and rated at least 15 tracks, they were forwarded to a post-task questionnaire. In this questionnaire, we asked the participants 11 questions about how they perceived the service, see also [@Pu:2011:UEF:2043932.2043962]. Specifically, the participants were asked to provide answers to the questions using seven-point Likert scale items, ranging from “completely disagree” to “completely agree”. The questions, which include a twelfth question as an attention check, are listed in Table \[tab:quality-questions\].
\[h!t\]
[1]{}[@lX@]{}\
Q1 & I liked the automatically generated radio station.\
Q2 & The radio suited my general taste in music.\
Q3 & The tracks on the radio musically matched the track I selected in the beginning.\
Q4 & The radio was tailored to my preferences the more positive feedback I gave.\
Q5 & The radio was diversified in a good way.\
Q6 & The tracks on the radio surprised me.\
Q7 & I discovered some unknown tracks that I liked in the process.\
Q8 & I am participating in this study with care so I change this slider to two.\
Q9 & I would listen to the same radio station based on that track again.\
Q10 & I would use this system again, e.g., with a different first song.\
Q11 & I would recommend this radio station to a friend.\
Q12 & I would recommend this system to a friend.\
The study itself was based on a between-subjects design, where the treatments for each user group correspond to different algorithmic approaches to generate the recommendations. We included algorithms from different families in our study.
- [<span style="font-variant:small-caps;">ar</span>]{}: Association rules of length two, as described in Section \[sec:algorithms\]. We included this method as a simple baseline.
- [<span style="font-variant:small-caps;">cagh</span>]{}: Another relatively simple baseline, which recommends the greatest hits of artists similar to those liked in the current session. This music-specific method is often competitive in offline evaluations as well, see [@Bonnin:2014:AGM:2658850.2652481].
- [<span style="font-variant:small-caps;">sknn</span>]{}: The basic nearest-neighbors method described above. We took the simple variant as a representative for the family of such approaches, as it performed particularly well in the ACM RecSys 2018 challenge [@Ludewig2018rsc].
- [<span style="font-variant:small-caps;">gru4rec</span>]{}: The RNN-based approach discussed above, used as a representative for neural methods. [<span style="font-variant:small-caps;">narm</span>]{}would have been a stable alternative, but did not scale well for the used dataset.
- [<span style="font-variant:small-caps;">spotify</span>]{}: Recommendations in this treatment group were retrieved in real time from Spotify’s API.
We optimized and trained all models on the Million Playlist Dataset Million Playlist Dataset (MPD) [^10] provided by Spotify. We then recruited study participants using Amazon’s Mechanical Turk crowdsourcing platform. After excluding participants who did not pass the attention checks, we ended up with *N=250* participants, i.e., 50 for each treatment group, for which we were confident that they provided reliable feedback.
Most of the recruited participants (almost 80%) were US-based. The most typical age range was between 25 and 34, with more than 50% of the participants falling into this category. On average, the participants considered themselves to be music enthusiasts, with an average response of 5.75 (on the seven-point scale) to a corresponding survey question. As usual, the participants received a compensation for their efforts through the crowdsourcing platform.
User Study Outcomes
-------------------
The main observations can be summarized as follows.
#### Feedback the Listening Experience.
Looking at the feedback that was observed during the listening session, we observed the following.
- *Number of Likes.* There were significant differences regarding the number of *likes* we observed across the treatment groups. Recommendations by the simple [<span style="font-variant:small-caps;">ar</span>]{}method received the highest number of likes (6.48), followed by [<span style="font-variant:small-caps;">sknn</span>]{}(5.63), [<span style="font-variant:small-caps;">cagh</span>]{}(5.38), [<span style="font-variant:small-caps;">gru4rec</span>]{}(5.36) and [<span style="font-variant:small-caps;">spotify</span>]{}(4.48).
- *Popularity of Tracks.* We found a clear correlation (*r*=0.89) between the general popularity of a track in the MPD dataset and the number of likes in the study. The [<span style="font-variant:small-caps;">ar</span>]{}and [<span style="font-variant:small-caps;">cagh</span>]{}methods recommended, on average, the most popular tracks. The recommendations by [<span style="font-variant:small-caps;">spotify</span>]{}and [<span style="font-variant:small-caps;">gru4rec</span>]{}were more oriented towards tracks with lower popularity.
- *Track Familiarity.* There were also clear differences in terms of how many of the recommended tracks were already known by the users. The [<span style="font-variant:small-caps;">cagh</span>]{}(10.83%) and [<span style="font-variant:small-caps;">sknn</span>]{}(10.13%) methods recommended the largest number of known tracks. The [<span style="font-variant:small-caps;">ar</span>]{}method, even though it recommended very popular tracks, led to much more unfamiliar recommendations (8.61%). [<span style="font-variant:small-caps;">gru4rec</span>]{}was somewhere in the middle (9.30%), and [<span style="font-variant:small-caps;">spotify</span>]{}recommended the most novel tracks to users (7.00%).
- *Suitability of Track Continuations.* The continuations created by [<span style="font-variant:small-caps;">sknn</span>]{}and [<span style="font-variant:small-caps;">cagh</span>]{}were perceived to be the most suitable ones. The differences between [<span style="font-variant:small-caps;">sknn</span>]{}and [<span style="font-variant:small-caps;">ar</span>]{}, [<span style="font-variant:small-caps;">gru4rec</span>]{}, and [<span style="font-variant:small-caps;">spotify</span>]{}were significant. The recommendations made by the [<span style="font-variant:small-caps;">ar</span>]{}method were considered to match the playlist the least. This is not too surprising because the [<span style="font-variant:small-caps;">ar</span>]{}method only considers the very last played track for the recommendation of subsequent tracks.
- *Individual Track Ratings.* The differences regarding the individual ratings for each track ratings are generally small and not significant. Interestingly, the playlist-independent ratings for tracks recommended by the [<span style="font-variant:small-caps;">ar</span>]{}method were the lowest ones, even though these recommendations received the highest number of likes. An analysis of the rating distribution shows that the [<span style="font-variant:small-caps;">ar</span>]{}method often produces very bad recommendations, with a *mode* value of 1 on the 1-7 rating scale.
#### Post-Task Questionnaire
The post-task questionnaire revealed the following aspects:
- Q1: The radio station based on [<span style="font-variant:small-caps;">sknn</span>]{}was significantly more liked than the stations that used [<span style="font-variant:small-caps;">gru4rec</span>]{}, [<span style="font-variant:small-caps;">ar</span>]{}, and [<span style="font-variant:small-caps;">spotify</span>]{}.
- Q2: All radio stations matched the users general taste quite well, with median values between 5 and 6 on a seven-point scale. Only the station based on the [<span style="font-variant:small-caps;">ar</span>]{}method received a significantly lower rating than the others.
- Q3: The [<span style="font-variant:small-caps;">sknn</span>]{}method was found to perform significantly better than [<span style="font-variant:small-caps;">ar</span>]{}and [<span style="font-variant:small-caps;">gru4rec</span>]{}with respect to identifying tracks that musically match the seed track.
- Q4: The adaptation of the playlist based on the like statements was considered good for all radio stations. Again, the feedback for the [<span style="font-variant:small-caps;">ar</span>]{}method was significantly lower than for the other methods.
- Q5 and Q6: No significant differences were found regarding the surprise level of the different recommendation strategies.
- Q7: Regarding the capability of recommending unknown tracks that the users liked, the recommendations by [<span style="font-variant:small-caps;">spotify</span>]{}were perceived to be much better than for the other methods, with significant differences compared to all other methods.
- Q9 to Q12: The best performing methods in terms of the intention to reuse and the intention to recommend the radio station to others were [<span style="font-variant:small-caps;">sknn</span>]{}, [<span style="font-variant:small-caps;">cagh</span>]{}, and [<span style="font-variant:small-caps;">spotify</span>]{}. [<span style="font-variant:small-caps;">gru4rec</span>]{}and [<span style="font-variant:small-caps;">ar</span>]{}were slightly worse, sometimes with differences that were statistically significant.
Overall, the study confirmed that methods like [<span style="font-variant:small-caps;">sknn</span>]{}do not only perform well in an offline evaluation, but are also able, according to our study, to generate recommendations that are well perceived in different dimensions by the users. The study also revealed a number of additional insights. First, we found that optimizing for *like* statements can be misleading. The [<span style="font-variant:small-caps;">ar</span>]{}method received the highest number of likes, but was consistently worse than other techniques in almost all other dimensions. Apparently, this was caused by the fact that the [<span style="font-variant:small-caps;">ar</span>]{}method made a number of bad recommendations; see also [@CHAU2013180] for an analysis of the effects on bad recommendations in the music domain. Second, it turned out that *discovery support* seems to be an important factor in this particular application domain. While the recommendations of [<span style="font-variant:small-caps;">spotify</span>]{}were slightly less appreciated than those by [<span style="font-variant:small-caps;">sknn</span>]{}, we found no difference in terms of the user’s intention to reuse the system or to recommend it to friends. We hypothesize that the better discovery support of [<span style="font-variant:small-caps;">spotify</span>]{}’s recommendations was an important factor for this phenomenon. This observation points to the importance of considering multiple potential quality factors when comparing systems.
Conclusions and Ways Forward {#sec:discussion}
============================
Our work reveals that despite a continuous stream of papers that propose new neural approaches for session-based recommendation, the progress in the field seems still limited. According to our evaluations, today’s deep learning techniques are in many cases not outperforming much simpler heuristic methods. Overall, this indicates that there still is a huge potential for more effective neural recommendation methods in the future in this area. In particular, methods that leverage deep learning techniques to incorporate side information represent a promising way forward, see [@moreira2019contextual; @deSouzaPereiraMoreira2018; @Huang2018; @Hidasi:2016:PRN:2959100.2959167].
In a related analysis of deep learning techniques for recommender systems [@Ferraridacremaetal2019], the authors found that different factors contribute to what they call *phantom progress*. One first problem is related to the reproducibility of the reported results. They found that in less than a third of the investigated papers, the code was made available to other researchers. The problem also exists to some extent for session-based recommendation approaches. To further increase the level of reproducibility, we share our evaluation framework publicly, so that other researchers can easily benchmark their own methods with a comprehensive set of neural and non-neural approaches on different datasets.
Through sharing our evaluation framework, we hope to also address other methodological and procedural issues mentioned in [@Ferraridacremaetal2019] that can make the comparison of algorithms unreliable or inconclusive. Regarding methodological issues, we for example found works that determined the optimal number of training epochs on the test set and furthermore determined the best Hit Rate and MRR values across different optimization epochs. Regarding procedural issues, we found that while researchers seemingly rely on the same datasets as previous works, they sometimes apply different data pre-processing strategies. Furthermore, the choice of the baselines can make the results inconclusive. Most investigated works do not consider the [<span style="font-variant:small-caps;">sknn</span>]{}method and its variants as a baseline. Some works only compare variants of one method and include a non-neural, but not necessarily strong other baseline. In many cases, little is also said about the optimization of the hyper-parameters of the baselines. The <span style="font-variant:small-caps;"></span> framework used in our evaluation should help to avoid these problems, as it contains all the code for data pre-processing, evaluation, and hyper-parameter optimization.
Finally, our analyses indicated that optimizing solely for accuracy can be insufficient also for session-based recommendation scenarios. Depending on the application domain, other quality factors such as coverage, diversity, or novelty should be considered, because they can be crucial for the adoption and success of the recommendation service. Given the insights from our controlled experiment, we furthermore argue that more user studies and field tests are necessary to understand the characteristics of successful recommendations in a given application domain.
Acknowledgement {#acknowledgement .unnumbered}
===============
We thank Liliana Ardissono for her valuable feedback on the paper.
[^1]: This work combines and significantly extends our own previous work published in [@ludewigjannach2019radio] and [@LudewigMauro2019]. This paper or a similar version is not currently under review by a journal or conference. This paper is void of plagiarism or self-plagiarism as defined by the Committee on Publication Ethics and Springer Guidelines. We plan to publish a pre-print version of this work, compliant to the rules of the journal.
[^2]: Compared to our preliminary work presented in [@LudewigMauro2019], our present analysis includes considerably more recent deep learning techniques and baseline approaches. We also provide the outcomes of additional measurements regarding the scalability and stability of different algorithms. Finally, we also contrast the outcomes of the offline experiments with the findings obtained in a user study [@ludewigjannach2019radio].
[^3]: <https://github.com/rn5l/session-rec>
[^4]: The number of days used for testing ($n$) was determined based on the characteristics of the dataset. We, for example, used the last day for the [RSC15]{}dataset, two for [RETAIL]{}, five for the music datasets, and seven for [DIGI]{}to ensure that train-test splits are comparable.
[^5]: <https://rn5l.github.io/session-rec/umuai>
[^6]: We also optimized the hyper-parameters on a subset of $T_0$ that was used as a validation set. The hyper-parameters were kept constant for the remaining measurements.
[^7]: The training time for [<span style="font-variant:small-caps;">sr</span><span style="font-variant:small-caps;">gnn</span>]{}is 10.000 times higher than for [<span style="font-variant:small-caps;">vstan</span>]{}.
[^8]: Generally, comparing the numbers across the datasets is not meaningful due to their different characteristics.
[^9]: The experiments for [<span style="font-variant:small-caps;">nextitnet</span>]{}could not be completed on this dataset because the method’s resource requirements exceeded our computing capacities.
[^10]: <https://recsys-challenge.spotify.com/>
| 50,334,161 |
"\nStudents Are Better Off Without a Laptop in the Classroom - thearn4\nhttps://www.scientificameric(...TRUNCATED) | 50,334,649 |
"The Microsoft® Web Platform offers the right tools for the right task. Design, build, debug, and d(...TRUNCATED) | 50,334,661 |
"Star Trek: Paramount keen to avoid stigma of movie licenses\n\nStar Trek: The Video game launches i(...TRUNCATED) | 50,335,447 |
This is dataset is related to and based on the contents of The Pile Deduplicated.