GPT Neo
Overview
The GPTNeo model was released in the EleutherAI/gpt-neo repository by Sid Black, Stella Biderman, Leo Gao, Phil Wang and Connor Leahy. It is a GPT2 like causal language model trained on the Pile dataset.
The architecture is similar to GPT2 except that GPT Neo uses local attention in every other layer with a window size of 256 tokens.
This model was contributed by valhalla.
Usage example
The generate()
method can be used to generate text using GPT Neo model.
>>> from transformers import GPTNeoForCausalLM, GPT2Tokenizer
>>> model = GPTNeoForCausalLM.from_pretrained("EleutherAI/gpt-neo-1.3B")
>>> tokenizer = GPT2Tokenizer.from_pretrained("EleutherAI/gpt-neo-1.3B")
>>> prompt = (
... "In a shocking finding, scientists discovered a herd of unicorns living in a remote, "
... "previously unexplored valley, in the Andes Mountains. Even more surprising to the "
... "researchers was the fact that the unicorns spoke perfect English."
... )
>>> input_ids = tokenizer(prompt, return_tensors="pt").input_ids
>>> gen_tokens = model.generate(
... input_ids,
... do_sample=True,
... temperature=0.9,
... max_length=100,
... )
>>> gen_text = tokenizer.batch_decode(gen_tokens)[0]
Combining GPT-Neo and Flash Attention 2
First, make sure to install the latest version of Flash Attention 2 to include the sliding window attention feature, and make sure your hardware is compatible with Flash-Attention 2. More details are available here concerning the installation.
Make sure as well to load your model in half-precision (e.g. torch.float16
).
To load and run a model using Flash Attention 2, refer to the snippet below:
>>> import torch
>>> from transformers import AutoModelForCausalLM, AutoTokenizer
>>> device = "cuda" # the device to load the model onto
>>> model = AutoModelForCausalLM.from_pretrained("EleutherAI/gpt-neo-2.7B", torch_dtype=torch.float16, attn_implementation="flash_attention_2")
>>> tokenizer = AutoTokenizer.from_pretrained("EleutherAI/gpt-neo-2.7B")
>>> prompt = "def hello_world():"
>>> model_inputs = tokenizer([prompt], return_tensors="pt").to(device)
>>> model.to(device)
>>> generated_ids = model.generate(**model_inputs, max_new_tokens=100, do_sample=True)
>>> tokenizer.batch_decode(generated_ids)[0]
"def hello_world():\n >>> run_script("hello.py")\n >>> exit(0)\n<|endoftext|>"
Expected speedups
Below is an expected speedup diagram that compares pure inference time between the native implementation in transformers using EleutherAI/gpt-neo-2.7B
checkpoint and the Flash Attention 2 version of the model.
Note that for GPT-Neo it is not possible to train / run on very long context as the max position embeddings is limited to 2048 - but this is applicable to all gpt-neo models and not specific to FA-2
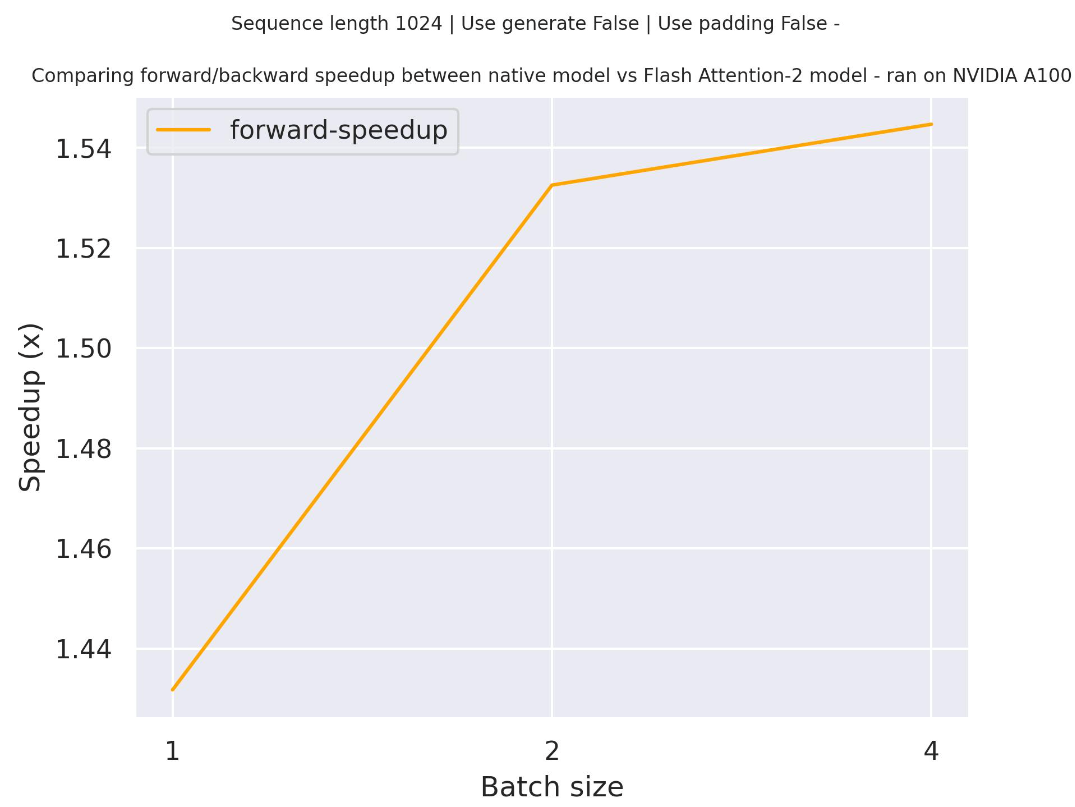
Resources
GPTNeoConfig
class transformers.GPTNeoConfig
< source >( vocab_size = 50257 max_position_embeddings = 2048 hidden_size = 2048 num_layers = 24 attention_types = [[['global', 'local'], 12]] num_heads = 16 intermediate_size = None window_size = 256 activation_function = 'gelu_new' resid_dropout = 0.0 embed_dropout = 0.0 attention_dropout = 0.0 classifier_dropout = 0.1 layer_norm_epsilon = 1e-05 initializer_range = 0.02 use_cache = True bos_token_id = 50256 eos_token_id = 50256 **kwargs )
Parameters
- vocab_size (
int
, optional, defaults to 50257) — Vocabulary size of the GPT Neo model. Defines the number of different tokens that can be represented by theinputs_ids
passed when calling GPTNeoModel. Vocabulary size of the model. Defines the different tokens that can be represented by the inputs_ids passed to the forward method of GPTNeoModel. - max_position_embeddings (
int
, optional, defaults to 2048) — The maximum sequence length that this model might ever be used with. Typically set this to something large just in case (e.g., 512 or 1024 or 2048). - hidden_size (
int
, optional, defaults to 2048) — Dimensionality of the encoder layers and the pooler layer. - num_layers (
int
, optional, defaults to 24) — Number of hidden layers in the Transformer encoder. - attention_types (
List
, optional, defaults to[[['global', 'local'], 12]]
) — The type of attention for each layer in aList
of the following format[[["attention_type"], num_layerss]]
e.g. for a 24 layer model[[["global"], 24]]
or[[["global", "local"], 12]]
Choose the value ofattention_type
from["global", "local"]
- num_heads (
int
, optional, defaults to 16) — Number of attention heads for each attention layer in the Transformer encoder. - intermediate_size (
int
, optional, defaults to 8192) — Dimensionality of the “intermediate” (i.e., feed-forward) layer in the Transformer encoder. - window_size (
int
, optional, defaults to 256) — The size of the sliding window for local attention. - activation_function (
str
orfunction
, optional, defaults to"gelu_new"
) — The non-linear activation function (function or string) in the encoder and pooler. If string,"gelu"
,"relu"
,"selu"
and"gelu_new"
are supported. - resid_dropout (
float
, optional, defaults to 0.0) — Residual dropout used in the attention pattern. - embed_dropout (
float
, optional, defaults to 0.0) — The dropout probability for all fully connected layers in the embeddings, encoder, and pooler. - attention_dropout (
float
, optional, defaults to 0.0) — The dropout ratio for the attention probabilities. - classifier_dropout (
float
, optional, defaults to 0.1) — Argument used when doing token classification, used in the model GPTNeoForTokenClassification. The dropout ratio for the hidden layer. - layer_norm_epsilon (
float
, optional, defaults to 1e-05) — The epsilon used by the layer normalization layers. - initializer_range (
float
, optional, defaults to 0.02) — The standard deviation of the truncated_normal_initializer for initializing all weight matrices. - use_cache (
bool
, optional, defaults toTrue
) — Whether or not the model should return the last key/values attentions (not used by all models). Only relevant ifconfig.is_decoder=True
. - bos_token_id (
int
, optional, defaults to 50256) — The id of the beginning of sentence token in the vocabulary. - eos_token_id (
int
, optional, defaults to 50256) — The id of the end of sentence token in the vocabulary.
This is the configuration class to store the configuration of a GPTNeoModel. It is used to instantiate a GPT Neo model according to the specified arguments, defining the model architecture. Instantiating a configuration with the defaults will yield a similar configuration to that of the GPTNeo EleutherAI/gpt-neo-1.3B architecture.
Configuration objects inherit from PretrainedConfig and can be used to control the model outputs. Read the documentation from PretrainedConfig for more information.
Example:
>>> from transformers import GPTNeoConfig, GPTNeoModel
>>> # Initializing a GPTNeo EleutherAI/gpt-neo-1.3B style configuration
>>> configuration = GPTNeoConfig()
>>> # Initializing a model (with random weights) from the EleutherAI/gpt-neo-1.3B style configuration
>>> model = GPTNeoModel(configuration)
>>> # Accessing the model configuration
>>> configuration = model.config
GPTNeoModel
class transformers.GPTNeoModel
< source >( config )
Parameters
- config (GPTNeoConfig) — Model configuration class with all the parameters of the model. Initializing with a config file does not load the weights associated with the model, only the configuration. Check out the from_pretrained() method to load the model weights.
The bare GPT Neo Model transformer outputting raw hidden-states without any specific head on top.
This model inherits from PreTrainedModel. Check the superclass documentation for the generic methods the library implements for all its model (such as downloading or saving, resizing the input embeddings, pruning heads etc.)
This model is also a PyTorch torch.nn.Module subclass. Use it as a regular PyTorch Module and refer to the PyTorch documentation for all matter related to general usage and behavior.
forward
< source >( input_ids: Optional = None past_key_values: Union = None attention_mask: Optional = None token_type_ids: Optional = None position_ids: Optional = None head_mask: Optional = None inputs_embeds: Optional = None use_cache: Optional = None output_attentions: Optional = None output_hidden_states: Optional = None return_dict: Optional = None cache_position: Optional = None ) β transformers.modeling_outputs.BaseModelOutputWithPastAndCrossAttentions or tuple(torch.FloatTensor)
Parameters
- input_ids (
torch.LongTensor
of shape(batch_size, input_ids_length)
) —input_ids_length
=sequence_length
ifpast_key_values
isNone
elsepast_key_values[0][0].shape[-2]
(sequence_length
of input past key value states). Indices of input sequence tokens in the vocabulary.If
past_key_values
is used, onlyinput_ids
that do not have their past calculated should be passed asinput_ids
.Indices can be obtained using AutoTokenizer. See PreTrainedTokenizer.encode() and PreTrainedTokenizer.call() for details.
- past_key_values (
Cache
ortuple(tuple(torch.FloatTensor))
, optional) — Pre-computed hidden-states (key and values in the self-attention blocks and in the cross-attention blocks) that can be used to speed up sequential decoding. This typically consists in thepast_key_values
returned by the model at a previous stage of decoding, whenuse_cache=True
orconfig.use_cache=True
.Two formats are allowed:
- a Cache instance, see our kv cache guide;
- Tuple of
tuple(torch.FloatTensor)
of lengthconfig.n_layers
, with each tuple having 2 tensors of shape(batch_size, num_heads, sequence_length, embed_size_per_head)
). This is also known as the legacy cache format.
The model will output the same cache format that is fed as input. If no
past_key_values
are passed, the legacy cache format will be returned.If
past_key_values
are used, the user can optionally input only the lastinput_ids
(those that don’t have their past key value states given to this model) of shape(batch_size, 1)
instead of allinput_ids
of shape(batch_size, sequence_length)
. - attention_mask (
torch.FloatTensor
of shape(batch_size, sequence_length)
, optional) — Mask to avoid performing attention on padding token indices. Mask values selected in[0, 1]
:- 1 for tokens that are not masked,
- 0 for tokens that are masked.
- token_type_ids (
torch.LongTensor
of shape(batch_size, input_ids_length)
, optional) — Segment token indices to indicate first and second portions of the inputs. Indices are selected in[0, 1]
:- 0 corresponds to a sentence A token,
- 1 corresponds to a sentence B token.
- position_ids (
torch.LongTensor
of shape(batch_size, sequence_length)
, optional) — Indices of positions of each input sequence tokens in the position embeddings. Selected in the range[0, config.max_position_embeddings - 1]
. - head_mask (
torch.FloatTensor
of shape(num_heads,)
or(num_layers, num_heads)
, optional) — Mask to nullify selected heads of the self-attention modules. Mask values selected in[0, 1]
:- 1 indicates the head is not masked,
- 0 indicates the head is masked.
- inputs_embeds (
torch.FloatTensor
of shape(batch_size, sequence_length, hidden_size)
, optional) — Optionally, instead of passinginput_ids
you can choose to directly pass an embedded representation. This is useful if you want more control over how to convertinput_ids
indices into associated vectors than the model’s internal embedding lookup matrix.If
past_key_values
is used, optionally only the lastinputs_embeds
have to be input (seepast_key_values
). - use_cache (
bool
, optional) — If set toTrue
,past_key_values
key value states are returned and can be used to speed up decoding (seepast_key_values
). - output_attentions (
bool
, optional) — Whether or not to return the attentions tensors of all attention layers. Seeattentions
under returned tensors for more detail. - output_hidden_states (
bool
, optional) — Whether or not to return the hidden states of all layers. Seehidden_states
under returned tensors for more detail. - return_dict (
bool
, optional) — Whether or not to return a ModelOutput instead of a plain tuple. - cache_position (
torch.LongTensor
of shape(sequence_length)
, optional) — Indices depicting the position of the input sequence tokens in the sequence. Contrarily toposition_ids
, this tensor is not affected by padding. It is used to update the cache in the correct position and to infer the complete sequence length.
Returns
transformers.modeling_outputs.BaseModelOutputWithPastAndCrossAttentions or tuple(torch.FloatTensor)
A transformers.modeling_outputs.BaseModelOutputWithPastAndCrossAttentions or a tuple of
torch.FloatTensor
(if return_dict=False
is passed or when config.return_dict=False
) comprising various
elements depending on the configuration (GPTNeoConfig) and inputs.
-
last_hidden_state (
torch.FloatTensor
of shape(batch_size, sequence_length, hidden_size)
) β Sequence of hidden-states at the output of the last layer of the model.If
past_key_values
is used only the last hidden-state of the sequences of shape(batch_size, 1, hidden_size)
is output. -
past_key_values (
tuple(tuple(torch.FloatTensor))
, optional, returned whenuse_cache=True
is passed or whenconfig.use_cache=True
) β Tuple oftuple(torch.FloatTensor)
of lengthconfig.n_layers
, with each tuple having 2 tensors of shape(batch_size, num_heads, sequence_length, embed_size_per_head)
) and optionally ifconfig.is_encoder_decoder=True
2 additional tensors of shape(batch_size, num_heads, encoder_sequence_length, embed_size_per_head)
.Contains pre-computed hidden-states (key and values in the self-attention blocks and optionally if
config.is_encoder_decoder=True
in the cross-attention blocks) that can be used (seepast_key_values
input) to speed up sequential decoding. -
hidden_states (
tuple(torch.FloatTensor)
, optional, returned whenoutput_hidden_states=True
is passed or whenconfig.output_hidden_states=True
) β Tuple oftorch.FloatTensor
(one for the output of the embeddings, if the model has an embedding layer, + one for the output of each layer) of shape(batch_size, sequence_length, hidden_size)
.Hidden-states of the model at the output of each layer plus the optional initial embedding outputs.
-
attentions (
tuple(torch.FloatTensor)
, optional, returned whenoutput_attentions=True
is passed or whenconfig.output_attentions=True
) β Tuple oftorch.FloatTensor
(one for each layer) of shape(batch_size, num_heads, sequence_length, sequence_length)
.Attentions weights after the attention softmax, used to compute the weighted average in the self-attention heads.
-
cross_attentions (
tuple(torch.FloatTensor)
, optional, returned whenoutput_attentions=True
andconfig.add_cross_attention=True
is passed or whenconfig.output_attentions=True
) β Tuple oftorch.FloatTensor
(one for each layer) of shape(batch_size, num_heads, sequence_length, sequence_length)
.Attentions weights of the decoderβs cross-attention layer, after the attention softmax, used to compute the weighted average in the cross-attention heads.
The GPTNeoModel forward method, overrides the __call__
special method.
Although the recipe for forward pass needs to be defined within this function, one should call the Module
instance afterwards instead of this since the former takes care of running the pre and post processing steps while
the latter silently ignores them.
Example:
>>> from transformers import AutoTokenizer, GPTNeoModel
>>> import torch
>>> tokenizer = AutoTokenizer.from_pretrained("EleutherAI/gpt-neo-1.3B")
>>> model = GPTNeoModel.from_pretrained("EleutherAI/gpt-neo-1.3B")
>>> inputs = tokenizer("Hello, my dog is cute", return_tensors="pt")
>>> outputs = model(**inputs)
>>> last_hidden_states = outputs.last_hidden_state
GPTNeoForCausalLM
class transformers.GPTNeoForCausalLM
< source >( config )
Parameters
- config (GPTNeoConfig) — Model configuration class with all the parameters of the model. Initializing with a config file does not load the weights associated with the model, only the configuration. Check out the from_pretrained() method to load the model weights.
The GPT Neo Model transformer with a language modeling head on top (linear layer with weights tied to the input embeddings).
This model inherits from PreTrainedModel. Check the superclass documentation for the generic methods the library implements for all its model (such as downloading or saving, resizing the input embeddings, pruning heads etc.)
This model is also a PyTorch torch.nn.Module subclass. Use it as a regular PyTorch Module and refer to the PyTorch documentation for all matter related to general usage and behavior.
forward
< source >( input_ids: Optional = None past_key_values: Union = None attention_mask: Optional = None token_type_ids: Optional = None position_ids: Optional = None head_mask: Optional = None inputs_embeds: Optional = None labels: Optional = None use_cache: Optional = None output_attentions: Optional = None output_hidden_states: Optional = None return_dict: Optional = None cache_position: Optional = None ) β transformers.modeling_outputs.CausalLMOutputWithCrossAttentions or tuple(torch.FloatTensor)
Parameters
- input_ids (
torch.LongTensor
of shape(batch_size, input_ids_length)
) —input_ids_length
=sequence_length
ifpast_key_values
isNone
elsepast_key_values[0][0].shape[-2]
(sequence_length
of input past key value states). Indices of input sequence tokens in the vocabulary.If
past_key_values
is used, onlyinput_ids
that do not have their past calculated should be passed asinput_ids
.Indices can be obtained using AutoTokenizer. See PreTrainedTokenizer.encode() and PreTrainedTokenizer.call() for details.
- past_key_values (
Cache
ortuple(tuple(torch.FloatTensor))
, optional) — Pre-computed hidden-states (key and values in the self-attention blocks and in the cross-attention blocks) that can be used to speed up sequential decoding. This typically consists in thepast_key_values
returned by the model at a previous stage of decoding, whenuse_cache=True
orconfig.use_cache=True
.Two formats are allowed:
- a Cache instance, see our kv cache guide;
- Tuple of
tuple(torch.FloatTensor)
of lengthconfig.n_layers
, with each tuple having 2 tensors of shape(batch_size, num_heads, sequence_length, embed_size_per_head)
). This is also known as the legacy cache format.
The model will output the same cache format that is fed as input. If no
past_key_values
are passed, the legacy cache format will be returned.If
past_key_values
are used, the user can optionally input only the lastinput_ids
(those that don’t have their past key value states given to this model) of shape(batch_size, 1)
instead of allinput_ids
of shape(batch_size, sequence_length)
. - attention_mask (
torch.FloatTensor
of shape(batch_size, sequence_length)
, optional) — Mask to avoid performing attention on padding token indices. Mask values selected in[0, 1]
:- 1 for tokens that are not masked,
- 0 for tokens that are masked.
- token_type_ids (
torch.LongTensor
of shape(batch_size, input_ids_length)
, optional) — Segment token indices to indicate first and second portions of the inputs. Indices are selected in[0, 1]
:- 0 corresponds to a sentence A token,
- 1 corresponds to a sentence B token.
- position_ids (
torch.LongTensor
of shape(batch_size, sequence_length)
, optional) — Indices of positions of each input sequence tokens in the position embeddings. Selected in the range[0, config.max_position_embeddings - 1]
. - head_mask (
torch.FloatTensor
of shape(num_heads,)
or(num_layers, num_heads)
, optional) — Mask to nullify selected heads of the self-attention modules. Mask values selected in[0, 1]
:- 1 indicates the head is not masked,
- 0 indicates the head is masked.
- inputs_embeds (
torch.FloatTensor
of shape(batch_size, sequence_length, hidden_size)
, optional) — Optionally, instead of passinginput_ids
you can choose to directly pass an embedded representation. This is useful if you want more control over how to convertinput_ids
indices into associated vectors than the model’s internal embedding lookup matrix.If
past_key_values
is used, optionally only the lastinputs_embeds
have to be input (seepast_key_values
). - use_cache (
bool
, optional) — If set toTrue
,past_key_values
key value states are returned and can be used to speed up decoding (seepast_key_values
). - output_attentions (
bool
, optional) — Whether or not to return the attentions tensors of all attention layers. Seeattentions
under returned tensors for more detail. - output_hidden_states (
bool
, optional) — Whether or not to return the hidden states of all layers. Seehidden_states
under returned tensors for more detail. - return_dict (
bool
, optional) — Whether or not to return a ModelOutput instead of a plain tuple. - cache_position (
torch.LongTensor
of shape(sequence_length)
, optional) — Indices depicting the position of the input sequence tokens in the sequence. Contrarily toposition_ids
, this tensor is not affected by padding. It is used to update the cache in the correct position and to infer the complete sequence length. - labels (
torch.LongTensor
of shape(batch_size, sequence_length)
, optional) — Labels for language modeling. Note that the labels are shifted inside the model, i.e. you can setlabels = input_ids
Indices are selected in[-100, 0, ..., config.vocab_size]
All labels set to-100
are ignored (masked), the loss is only computed for labels in[0, ..., config.vocab_size]
Returns
transformers.modeling_outputs.CausalLMOutputWithCrossAttentions or tuple(torch.FloatTensor)
A transformers.modeling_outputs.CausalLMOutputWithCrossAttentions or a tuple of
torch.FloatTensor
(if return_dict=False
is passed or when config.return_dict=False
) comprising various
elements depending on the configuration (GPTNeoConfig) and inputs.
-
loss (
torch.FloatTensor
of shape(1,)
, optional, returned whenlabels
is provided) β Language modeling loss (for next-token prediction). -
logits (
torch.FloatTensor
of shape(batch_size, sequence_length, config.vocab_size)
) β Prediction scores of the language modeling head (scores for each vocabulary token before SoftMax). -
hidden_states (
tuple(torch.FloatTensor)
, optional, returned whenoutput_hidden_states=True
is passed or whenconfig.output_hidden_states=True
) β Tuple oftorch.FloatTensor
(one for the output of the embeddings, if the model has an embedding layer, + one for the output of each layer) of shape(batch_size, sequence_length, hidden_size)
.Hidden-states of the model at the output of each layer plus the optional initial embedding outputs.
-
attentions (
tuple(torch.FloatTensor)
, optional, returned whenoutput_attentions=True
is passed or whenconfig.output_attentions=True
) β Tuple oftorch.FloatTensor
(one for each layer) of shape(batch_size, num_heads, sequence_length, sequence_length)
.Attentions weights after the attention softmax, used to compute the weighted average in the self-attention heads.
-
cross_attentions (
tuple(torch.FloatTensor)
, optional, returned whenoutput_attentions=True
is passed or whenconfig.output_attentions=True
) β Tuple oftorch.FloatTensor
(one for each layer) of shape(batch_size, num_heads, sequence_length, sequence_length)
.Cross attentions weights after the attention softmax, used to compute the weighted average in the cross-attention heads.
-
past_key_values (
tuple(tuple(torch.FloatTensor))
, optional, returned whenuse_cache=True
is passed or whenconfig.use_cache=True
) β Tuple oftorch.FloatTensor
tuples of lengthconfig.n_layers
, with each tuple containing the cached key, value states of the self-attention and the cross-attention layers if model is used in encoder-decoder setting. Only relevant ifconfig.is_decoder = True
.Contains pre-computed hidden-states (key and values in the attention blocks) that can be used (see
past_key_values
input) to speed up sequential decoding.
The GPTNeoForCausalLM forward method, overrides the __call__
special method.
Although the recipe for forward pass needs to be defined within this function, one should call the Module
instance afterwards instead of this since the former takes care of running the pre and post processing steps while
the latter silently ignores them.
Example:
>>> import torch
>>> from transformers import AutoTokenizer, GPTNeoForCausalLM
>>> tokenizer = AutoTokenizer.from_pretrained("EleutherAI/gpt-neo-1.3B")
>>> model = GPTNeoForCausalLM.from_pretrained("EleutherAI/gpt-neo-1.3B")
>>> inputs = tokenizer("Hello, my dog is cute", return_tensors="pt")
>>> outputs = model(**inputs, labels=inputs["input_ids"])
>>> loss = outputs.loss
>>> logits = outputs.logits
GPTNeoForQuestionAnswering
class transformers.GPTNeoForQuestionAnswering
< source >( config )
Parameters
- config (GPTNeoConfig) — Model configuration class with all the parameters of the model. Initializing with a config file does not load the weights associated with the model, only the configuration. Check out the from_pretrained() method to load the model weights.
The GPT-Neo Model transformer with a span classification head on top for extractive question-answering tasks like
SQuAD (a linear layer on top of the hidden-states output to compute span start logits
and span end logits
).
This model inherits from PreTrainedModel. Check the superclass documentation for the generic methods the library implements for all its model (such as downloading or saving, resizing the input embeddings, pruning heads etc.)
This model is also a PyTorch torch.nn.Module subclass. Use it as a regular PyTorch Module and refer to the PyTorch documentation for all matter related to general usage and behavior.
forward
< source >( input_ids: Optional = None attention_mask: Optional = None token_type_ids: Optional = None position_ids: Optional = None head_mask: Optional = None inputs_embeds: Optional = None start_positions: Optional = None end_positions: Optional = None output_attentions: Optional = None output_hidden_states: Optional = None return_dict: Optional = None ) β transformers.modeling_outputs.QuestionAnsweringModelOutput or tuple(torch.FloatTensor)
Parameters
- input_ids (
torch.LongTensor
of shape(batch_size, input_ids_length)
) —input_ids_length
=sequence_length
ifpast_key_values
isNone
elsepast_key_values[0][0].shape[-2]
(sequence_length
of input past key value states). Indices of input sequence tokens in the vocabulary.If
past_key_values
is used, onlyinput_ids
that do not have their past calculated should be passed asinput_ids
.Indices can be obtained using AutoTokenizer. See PreTrainedTokenizer.encode() and PreTrainedTokenizer.call() for details.
- past_key_values (
Cache
ortuple(tuple(torch.FloatTensor))
, optional) — Pre-computed hidden-states (key and values in the self-attention blocks and in the cross-attention blocks) that can be used to speed up sequential decoding. This typically consists in thepast_key_values
returned by the model at a previous stage of decoding, whenuse_cache=True
orconfig.use_cache=True
.Two formats are allowed:
- a Cache instance, see our kv cache guide;
- Tuple of
tuple(torch.FloatTensor)
of lengthconfig.n_layers
, with each tuple having 2 tensors of shape(batch_size, num_heads, sequence_length, embed_size_per_head)
). This is also known as the legacy cache format.
The model will output the same cache format that is fed as input. If no
past_key_values
are passed, the legacy cache format will be returned.If
past_key_values
are used, the user can optionally input only the lastinput_ids
(those that don’t have their past key value states given to this model) of shape(batch_size, 1)
instead of allinput_ids
of shape(batch_size, sequence_length)
. - attention_mask (
torch.FloatTensor
of shape(batch_size, sequence_length)
, optional) — Mask to avoid performing attention on padding token indices. Mask values selected in[0, 1]
:- 1 for tokens that are not masked,
- 0 for tokens that are masked.
- token_type_ids (
torch.LongTensor
of shape(batch_size, input_ids_length)
, optional) — Segment token indices to indicate first and second portions of the inputs. Indices are selected in[0, 1]
:- 0 corresponds to a sentence A token,
- 1 corresponds to a sentence B token.
- position_ids (
torch.LongTensor
of shape(batch_size, sequence_length)
, optional) — Indices of positions of each input sequence tokens in the position embeddings. Selected in the range[0, config.max_position_embeddings - 1]
. - head_mask (
torch.FloatTensor
of shape(num_heads,)
or(num_layers, num_heads)
, optional) — Mask to nullify selected heads of the self-attention modules. Mask values selected in[0, 1]
:- 1 indicates the head is not masked,
- 0 indicates the head is masked.
- inputs_embeds (
torch.FloatTensor
of shape(batch_size, sequence_length, hidden_size)
, optional) — Optionally, instead of passinginput_ids
you can choose to directly pass an embedded representation. This is useful if you want more control over how to convertinput_ids
indices into associated vectors than the model’s internal embedding lookup matrix.If
past_key_values
is used, optionally only the lastinputs_embeds
have to be input (seepast_key_values
). - use_cache (
bool
, optional) — If set toTrue
,past_key_values
key value states are returned and can be used to speed up decoding (seepast_key_values
). - output_attentions (
bool
, optional) — Whether or not to return the attentions tensors of all attention layers. Seeattentions
under returned tensors for more detail. - output_hidden_states (
bool
, optional) — Whether or not to return the hidden states of all layers. Seehidden_states
under returned tensors for more detail. - return_dict (
bool
, optional) — Whether or not to return a ModelOutput instead of a plain tuple. - cache_position (
torch.LongTensor
of shape(sequence_length)
, optional) — Indices depicting the position of the input sequence tokens in the sequence. Contrarily toposition_ids
, this tensor is not affected by padding. It is used to update the cache in the correct position and to infer the complete sequence length. - start_positions (
torch.LongTensor
of shape(batch_size,)
, optional) — Labels for position (index) of the start of the labelled span for computing the token classification loss. Positions are clamped to the length of the sequence (sequence_length
). Position outside of the sequence are not taken into account for computing the loss. - end_positions (
torch.LongTensor
of shape(batch_size,)
, optional) — Labels for position (index) of the end of the labelled span for computing the token classification loss. Positions are clamped to the length of the sequence (sequence_length
). Position outside of the sequence are not taken into account for computing the loss.
Returns
transformers.modeling_outputs.QuestionAnsweringModelOutput or tuple(torch.FloatTensor)
A transformers.modeling_outputs.QuestionAnsweringModelOutput or a tuple of
torch.FloatTensor
(if return_dict=False
is passed or when config.return_dict=False
) comprising various
elements depending on the configuration (GPTNeoConfig) and inputs.
-
loss (
torch.FloatTensor
of shape(1,)
, optional, returned whenlabels
is provided) β Total span extraction loss is the sum of a Cross-Entropy for the start and end positions. -
start_logits (
torch.FloatTensor
of shape(batch_size, sequence_length)
) β Span-start scores (before SoftMax). -
end_logits (
torch.FloatTensor
of shape(batch_size, sequence_length)
) β Span-end scores (before SoftMax). -
hidden_states (
tuple(torch.FloatTensor)
, optional, returned whenoutput_hidden_states=True
is passed or whenconfig.output_hidden_states=True
) β Tuple oftorch.FloatTensor
(one for the output of the embeddings, if the model has an embedding layer, + one for the output of each layer) of shape(batch_size, sequence_length, hidden_size)
.Hidden-states of the model at the output of each layer plus the optional initial embedding outputs.
-
attentions (
tuple(torch.FloatTensor)
, optional, returned whenoutput_attentions=True
is passed or whenconfig.output_attentions=True
) β Tuple oftorch.FloatTensor
(one for each layer) of shape(batch_size, num_heads, sequence_length, sequence_length)
.Attentions weights after the attention softmax, used to compute the weighted average in the self-attention heads.
The GPTNeoForQuestionAnswering forward method, overrides the __call__
special method.
Although the recipe for forward pass needs to be defined within this function, one should call the Module
instance afterwards instead of this since the former takes care of running the pre and post processing steps while
the latter silently ignores them.
This example uses a random model as the real ones are all very big. To get proper results, you should use
EleutherAI/gpt-neo-1.3B instead of EleutherAI/gpt-neo-1.3B. If you get out-of-memory when loading that checkpoint, you can try
adding device_map="auto"
in the from_pretrained
call.
Example:
>>> from transformers import AutoTokenizer, GPTNeoForQuestionAnswering
>>> import torch
>>> tokenizer = AutoTokenizer.from_pretrained("EleutherAI/gpt-neo-1.3B")
>>> model = GPTNeoForQuestionAnswering.from_pretrained("EleutherAI/gpt-neo-1.3B")
>>> question, text = "Who was Jim Henson?", "Jim Henson was a nice puppet"
>>> inputs = tokenizer(question, text, return_tensors="pt")
>>> with torch.no_grad():
... outputs = model(**inputs)
>>> answer_start_index = outputs.start_logits.argmax()
>>> answer_end_index = outputs.end_logits.argmax()
>>> predict_answer_tokens = inputs.input_ids[0, answer_start_index : answer_end_index + 1]
>>> # target is "nice puppet"
>>> target_start_index = torch.tensor([14])
>>> target_end_index = torch.tensor([15])
>>> outputs = model(**inputs, start_positions=target_start_index, end_positions=target_end_index)
>>> loss = outputs.loss
GPTNeoForSequenceClassification
class transformers.GPTNeoForSequenceClassification
< source >( config )
Parameters
- config (GPTNeoConfig) — Model configuration class with all the parameters of the model. Initializing with a config file does not load the weights associated with the model, only the configuration. Check out the from_pretrained() method to load the model weights.
The GPTNeo Model transformer with a sequence classification head on top (linear layer).
GPTNeoForSequenceClassification uses the last token in order to do the classification, as other causal models (e.g. GPT-1) do.
Since it does classification on the last token, it requires to know the position of the last token. If a
pad_token_id
is defined in the configuration, it finds the last token that is not a padding token in each row. If
no pad_token_id
is defined, it simply takes the last value in each row of the batch. Since it cannot guess the
padding tokens when inputs_embeds
are passed instead of input_ids
, it does the same (take the last value in
each row of the batch).
This model inherits from PreTrainedModel. Check the superclass documentation for the generic methods the library implements for all its model (such as downloading or saving, resizing the input embeddings, pruning heads etc.)
This model is also a PyTorch torch.nn.Module subclass. Use it as a regular PyTorch Module and refer to the PyTorch documentation for all matter related to general usage and behavior.
forward
< source >( input_ids: Optional = None past_key_values: Union = None attention_mask: Optional = None token_type_ids: Optional = None position_ids: Optional = None head_mask: Optional = None inputs_embeds: Optional = None labels: Optional = None use_cache: Optional = None output_attentions: Optional = None output_hidden_states: Optional = None return_dict: Optional = None ) β transformers.modeling_outputs.SequenceClassifierOutputWithPast
or tuple(torch.FloatTensor)
Parameters
- input_ids (
torch.LongTensor
of shape(batch_size, input_ids_length)
) —input_ids_length
=sequence_length
ifpast_key_values
isNone
elsepast_key_values[0][0].shape[-2]
(sequence_length
of input past key value states). Indices of input sequence tokens in the vocabulary.If
past_key_values
is used, onlyinput_ids
that do not have their past calculated should be passed asinput_ids
.Indices can be obtained using AutoTokenizer. See PreTrainedTokenizer.encode() and PreTrainedTokenizer.call() for details.
- past_key_values (
Cache
ortuple(tuple(torch.FloatTensor))
, optional) — Pre-computed hidden-states (key and values in the self-attention blocks and in the cross-attention blocks) that can be used to speed up sequential decoding. This typically consists in thepast_key_values
returned by the model at a previous stage of decoding, whenuse_cache=True
orconfig.use_cache=True
.Two formats are allowed:
- a Cache instance, see our kv cache guide;
- Tuple of
tuple(torch.FloatTensor)
of lengthconfig.n_layers
, with each tuple having 2 tensors of shape(batch_size, num_heads, sequence_length, embed_size_per_head)
). This is also known as the legacy cache format.
The model will output the same cache format that is fed as input. If no
past_key_values
are passed, the legacy cache format will be returned.If
past_key_values
are used, the user can optionally input only the lastinput_ids
(those that don’t have their past key value states given to this model) of shape(batch_size, 1)
instead of allinput_ids
of shape(batch_size, sequence_length)
. - attention_mask (
torch.FloatTensor
of shape(batch_size, sequence_length)
, optional) — Mask to avoid performing attention on padding token indices. Mask values selected in[0, 1]
:- 1 for tokens that are not masked,
- 0 for tokens that are masked.
- token_type_ids (
torch.LongTensor
of shape(batch_size, input_ids_length)
, optional) — Segment token indices to indicate first and second portions of the inputs. Indices are selected in[0, 1]
:- 0 corresponds to a sentence A token,
- 1 corresponds to a sentence B token.
- position_ids (
torch.LongTensor
of shape(batch_size, sequence_length)
, optional) — Indices of positions of each input sequence tokens in the position embeddings. Selected in the range[0, config.max_position_embeddings - 1]
. - head_mask (
torch.FloatTensor
of shape(num_heads,)
or(num_layers, num_heads)
, optional) — Mask to nullify selected heads of the self-attention modules. Mask values selected in[0, 1]
:- 1 indicates the head is not masked,
- 0 indicates the head is masked.
- inputs_embeds (
torch.FloatTensor
of shape(batch_size, sequence_length, hidden_size)
, optional) — Optionally, instead of passinginput_ids
you can choose to directly pass an embedded representation. This is useful if you want more control over how to convertinput_ids
indices into associated vectors than the model’s internal embedding lookup matrix.If
past_key_values
is used, optionally only the lastinputs_embeds
have to be input (seepast_key_values
). - use_cache (
bool
, optional) — If set toTrue
,past_key_values
key value states are returned and can be used to speed up decoding (seepast_key_values
). - output_attentions (
bool
, optional) — Whether or not to return the attentions tensors of all attention layers. Seeattentions
under returned tensors for more detail. - output_hidden_states (
bool
, optional) — Whether or not to return the hidden states of all layers. Seehidden_states
under returned tensors for more detail. - return_dict (
bool
, optional) — Whether or not to return a ModelOutput instead of a plain tuple. - cache_position (
torch.LongTensor
of shape(sequence_length)
, optional) — Indices depicting the position of the input sequence tokens in the sequence. Contrarily toposition_ids
, this tensor is not affected by padding. It is used to update the cache in the correct position and to infer the complete sequence length. - labels (
torch.LongTensor
of shape(batch_size,)
, optional) — Labels for computing the sequence classification/regression loss. Indices should be in[0, ..., config.num_labels - 1]
. Ifconfig.num_labels == 1
a regression loss is computed (Mean-Square loss), Ifconfig.num_labels > 1
a classification loss is computed (Cross-Entropy).
Returns
transformers.modeling_outputs.SequenceClassifierOutputWithPast
or tuple(torch.FloatTensor)
A transformers.modeling_outputs.SequenceClassifierOutputWithPast
or a tuple of
torch.FloatTensor
(if return_dict=False
is passed or when config.return_dict=False
) comprising various
elements depending on the configuration (GPTNeoConfig) and inputs.
-
loss (
torch.FloatTensor
of shape(1,)
, optional, returned whenlabels
is provided) β Classification (or regression if config.num_labels==1) loss. -
logits (
torch.FloatTensor
of shape(batch_size, config.num_labels)
) β Classification (or regression if config.num_labels==1) scores (before SoftMax). -
past_key_values (
tuple(tuple(torch.FloatTensor))
, optional, returned whenuse_cache=True
is passed or whenconfig.use_cache=True
) β Tuple oftuple(torch.FloatTensor)
of lengthconfig.n_layers
, with each tuple having 2 tensors of shape(batch_size, num_heads, sequence_length, embed_size_per_head)
)Contains pre-computed hidden-states (key and values in the self-attention blocks) that can be used (see
past_key_values
input) to speed up sequential decoding. -
hidden_states (
tuple(torch.FloatTensor)
, optional, returned whenoutput_hidden_states=True
is passed or whenconfig.output_hidden_states=True
) β Tuple oftorch.FloatTensor
(one for the output of the embeddings, if the model has an embedding layer, + one for the output of each layer) of shape(batch_size, sequence_length, hidden_size)
.Hidden-states of the model at the output of each layer plus the optional initial embedding outputs.
-
attentions (
tuple(torch.FloatTensor)
, optional, returned whenoutput_attentions=True
is passed or whenconfig.output_attentions=True
) β Tuple oftorch.FloatTensor
(one for each layer) of shape(batch_size, num_heads, sequence_length, sequence_length)
.Attentions weights after the attention softmax, used to compute the weighted average in the self-attention heads.
The GPTNeoForSequenceClassification forward method, overrides the __call__
special method.
Although the recipe for forward pass needs to be defined within this function, one should call the Module
instance afterwards instead of this since the former takes care of running the pre and post processing steps while
the latter silently ignores them.
Example of single-label classification:
>>> import torch
>>> from transformers import AutoTokenizer, GPTNeoForSequenceClassification
>>> tokenizer = AutoTokenizer.from_pretrained("EleutherAI/gpt-neo-1.3B")
>>> model = GPTNeoForSequenceClassification.from_pretrained("EleutherAI/gpt-neo-1.3B")
>>> inputs = tokenizer("Hello, my dog is cute", return_tensors="pt")
>>> with torch.no_grad():
... logits = model(**inputs).logits
>>> predicted_class_id = logits.argmax().item()
>>> # To train a model on `num_labels` classes, you can pass `num_labels=num_labels` to `.from_pretrained(...)`
>>> num_labels = len(model.config.id2label)
>>> model = GPTNeoForSequenceClassification.from_pretrained("EleutherAI/gpt-neo-1.3B", num_labels=num_labels)
>>> labels = torch.tensor([1])
>>> loss = model(**inputs, labels=labels).loss
Example of multi-label classification:
>>> import torch
>>> from transformers import AutoTokenizer, GPTNeoForSequenceClassification
>>> tokenizer = AutoTokenizer.from_pretrained("EleutherAI/gpt-neo-1.3B")
>>> model = GPTNeoForSequenceClassification.from_pretrained("EleutherAI/gpt-neo-1.3B", problem_type="multi_label_classification")
>>> inputs = tokenizer("Hello, my dog is cute", return_tensors="pt")
>>> with torch.no_grad():
... logits = model(**inputs).logits
>>> predicted_class_ids = torch.arange(0, logits.shape[-1])[torch.sigmoid(logits).squeeze(dim=0) > 0.5]
>>> # To train a model on `num_labels` classes, you can pass `num_labels=num_labels` to `.from_pretrained(...)`
>>> num_labels = len(model.config.id2label)
>>> model = GPTNeoForSequenceClassification.from_pretrained(
... "EleutherAI/gpt-neo-1.3B", num_labels=num_labels, problem_type="multi_label_classification"
... )
>>> labels = torch.sum(
... torch.nn.functional.one_hot(predicted_class_ids[None, :].clone(), num_classes=num_labels), dim=1
... ).to(torch.float)
>>> loss = model(**inputs, labels=labels).loss
GPTNeoForTokenClassification
class transformers.GPTNeoForTokenClassification
< source >( config )
Parameters
- config (GPTNeoConfig) — Model configuration class with all the parameters of the model. Initializing with a config file does not load the weights associated with the model, only the configuration. Check out the from_pretrained() method to load the model weights.
GPT Neo model with a token classification head on top (a linear layer on top of the hidden-states output) e.g. for Named-Entity-Recognition (NER) tasks.
This model inherits from PreTrainedModel. Check the superclass documentation for the generic methods the library implements for all its model (such as downloading or saving, resizing the input embeddings, pruning heads etc.)
This model is also a PyTorch torch.nn.Module subclass. Use it as a regular PyTorch Module and refer to the PyTorch documentation for all matter related to general usage and behavior.
forward
< source >( input_ids: Optional = None past_key_values: Union = None attention_mask: Optional = None token_type_ids: Optional = None position_ids: Optional = None head_mask: Optional = None inputs_embeds: Optional = None labels: Optional = None use_cache: Optional = None output_attentions: Optional = None output_hidden_states: Optional = None return_dict: Optional = None ) β transformers.modeling_outputs.TokenClassifierOutput or tuple(torch.FloatTensor)
Parameters
- input_ids (
torch.LongTensor
of shape(batch_size, input_ids_length)
) —input_ids_length
=sequence_length
ifpast_key_values
isNone
elsepast_key_values[0][0].shape[-2]
(sequence_length
of input past key value states). Indices of input sequence tokens in the vocabulary.If
past_key_values
is used, onlyinput_ids
that do not have their past calculated should be passed asinput_ids
.Indices can be obtained using AutoTokenizer. See PreTrainedTokenizer.encode() and PreTrainedTokenizer.call() for details.
- past_key_values (
Cache
ortuple(tuple(torch.FloatTensor))
, optional) — Pre-computed hidden-states (key and values in the self-attention blocks and in the cross-attention blocks) that can be used to speed up sequential decoding. This typically consists in thepast_key_values
returned by the model at a previous stage of decoding, whenuse_cache=True
orconfig.use_cache=True
.Two formats are allowed:
- a Cache instance, see our kv cache guide;
- Tuple of
tuple(torch.FloatTensor)
of lengthconfig.n_layers
, with each tuple having 2 tensors of shape(batch_size, num_heads, sequence_length, embed_size_per_head)
). This is also known as the legacy cache format.
The model will output the same cache format that is fed as input. If no
past_key_values
are passed, the legacy cache format will be returned.If
past_key_values
are used, the user can optionally input only the lastinput_ids
(those that don’t have their past key value states given to this model) of shape(batch_size, 1)
instead of allinput_ids
of shape(batch_size, sequence_length)
. - attention_mask (
torch.FloatTensor
of shape(batch_size, sequence_length)
, optional) — Mask to avoid performing attention on padding token indices. Mask values selected in[0, 1]
:- 1 for tokens that are not masked,
- 0 for tokens that are masked.
- token_type_ids (
torch.LongTensor
of shape(batch_size, input_ids_length)
, optional) — Segment token indices to indicate first and second portions of the inputs. Indices are selected in[0, 1]
:- 0 corresponds to a sentence A token,
- 1 corresponds to a sentence B token.
- position_ids (
torch.LongTensor
of shape(batch_size, sequence_length)
, optional) — Indices of positions of each input sequence tokens in the position embeddings. Selected in the range[0, config.max_position_embeddings - 1]
. - head_mask (
torch.FloatTensor
of shape(num_heads,)
or(num_layers, num_heads)
, optional) — Mask to nullify selected heads of the self-attention modules. Mask values selected in[0, 1]
:- 1 indicates the head is not masked,
- 0 indicates the head is masked.
- inputs_embeds (
torch.FloatTensor
of shape(batch_size, sequence_length, hidden_size)
, optional) — Optionally, instead of passinginput_ids
you can choose to directly pass an embedded representation. This is useful if you want more control over how to convertinput_ids
indices into associated vectors than the model’s internal embedding lookup matrix.If
past_key_values
is used, optionally only the lastinputs_embeds
have to be input (seepast_key_values
). - use_cache (
bool
, optional) — If set toTrue
,past_key_values
key value states are returned and can be used to speed up decoding (seepast_key_values
). - output_attentions (
bool
, optional) — Whether or not to return the attentions tensors of all attention layers. Seeattentions
under returned tensors for more detail. - output_hidden_states (
bool
, optional) — Whether or not to return the hidden states of all layers. Seehidden_states
under returned tensors for more detail. - return_dict (
bool
, optional) — Whether or not to return a ModelOutput instead of a plain tuple. - cache_position (
torch.LongTensor
of shape(sequence_length)
, optional) — Indices depicting the position of the input sequence tokens in the sequence. Contrarily toposition_ids
, this tensor is not affected by padding. It is used to update the cache in the correct position and to infer the complete sequence length. - labels (
torch.LongTensor
of shape(batch_size, sequence_length)
, optional) — Labels for computing the sequence classification/regression loss. Indices should be in[0, ..., config.num_labels - 1]
. Ifconfig.num_labels == 1
a regression loss is computed (Mean-Square loss), Ifconfig.num_labels > 1
a classification loss is computed (Cross-Entropy).
Returns
transformers.modeling_outputs.TokenClassifierOutput or tuple(torch.FloatTensor)
A transformers.modeling_outputs.TokenClassifierOutput or a tuple of
torch.FloatTensor
(if return_dict=False
is passed or when config.return_dict=False
) comprising various
elements depending on the configuration (GPTNeoConfig) and inputs.
-
loss (
torch.FloatTensor
of shape(1,)
, optional, returned whenlabels
is provided) β Classification loss. -
logits (
torch.FloatTensor
of shape(batch_size, sequence_length, config.num_labels)
) β Classification scores (before SoftMax). -
hidden_states (
tuple(torch.FloatTensor)
, optional, returned whenoutput_hidden_states=True
is passed or whenconfig.output_hidden_states=True
) β Tuple oftorch.FloatTensor
(one for the output of the embeddings, if the model has an embedding layer, + one for the output of each layer) of shape(batch_size, sequence_length, hidden_size)
.Hidden-states of the model at the output of each layer plus the optional initial embedding outputs.
-
attentions (
tuple(torch.FloatTensor)
, optional, returned whenoutput_attentions=True
is passed or whenconfig.output_attentions=True
) β Tuple oftorch.FloatTensor
(one for each layer) of shape(batch_size, num_heads, sequence_length, sequence_length)
.Attentions weights after the attention softmax, used to compute the weighted average in the self-attention heads.
The GPTNeoForTokenClassification forward method, overrides the __call__
special method.
Although the recipe for forward pass needs to be defined within this function, one should call the Module
instance afterwards instead of this since the former takes care of running the pre and post processing steps while
the latter silently ignores them.
Example:
>>> from transformers import AutoTokenizer, GPTNeoForTokenClassification
>>> import torch
>>> tokenizer = AutoTokenizer.from_pretrained("EleutherAI/gpt-neo-125m")
>>> model = GPTNeoForTokenClassification.from_pretrained("EleutherAI/gpt-neo-125m")
>>> inputs = tokenizer(
... "HuggingFace is a company based in Paris and New York", add_special_tokens=False, return_tensors="pt"
... )
>>> with torch.no_grad():
... logits = model(**inputs).logits
>>> predicted_token_class_ids = logits.argmax(-1)
>>> # Note that tokens are classified rather then input words which means that
>>> # there might be more predicted token classes than words.
>>> # Multiple token classes might account for the same word
>>> predicted_tokens_classes = [model.config.id2label[t.item()] for t in predicted_token_class_ids[0]]
>>> labels = predicted_token_class_ids
>>> loss = model(**inputs, labels=labels).loss
>>> round(loss.item(), 2)
0.25
FlaxGPTNeoModel
class transformers.FlaxGPTNeoModel
< source >( config: GPTNeoConfig input_shape: Tuple = (1, 1) seed: int = 0 dtype: dtype = <class 'jax.numpy.float32'> _do_init: bool = True **kwargs )
Parameters
- config (GPTNeoConfig) — Model configuration class with all the parameters of the model. Initializing with a config file does not load the weights associated with the model, only the configuration. Check out the from_pretrained() method to load the model weights.
- dtype (
jax.numpy.dtype
, optional, defaults tojax.numpy.float32
) — The data type of the computation. Can be one ofjax.numpy.float32
,jax.numpy.float16
(on GPUs) andjax.numpy.bfloat16
(on TPUs).This can be used to enable mixed-precision training or half-precision inference on GPUs or TPUs. If specified all the computation will be performed with the given
dtype
.Note that this only specifies the dtype of the computation and does not influence the dtype of model parameters.
If you wish to change the dtype of the model parameters, see to_fp16() and to_bf16().
The bare GPTNeo Model transformer outputting raw hidden-states without any specific head on top.
This model inherits from FlaxPreTrainedModel. Check the superclass documentation for the generic methods the library implements for all its model (such as downloading or saving, resizing the input embeddings, pruning heads etc.)
This model is also a Flax Linen flax.nn.Module subclass. Use it as a regular Flax Module and refer to the Flax documentation for all matter related to general usage and behavior.
Finally, this model supports inherent JAX features such as:
__call__
< source >( input_ids attention_mask = None position_ids = None params: dict = None past_key_values: dict = None dropout_rng: PRNGKey = None train: bool = False output_attentions: Optional = None output_hidden_states: Optional = None return_dict: Optional = None ) β transformers.modeling_flax_outputs.FlaxBaseModelOutput or tuple(torch.FloatTensor)
Parameters
- input_ids (
numpy.ndarray
of shape(batch_size, input_ids_length)
) —input_ids_length
=sequence_length
. Indices of input sequence tokens in the vocabulary.Indices can be obtained using AutoTokenizer. See PreTrainedTokenizer.encode() and PreTrainedTokenizer.call() for details.
- attention_mask (
numpy.ndarray
of shape(batch_size, sequence_length)
, optional) — Mask to avoid performing attention on padding token indices. Mask values selected in[0, 1]
:- 1 for tokens that are not masked,
- 0 for tokens that are masked.
- position_ids (
numpy.ndarray
of shape(batch_size, sequence_length)
, optional) — Indices of positions of each input sequence tokens in the position embeddings. Selected in the range[0, config.max_position_embeddings - 1]
. - past_key_values (
Dict[str, np.ndarray]
, optional, returned byinit_cache
or when passing previouspast_key_values
) — Dictionary of pre-computed hidden-states (key and values in the attention blocks) that can be used for fast auto-regressive decoding. Pre-computed key and value hidden-states are of shape [batch_size, max_length]. - output_attentions (
bool
, optional) — Whether or not to return the attentions tensors of all attention layers. Seeattentions
under returned tensors for more detail. - output_hidden_states (
bool
, optional) — Whether or not to return the hidden states of all layers. Seehidden_states
under returned tensors for more detail. - return_dict (
bool
, optional) — Whether or not to return a ModelOutput instead of a plain tuple.
Returns
transformers.modeling_flax_outputs.FlaxBaseModelOutput or tuple(torch.FloatTensor)
A transformers.modeling_flax_outputs.FlaxBaseModelOutput or a tuple of
torch.FloatTensor
(if return_dict=False
is passed or when config.return_dict=False
) comprising various
elements depending on the configuration (GPTNeoConfig) and inputs.
-
last_hidden_state (
jnp.ndarray
of shape(batch_size, sequence_length, hidden_size)
) β Sequence of hidden-states at the output of the last layer of the model. -
hidden_states (
tuple(jnp.ndarray)
, optional, returned whenoutput_hidden_states=True
is passed or whenconfig.output_hidden_states=True
) β Tuple ofjnp.ndarray
(one for the output of the embeddings + one for the output of each layer) of shape(batch_size, sequence_length, hidden_size)
.Hidden-states of the model at the output of each layer plus the initial embedding outputs.
-
attentions (
tuple(jnp.ndarray)
, optional, returned whenoutput_attentions=True
is passed or whenconfig.output_attentions=True
) β Tuple ofjnp.ndarray
(one for each layer) of shape(batch_size, num_heads, sequence_length, sequence_length)
.Attentions weights after the attention softmax, used to compute the weighted average in the self-attention heads.
The FlaxGPTNeoPreTrainedModel
forward method, overrides the __call__
special method.
Although the recipe for forward pass needs to be defined within this function, one should call the Module
instance afterwards instead of this since the former takes care of running the pre and post processing steps while
the latter silently ignores them.
Example:
>>> from transformers import AutoTokenizer, FlaxGPTNeoModel
>>> tokenizer = AutoTokenizer.from_pretrained("EleutherAI/gpt-neo-1.3B")
>>> model = FlaxGPTNeoModel.from_pretrained("EleutherAI/gpt-neo-1.3B")
>>> inputs = tokenizer("Hello, my dog is cute", return_tensors="jax")
>>> outputs = model(**inputs)
>>> last_hidden_states = outputs.last_hidden_state
FlaxGPTNeoForCausalLM
class transformers.FlaxGPTNeoForCausalLM
< source >( config: GPTNeoConfig input_shape: Tuple = (1, 1) seed: int = 0 dtype: dtype = <class 'jax.numpy.float32'> _do_init: bool = True **kwargs )
Parameters
- config (GPTNeoConfig) — Model configuration class with all the parameters of the model. Initializing with a config file does not load the weights associated with the model, only the configuration. Check out the from_pretrained() method to load the model weights.
- dtype (
jax.numpy.dtype
, optional, defaults tojax.numpy.float32
) — The data type of the computation. Can be one ofjax.numpy.float32
,jax.numpy.float16
(on GPUs) andjax.numpy.bfloat16
(on TPUs).This can be used to enable mixed-precision training or half-precision inference on GPUs or TPUs. If specified all the computation will be performed with the given
dtype
.Note that this only specifies the dtype of the computation and does not influence the dtype of model parameters.
If you wish to change the dtype of the model parameters, see to_fp16() and to_bf16().
The GPTNeo Model transformer with a language modeling head on top (linear layer with weights tied to the input embeddings).
This model inherits from FlaxPreTrainedModel. Check the superclass documentation for the generic methods the library implements for all its model (such as downloading or saving, resizing the input embeddings, pruning heads etc.)
This model is also a Flax Linen flax.nn.Module subclass. Use it as a regular Flax Module and refer to the Flax documentation for all matter related to general usage and behavior.
Finally, this model supports inherent JAX features such as:
__call__
< source >( input_ids attention_mask = None position_ids = None params: dict = None past_key_values: dict = None dropout_rng: PRNGKey = None train: bool = False output_attentions: Optional = None output_hidden_states: Optional = None return_dict: Optional = None ) β transformers.modeling_flax_outputs.FlaxMaskedLMOutput or tuple(torch.FloatTensor)
Parameters
- input_ids (
numpy.ndarray
of shape(batch_size, input_ids_length)
) —input_ids_length
=sequence_length
. Indices of input sequence tokens in the vocabulary.Indices can be obtained using AutoTokenizer. See PreTrainedTokenizer.encode() and PreTrainedTokenizer.call() for details.
- attention_mask (
numpy.ndarray
of shape(batch_size, sequence_length)
, optional) — Mask to avoid performing attention on padding token indices. Mask values selected in[0, 1]
:- 1 for tokens that are not masked,
- 0 for tokens that are masked.
- position_ids (
numpy.ndarray
of shape(batch_size, sequence_length)
, optional) — Indices of positions of each input sequence tokens in the position embeddings. Selected in the range[0, config.max_position_embeddings - 1]
. - past_key_values (
Dict[str, np.ndarray]
, optional, returned byinit_cache
or when passing previouspast_key_values
) — Dictionary of pre-computed hidden-states (key and values in the attention blocks) that can be used for fast auto-regressive decoding. Pre-computed key and value hidden-states are of shape [batch_size, max_length]. - output_attentions (
bool
, optional) — Whether or not to return the attentions tensors of all attention layers. Seeattentions
under returned tensors for more detail. - output_hidden_states (
bool
, optional) — Whether or not to return the hidden states of all layers. Seehidden_states
under returned tensors for more detail. - return_dict (
bool
, optional) — Whether or not to return a ModelOutput instead of a plain tuple.
Returns
transformers.modeling_flax_outputs.FlaxMaskedLMOutput or tuple(torch.FloatTensor)
A transformers.modeling_flax_outputs.FlaxMaskedLMOutput or a tuple of
torch.FloatTensor
(if return_dict=False
is passed or when config.return_dict=False
) comprising various
elements depending on the configuration (GPTNeoConfig) and inputs.
-
logits (
jnp.ndarray
of shape(batch_size, sequence_length, config.vocab_size)
) β Prediction scores of the language modeling head (scores for each vocabulary token before SoftMax). -
hidden_states (
tuple(jnp.ndarray)
, optional, returned whenoutput_hidden_states=True
is passed or whenconfig.output_hidden_states=True
) β Tuple ofjnp.ndarray
(one for the output of the embeddings + one for the output of each layer) of shape(batch_size, sequence_length, hidden_size)
.Hidden-states of the model at the output of each layer plus the initial embedding outputs.
-
attentions (
tuple(jnp.ndarray)
, optional, returned whenoutput_attentions=True
is passed or whenconfig.output_attentions=True
) β Tuple ofjnp.ndarray
(one for each layer) of shape(batch_size, num_heads, sequence_length, sequence_length)
.Attentions weights after the attention softmax, used to compute the weighted average in the self-attention heads.
The FlaxGPTNeoPreTrainedModel
forward method, overrides the __call__
special method.
Although the recipe for forward pass needs to be defined within this function, one should call the Module
instance afterwards instead of this since the former takes care of running the pre and post processing steps while
the latter silently ignores them.
Example:
>>> from transformers import AutoTokenizer, FlaxGPTNeoForCausalLM
>>> tokenizer = AutoTokenizer.from_pretrained("EleutherAI/gpt-neo-1.3B")
>>> model = FlaxGPTNeoForCausalLM.from_pretrained("EleutherAI/gpt-neo-1.3B")
>>> inputs = tokenizer("Hello, my dog is cute", return_tensors="np")
>>> outputs = model(**inputs)
>>> # retrieve logts for next token
>>> next_token_logits = outputs.logits[:, -1]