Model Card for udever-bloom
udever-bloom-560m
is finetuned from bigscience/bloom-560m via BitFit on MS MARCO Passage Ranking, SNLI and MultiNLI data.
It is a universal embedding model across tasks, natural and programming languages.
(From the technical view, udever
is merely with some minor improvements to sgpt-bloom
)
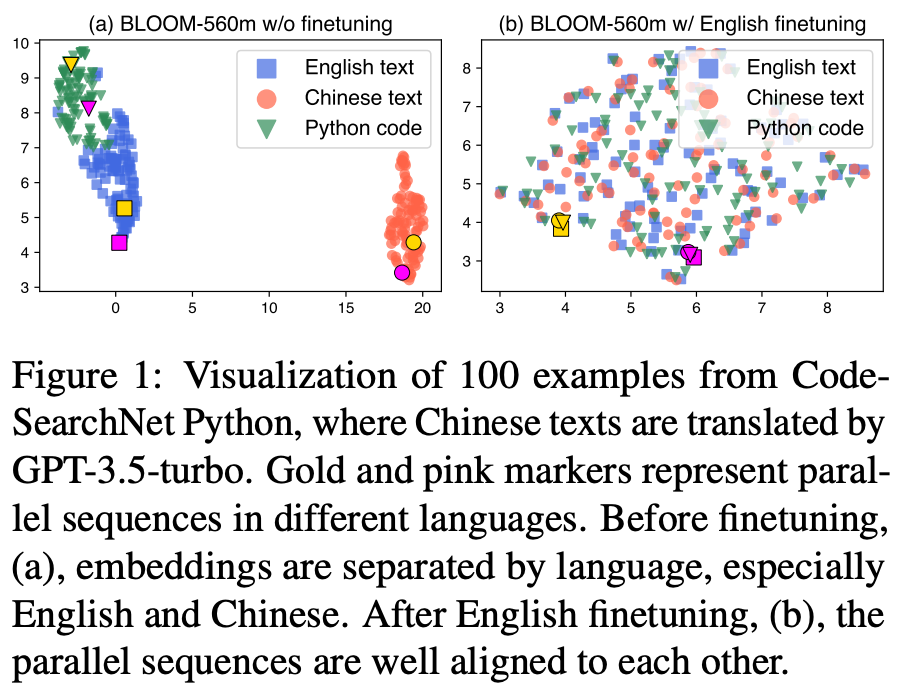
Model Details
Model Description
- Developed by: Alibaba Group
- Model type: Transformer-based Language Model (decoder-only)
- Language(s) (NLP): Multiple; see bloom training data
- Finetuned from model : bigscience/bloom-560m
Model Sources
- Repository: github.com/izhx/uni-rep
- Paper : Language Models are Universal Embedders
- Training Date : 2023-06
Checkpoints
On ModelScope / 魔搭社区: udever-bloom-560m, udever-bloom-1b1, udever-bloom-3b, udever-bloom-7b1
How to Get Started with the Model
Use the code below to get started with the model.
import torch
from transformers import AutoTokenizer, BloomModel
tokenizer = AutoTokenizer.from_pretrained('izhx/udever-bloom-560m')
model = BloomModel.from_pretrained('izhx/udever-bloom-560m')
boq, eoq, bod, eod = '[BOQ]', '[EOQ]', '[BOD]', '[EOD]'
eoq_id, eod_id = tokenizer.convert_tokens_to_ids([eoq, eod])
if tokenizer.padding_side != 'left':
print('!!!', tokenizer.padding_side)
tokenizer.padding_side = 'left'
def encode(texts: list, is_query: bool = True, max_length=300):
bos = boq if is_query else bod
eos_id = eoq_id if is_query else eod_id
texts = [bos + t for t in texts]
encoding = tokenizer(
texts, truncation=True, max_length=max_length - 1, padding=True
)
for ids, mask in zip(encoding['input_ids'], encoding['attention_mask']):
ids.append(eos_id)
mask.append(1)
inputs = tokenizer.pad(encoding, return_tensors='pt')
with torch.inference_mode():
outputs = model(**inputs)
embeds = outputs.last_hidden_state[:, -1]
return embeds
encode(['I am Bert', 'You are Elmo'])
Training Details
Training Data
- MS MARCO Passage Ranking, retrieved by (https://github.com/UKPLab/sentence-transformers/blob/master/examples/training/ms_marco/train_bi-encoder_mnrl.py#L86)
- SNLI and MultiNLI (https://sbert.net/datasets/AllNLI.tsv.gz)
Training Procedure
Preprocessing
MS MARCO hard negatives provided by (https://github.com/UKPLab/sentence-transformers/blob/master/examples/training/ms_marco/train_bi-encoder_mnrl.py#L86). Negatives for SNLI and MultiNLI are randomly sampled.
Training Hyperparameters
- Training regime: tf32, BitFit
- Batch size: 1024
- Epochs: 3
- Optimizer: AdamW
- Learning rate: 1e-4
- Scheduler: constant with warmup.
- Warmup: 0.25 epoch
Evaluation
Table 1: Massive Text Embedding Benchmark MTEB
MTEB | Avg. | Class. | Clust. | PairClass. | Rerank. | Retr. | STS | Summ. |
---|---|---|---|---|---|---|---|---|
#Datasets ➡️ | 56 | 12 | 11 | 3 | 4 | 15 | 10 | 1 |
bge-large-en-v1.5 | 64.23 | 75.97 | 46.08 | 87.12 | 60.03 | 54.29 | 83.11 | 31.61 |
bge-base-en-v1.5 | 63.55 | 75.53 | 45.77 | 86.55 | 58.86 | 53.25 | 82.4 | 31.07 |
gte-large | 63.13 | 73.33 | 46.84 | 85 | 59.13 | 52.22 | 83.35 | 31.66 |
gte-base | 62.39 | 73.01 | 46.2 | 84.57 | 58.61 | 51.14 | 82.3 | 31.17 |
e5-large-v2 | 62.25 | 75.24 | 44.49 | 86.03 | 56.61 | 50.56 | 82.05 | 30.19 |
instructor-xl | 61.79 | 73.12 | 44.74 | 86.62 | 57.29 | 49.26 | 83.06 | 32.32 |
instructor-large | 61.59 | 73.86 | 45.29 | 85.89 | 57.54 | 47.57 | 83.15 | 31.84 |
e5-base-v2 | 61.5 | 73.84 | 43.8 | 85.73 | 55.91 | 50.29 | 81.05 | 30.28 |
e5-large | 61.42 | 73.14 | 43.33 | 85.94 | 56.53 | 49.99 | 82.06 | 30.97 |
text-embedding-ada-002 (OpenAI API) | 60.99 | 70.93 | 45.9 | 84.89 | 56.32 | 49.25 | 80.97 | 30.8 |
e5-base | 60.44 | 72.63 | 42.11 | 85.09 | 55.7 | 48.75 | 80.96 | 31.01 |
SGPT-5.8B-msmarco | 58.93 | 68.13 | 40.34 | 82 | 56.56 | 50.25 | 78.1 | 31.46 |
sgpt-bloom-7b1-msmarco | 57.59 | 66.19 | 38.93 | 81.9 | 55.65 | 48.22 | 77.74 | 33.6 |
Udever-bloom-560m | 55.80 | 68.04 | 36.89 | 81.05 | 52.60 | 41.19 | 79.93 | 32.06 |
Udever-bloom-1b1 | 58.28 | 70.18 | 39.11 | 83.11 | 54.28 | 45.27 | 81.52 | 31.10 |
Udever-bloom-3b | 59.86 | 71.91 | 40.74 | 84.06 | 54.90 | 47.67 | 82.37 | 30.62 |
Udever-bloom-7b1 | 60.63 | 72.13 | 40.81 | 85.40 | 55.91 | 49.34 | 83.01 | 30.97 |
Table 2: CodeSearchNet
CodeSearchNet | Go | Ruby | Python | Java | JS | PHP | Avg. |
---|---|---|---|---|---|---|---|
CodeBERT | 69.3 | 70.6 | 84.0 | 86.8 | 74.8 | 70.6 | 76.0 |
GraphCodeBERT | 84.1 | 73.2 | 87.9 | 75.7 | 71.1 | 72.5 | 77.4 |
cpt-code S | 97.7 | 86.3 | 99.8 | 94.0 | 86.0 | 96.7 | 93.4 |
cpt-code M | 97.5 | 85.5 | 99.9 | 94.4 | 86.5 | 97.2 | 93.5 |
sgpt-bloom-7b1-msmarco | 76.79 | 69.25 | 95.68 | 77.93 | 70.35 | 73.45 | 77.24 |
Udever-bloom-560m | 75.38 | 66.67 | 96.23 | 78.99 | 69.39 | 73.69 | 76.73 |
Udever-bloom-1b1 | 78.76 | 72.85 | 97.67 | 82.77 | 74.38 | 78.97 | 80.90 |
Udever-bloom-3b | 80.63 | 75.40 | 98.02 | 83.88 | 76.18 | 79.67 | 82.29 |
Udever-bloom-7b1 | 79.37 | 76.59 | 98.38 | 84.68 | 77.49 | 80.03 | 82.76 |
Table 3: Chinese multi-domain retrieval Multi-cpr
E-commerce | Entertainment video | Medical | ||||||
---|---|---|---|---|---|---|---|---|
Model | Train | Backbone | MRR@10 | Recall@1k | MRR@10 | Recall@1k | MRR@10 | Recall@1k |
BM25 | - | - | 0.225 | 0.815 | 0.225 | 0.780 | 0.187 | 0.482 |
Doc2Query | - | - | 0.239 | 0.826 | 0.238 | 0.794 | 0.210 | 0.505 |
DPR-1 | In-Domain | BERT | 0.270 | 0.921 | 0.254 | 0.934 | 0.327 | 0.747 |
DPR-2 | In-Domain | BERT-CT | 0.289 | 0.926 | 0.263 | 0.935 | 0.339 | 0.769 |
text-embedding-ada-002 | General | GPT | 0.183 | 0.825 | 0.159 | 0.786 | 0.245 | 0.593 |
sgpt-bloom-7b1-msmarco | General | BLOOM | 0.242 | 0.840 | 0.227 | 0.829 | 0.311 | 0.675 |
Udever-bloom-560m | General | BLOOM | 0.156 | 0.802 | 0.149 | 0.749 | 0.245 | 0.571 |
Udever-bloom-1b1 | General | BLOOM | 0.244 | 0.863 | 0.208 | 0.815 | 0.241 | 0.557 |
Udever-bloom-3b | General | BLOOM | 0.267 | 0.871 | 0.228 | 0.836 | 0.288 | 0.619 |
Udever-bloom-7b1 | General | BLOOM | 0.296 | 0.889 | 0.267 | 0.907 | 0.343 | 0.705 |
More results refer to paper section 3.
Technical Specifications
Model Architecture and Objective
- Model: bigscience/bloom-560m.
- Objective: Constrastive loss with hard negatives (refer to paper section 2.2).
Compute Infrastructure
- Nvidia A100 SXM4 80GB.
- torch 2.0.0, transformers 4.29.2.
Citation
BibTeX:
@article{zhang2023language,
title={Language Models are Universal Embedders},
author={Zhang, Xin and Li, Zehan and Zhang, Yanzhao and Long, Dingkun and Xie, Pengjun and Zhang, Meishan and Zhang, Min},
journal={arXiv preprint arXiv:2310.08232},
year={2023}
}
- Downloads last month
- 1,078
This model does not have enough activity to be deployed to Inference API (serverless) yet. Increase its social
visibility and check back later, or deploy to Inference Endpoints (dedicated)
instead.
Spaces using izhx/udever-bloom-560m 6
Evaluation results
- cos_sim_pearson on MTEB AFQMCvalidation set self-reported25.170
- cos_sim_spearman on MTEB AFQMCvalidation set self-reported25.320
- euclidean_pearson on MTEB AFQMCvalidation set self-reported25.343
- euclidean_spearman on MTEB AFQMCvalidation set self-reported25.528
- manhattan_pearson on MTEB AFQMCvalidation set self-reported25.734
- manhattan_spearman on MTEB AFQMCvalidation set self-reported25.922
- cos_sim_pearson on MTEB ATECtest set self-reported32.336
- cos_sim_spearman on MTEB ATECtest set self-reported33.458
- euclidean_pearson on MTEB ATECtest set self-reported35.147
- euclidean_spearman on MTEB ATECtest set self-reported33.378