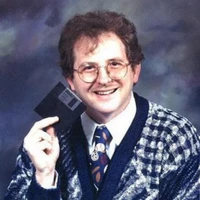
language:
- multilingual
- pt
- en
tags:
- bert-base-multilingual-cased
- semantic role labeling
- finetuned
license: apache-2.0
datasets:
- PropBank.Br
- CoNLL-2012
metrics:
- F1 Measure
mBERT base fine-tune in English and Portuguese semantic role labeling
Model description
This model is the bert-base-multilingual-cased
fine-tuned first on the English CoNLL formatted OntoNotes v5.0 semantic role labeling data and then fine-tuned on the PropBank.Br data. This is part of a project from which resulted the following models:
- liaad/srl-pt_bertimbau-base
- liaad/srl-pt_bertimbau-large
- liaad/srl-pt_xlmr-base
- liaad/srl-pt_xlmr-large
- liaad/srl-pt_mbert-base
- liaad/srl-en_xlmr-base
- liaad/srl-en_xlmr-large
- liaad/srl-en_mbert-base
- liaad/srl-enpt_xlmr-base
- liaad/srl-enpt_xlmr-large
- liaad/srl-enpt_mbert-base
- liaad/ud_srl-pt_bertimbau-large
- liaad/ud_srl-pt_xlmr-large
- liaad/ud_srl-enpt_xlmr-large
For more information, please see the accompanying article (See BibTeX entry and citation info below) and the project's github.
Intended uses & limitations
How to use
To use the transformers portion of this model:
from transformers import AutoTokenizer, AutoModel
tokenizer = AutoTokenizer.from_pretrained("liaad/srl-enpt_mbert-base")
model = AutoModel.from_pretrained("liaad/srl-enpt_mbert-base")
To use the full SRL model (transformers portion + a decoding layer), refer to the project's github.
Limitations and bias
- The English data was preprocessed to match the Portuguese data, so there are some differences in role attributions and some roles were removed from the data.
Training procedure
The model was first fine-tuned on the CoNLL-2012 dataset, preprocessed to match the Portuguese PropBank.Br data; then it was fine-tuned in the PropBank.Br dataset using 10-fold Cross-Validation. The resulting models were tested on the folds as well as on a smaller opinion dataset "Buscapé". For more information, please see the accompanying article (See BibTeX entry and citation info below) and the project's github.
Eval results
Model Name | F1 CV PropBank.Br (in domain) | F1 Buscapé (out of domain) |
---|---|---|
srl-pt_bertimbau-base |
76.30 | 73.33 |
srl-pt_bertimbau-large |
77.42 | 74.85 |
srl-pt_xlmr-base |
75.22 | 72.82 |
srl-pt_xlmr-large |
77.59 | 73.84 |
srl-pt_mbert-base |
72.76 | 66.89 |
srl-en_xlmr-base |
66.59 | 65.24 |
srl-en_xlmr-large |
67.60 | 64.94 |
srl-en_mbert-base |
63.07 | 58.56 |
srl-enpt_xlmr-base |
76.50 | 73.74 |
srl-enpt_xlmr-large |
78.22 | 74.55 |
srl-enpt_mbert-base |
74.88 | 69.19 |
ud_srl-pt_bertimbau-large |
77.53 | 74.49 |
ud_srl-pt_xlmr-large |
77.69 | 74.91 |
ud_srl-enpt_xlmr-large |
77.97 | 75.05 |
BibTeX entry and citation info
@misc{oliveira2021transformers,
title={Transformers and Transfer Learning for Improving Portuguese Semantic Role Labeling},
author={Sofia Oliveira and Daniel Loureiro and Alípio Jorge},
year={2021},
eprint={2101.01213},
archivePrefix={arXiv},
primaryClass={cs.CL}
}