File size: 4,069 Bytes
564d640 724da95 60fdf15 564d640 724da95 d7b87f3 564d640 d7b87f3 e9db55f 564d640 2d36291 564d640 2e03b73 8088372 e19041c 8b5a5bd 564d640 e9db55f 7aa3560 e9db55f 8b5a5bd 7aa3560 564d640 e9db55f 564d640 b99cd90 e19041c |
1 2 3 4 5 6 7 8 9 10 11 12 13 14 15 16 17 18 19 20 21 22 23 24 25 26 27 28 29 30 31 32 33 34 35 36 37 38 39 40 41 |
---
base_model:
- sometimesanotion/Lamarck-14B-v0.6
- deepseek-ai/DeepSeek-R1-Distill-Qwen-14B
- sometimesanotion/Lamarck-14B-v0.3
- sometimesanotion/Qwenvergence-14B-v9
- sometimesanotion/Qwenvergence-14B-v3-Prose
- arcee-ai/Virtuoso-Small
library_name: transformers
tags:
- mergekit
- merge
license: apache-2.0
language:
- en
pipeline_tag: text-generation
metrics:
- accuracy
---

---
> [!TIP] With no regressions, mostly gains over the previous release, this version of Lamarck has [broken the 41.0 average](https://shorturl.at/jUqEk) maximum for 14B parameter models. As of this writing, Lamarck v0.7 ranks #8 among models under 70B parameters on the Open LLM Leaderboard. Given the quality models in the 32B range, I think Lamarck deserves his shades. A little layer analysis of a model in the 14B range goes a long, long way.
> [!TIP] The first DPO finetune of Lamarck has appeared! Check out [jpacifico/Chocolatine-2-14B-Instruct-v2.0b3](http://huggingface.co/jpacifico/Chocolatine-2-14B-Instruct-v2.0b3), whose notes say, "The Chocolatine model series is a quick demonstration that a base model can be easily fine-tuned to achieve compelling performance." Lamarck's painstaking merge process was intended to make finetuning to a desired polish as easy and energy-efficient as possible. Thank you, @jpacifico!
Lamarck 14B v0.7: A generalist merge with emphasis on multi-step reasoning, prose, and multi-language ability. The 14B parameter model class has a lot of strong performers, and Lamarck strives to be well-rounded and solid: 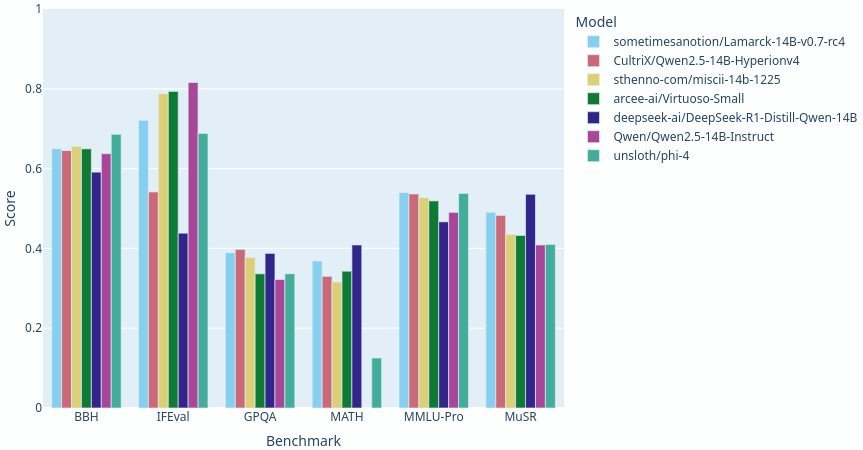
Lamarck is produced by a custom toolchain to automate a complex sequences of LoRAs and various layer-targeting merges:
- **Extracted LoRA adapters from special-purpose merges**
- **Custom base models and model_stocks, with LoRAs from from [huihui-ai/Qwen2.5-14B-Instruct-abliterated-v2](https://huggingface.co/huihui-ai/Qwen2.5-14B-Instruct-abliterated-v2) to minimize IFEVAL loss often seen in model_stock merges**
- **Separate branches for aggressive breadcrumbs and conservative DELLA merges**
- **Highly targeted weight/density gradients for every 2-4 layers, at each stage**
- **Finalization through SLERP+TIES merges recombining the breadcrumbs and DELLA branches to taste**
Lamarck's performance comes from an ancestry that goes back through careful merges to select finetuning work, upcycled and combined. Through intermediate merges, [arcee-ai/Virtuoso-Small](https://huggingface.co/arcee-ai/Virtuoso-Small) [sthenno-com/miscii-14b-1225](https://huggingface.co/sthenno-com/miscii-14b-1225) and [VAGOsolutions/SauerkrautLM-v2-14b-DPO](https://huggingface.co/VAGOsolutions/SauerkrautLM-v2-14b-DPO) are emphasized in early layers for extra BBH; later layers add synergistic influence from [deepseek-ai/DeepSeek-R1-Distill-Qwen-14B](https://huggingface.co/deepseek-ai/DeepSeek-R1-Distill-Qwen-14B), [Krystalan/DRT-o1-14B](https://huggingface.co/Krystalan/DRT-o1-14B), [EVA-UNIT-01/EVA-Qwen2.5-14B-v0.2](https://huggingface.co/EVA-UNIT-01/EVA-Qwen2.5-14B-v0.2), and [CultriX/Qwen2.5-14B-Wernicke](https://huggingface.co/CultriX/Qwen2.5-14B-Wernicke).
More subjectively, its prose and translation abilities are boosted by repeated re-emphasis of [Krystalan/DRT-o1-14B](https://huggingface.co/Krystalan/DRT-o1-14B) and [underwoods/medius-erebus-magnum-14b](https://huggingface.co/underwoods/medius-erebus-magnum-14b). Other models found in [sometimesanotion/Qwenvergence-14B-v3-Prose](https://huggingface/sometimesanotion/Qwenvergence-14B-v3-Prose) have their impact on prose quality - and surprising synergy of reasoning.
Kudos to @arcee-ai, @deepseek-ai, @Krystalan, @underwoods, @VAGOSolutions, @CultriX, @sthenno-com, and @rombodawg whose models had the most influence. [Vimarckoso v3](https://huggingface.co/sometimesanotion/Qwen2.5-14B-Vimarckoso-v3) has the model card which documents its extended lineage. |