A newer version of the Gradio SDK is available:
5.5.0
BigVGAN: A Universal Neural Vocoder with Large-Scale Training
Sang-gil Lee, Wei Ping, Boris Ginsburg, Bryan Catanzaro, Sungroh Yoon
[Paper] - [Code] - [Showcase] - [Project Page] - [Weights] - [Demo]
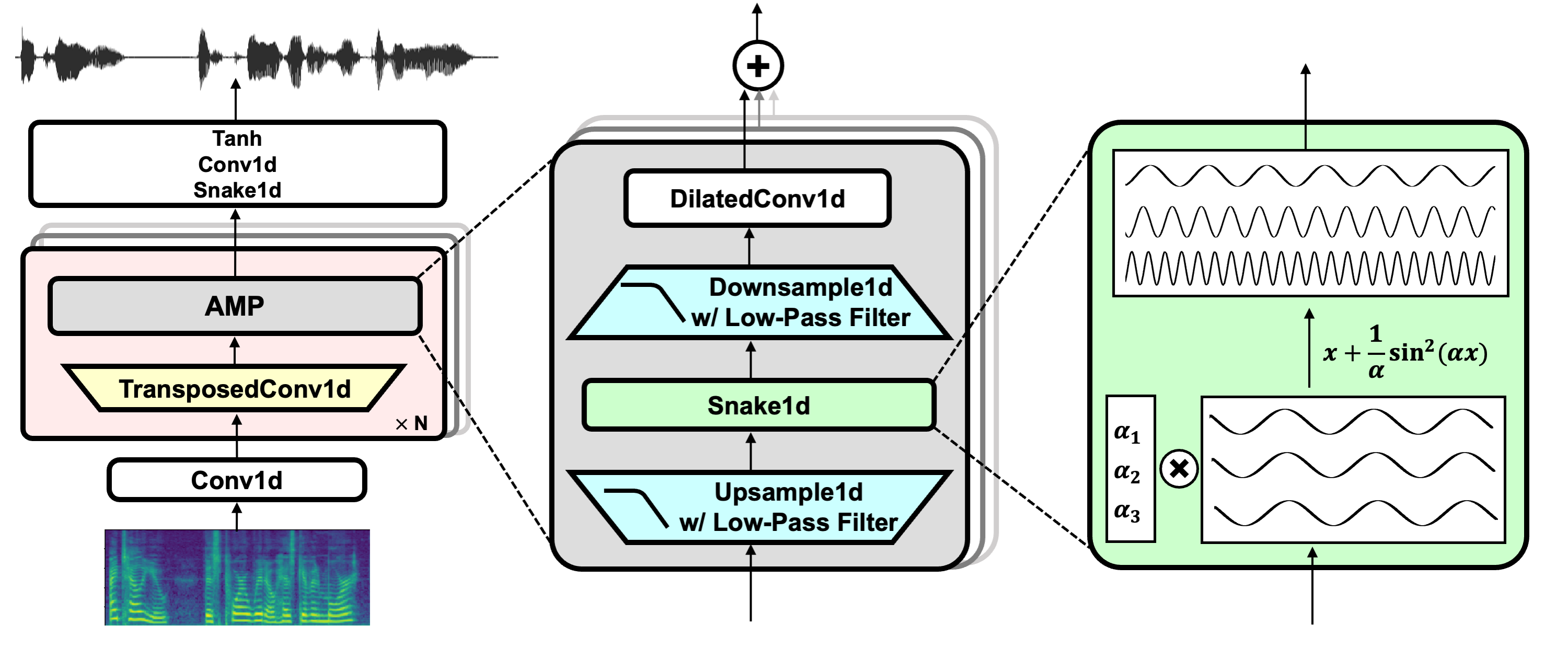
News
Jul 2024 (v2.3):
- General refactor and code improvements for improved readability.
- Fully fused CUDA kernel of anti-alised activation (upsampling + activation + downsampling) with inference speed benchmark.
Jul 2024 (v2.2): The repository now includes an interactive local demo using gradio.
Jul 2024 (v2.1): BigVGAN is now integrated with π€ Hugging Face Hub with easy access to inference using pretrained checkpoints. We also provide an interactive demo on Hugging Face Spaces.
Jul 2024 (v2): We release BigVGAN-v2 along with pretrained checkpoints. Below are the highlights:
- Custom CUDA kernel for inference: we provide a fused upsampling + activation kernel written in CUDA for accelerated inference speed. Our test shows 1.5 - 3x faster speed on a single A100 GPU.
- Improved discriminator and loss: BigVGAN-v2 is trained using a multi-scale sub-band CQT discriminator and a multi-scale mel spectrogram loss.
- Larger training data: BigVGAN-v2 is trained using datasets containing diverse audio types, including speech in multiple languages, environmental sounds, and instruments.
- We provide pretrained checkpoints of BigVGAN-v2 using diverse audio configurations, supporting up to 44 kHz sampling rate and 512x upsampling ratio.
Installation
The codebase has been tested on Python 3.10
and PyTorch 2.3.1
conda packages with either pytorch-cuda=12.1
or pytorch-cuda=11.8
. Below is an example command to create the conda environment:
conda create -n bigvgan python=3.10 pytorch torchvision torchaudio pytorch-cuda=12.1 -c pytorch -c nvidia
conda activate bigvgan
Clone the repository and install dependencies:
git clone https://github.com/NVIDIA/BigVGAN
cd BigVGAN
pip install -r requirements.txt
Inference Quickstart using π€ Hugging Face Hub
Below example describes how you can use BigVGAN: load the pretrained BigVGAN generator from Hugging Face Hub, compute mel spectrogram from input waveform, and generate synthesized waveform using the mel spectrogram as the model's input.
device = 'cuda'
import torch
import bigvgan
import librosa
from meldataset import get_mel_spectrogram
# instantiate the model. You can optionally set use_cuda_kernel=True for faster inference.
model = bigvgan.BigVGAN.from_pretrained('nvidia/bigvgan_v2_24khz_100band_256x', use_cuda_kernel=False)
# remove weight norm in the model and set to eval mode
model.remove_weight_norm()
model = model.eval().to(device)
# load wav file and compute mel spectrogram
wav_path = '/path/to/your/audio.wav'
wav, sr = librosa.load(wav_path, sr=model.h.sampling_rate, mono=True) # wav is np.ndarray with shape [T_time] and values in [-1, 1]
wav = torch.FloatTensor(wav).unsqueeze(0) # wav is FloatTensor with shape [B(1), T_time]
# compute mel spectrogram from the ground truth audio
mel = get_mel_spectrogram(wav, model.h).to(device) # mel is FloatTensor with shape [B(1), C_mel, T_frame]
# generate waveform from mel
with torch.inference_mode():
wav_gen = model(mel) # wav_gen is FloatTensor with shape [B(1), 1, T_time] and values in [-1, 1]
wav_gen_float = wav_gen.squeeze(0).cpu() # wav_gen is FloatTensor with shape [1, T_time]
# you can convert the generated waveform to 16 bit linear PCM
wav_gen_int16 = (wav_gen_float * 32767.0).numpy().astype('int16') # wav_gen is now np.ndarray with shape [1, T_time] and int16 dtype
Local gradio demo
You can run a local gradio demo using below command:
pip install -r demo/requirements.txt
python demo/app.py
Training
Create symbolic link to the root of the dataset. The codebase uses filelist with the relative path from the dataset. Below are the example commands for LibriTTS dataset:
cd filelists/LibriTTS && \
ln -s /path/to/your/LibriTTS/train-clean-100 train-clean-100 && \
ln -s /path/to/your/LibriTTS/train-clean-360 train-clean-360 && \
ln -s /path/to/your/LibriTTS/train-other-500 train-other-500 && \
ln -s /path/to/your/LibriTTS/dev-clean dev-clean && \
ln -s /path/to/your/LibriTTS/dev-other dev-other && \
ln -s /path/to/your/LibriTTS/test-clean test-clean && \
ln -s /path/to/your/LibriTTS/test-other test-other && \
cd ../..
Train BigVGAN model. Below is an example command for training BigVGAN-v2 using LibriTTS dataset at 24kHz with a full 100-band mel spectrogram as input:
python train.py \
--config configs/bigvgan_v2_24khz_100band_256x.json \
--input_wavs_dir filelists/LibriTTS \
--input_training_file filelists/LibriTTS/train-full.txt \
--input_validation_file filelists/LibriTTS/val-full.txt \
--list_input_unseen_wavs_dir filelists/LibriTTS filelists/LibriTTS \
--list_input_unseen_validation_file filelists/LibriTTS/dev-clean.txt filelists/LibriTTS/dev-other.txt \
--checkpoint_path exp/bigvgan_v2_24khz_100band_256x
Synthesis
Synthesize from BigVGAN model. Below is an example command for generating audio from the model.
It computes mel spectrograms using wav files from --input_wavs_dir
and saves the generated audio to --output_dir
.
python inference.py \
--checkpoint_file /path/to/your/bigvgan_v2_24khz_100band_256x/bigvgan_generator.pt \
--input_wavs_dir /path/to/your/input_wav \
--output_dir /path/to/your/output_wav
inference_e2e.py
supports synthesis directly from the mel spectrogram saved in .npy
format, with shapes [1, channel, frame]
or [channel, frame]
.
It loads mel spectrograms from --input_mels_dir
and saves the generated audio to --output_dir
.
Make sure that the STFT hyperparameters for mel spectrogram are the same as the model, which are defined in config.json
of the corresponding model.
python inference_e2e.py \
--checkpoint_file /path/to/your/bigvgan_v2_24khz_100band_256x/bigvgan_generator.pt \
--input_mels_dir /path/to/your/input_mel \
--output_dir /path/to/your/output_wav
Using Custom CUDA Kernel for Synthesis
You can apply the fast CUDA inference kernel by using a parameter use_cuda_kernel
when instantiating BigVGAN:
generator = BigVGAN(h, use_cuda_kernel=True)
You can also pass --use_cuda_kernel
to inference.py
and inference_e2e.py
to enable this feature.
When applied for the first time, it builds the kernel using nvcc
and ninja
. If the build succeeds, the kernel is saved to alias_free_activation/cuda/build
and the model automatically loads the kernel. The codebase has been tested using CUDA 12.1
.
Please make sure that both are installed in your system and nvcc
installed in your system matches the version your PyTorch build is using.
We recommend running test_cuda_vs_torch_model.py
first to build and check the correctness of the CUDA kernel. See below example command and its output, where it returns [Success] test CUDA fused vs. plain torch BigVGAN inference
:
python tests/test_cuda_vs_torch_model.py \
--checkpoint_file /path/to/your/bigvgan_generator.pt
loading plain Pytorch BigVGAN
...
loading CUDA kernel BigVGAN with auto-build
Detected CUDA files, patching ldflags
Emitting ninja build file /path/to/your/BigVGAN/alias_free_activation/cuda/build/build.ninja..
Building extension module anti_alias_activation_cuda...
...
Loading extension module anti_alias_activation_cuda...
...
Loading '/path/to/your/bigvgan_generator.pt'
...
[Success] test CUDA fused vs. plain torch BigVGAN inference
> mean_difference=0.0007238413265440613
...
If you see [Fail] test CUDA fused vs. plain torch BigVGAN inference
, it means that the CUDA kernel inference is incorrect. Please check if nvcc
installed in your system is compatible with your PyTorch version.
Pretrained Models
We provide the pretrained models on Hugging Face Collections.
One can download the checkpoints of the generator weight (named bigvgan_generator.pt
) and its discriminator/optimizer states (named bigvgan_discriminator_optimizer.pt
) within the listed model repositories.
Model Name | Sampling Rate | Mel band | fmax | Upsampling Ratio | Params | Dataset | Steps | Fine-Tuned |
---|---|---|---|---|---|---|---|---|
bigvgan_v2_44khz_128band_512x | 44 kHz | 128 | 22050 | 512 | 122M | Large-scale Compilation | 3M | No |
bigvgan_v2_44khz_128band_256x | 44 kHz | 128 | 22050 | 256 | 112M | Large-scale Compilation | 3M | No |
bigvgan_v2_24khz_100band_256x | 24 kHz | 100 | 12000 | 256 | 112M | Large-scale Compilation | 3M | No |
bigvgan_v2_22khz_80band_256x | 22 kHz | 80 | 11025 | 256 | 112M | Large-scale Compilation | 3M | No |
bigvgan_v2_22khz_80band_fmax8k_256x | 22 kHz | 80 | 8000 | 256 | 112M | Large-scale Compilation | 3M | No |
bigvgan_24khz_100band | 24 kHz | 100 | 12000 | 256 | 112M | LibriTTS | 5M | No |
bigvgan_base_24khz_100band | 24 kHz | 100 | 12000 | 256 | 14M | LibriTTS | 5M | No |
bigvgan_22khz_80band | 22 kHz | 80 | 8000 | 256 | 112M | LibriTTS + VCTK + LJSpeech | 5M | No |
bigvgan_base_22khz_80band | 22 kHz | 80 | 8000 | 256 | 14M | LibriTTS + VCTK + LJSpeech | 5M | No |
The paper results are based on the original 24kHz BigVGAN models (bigvgan_24khz_100band
and bigvgan_base_24khz_100band
) trained on LibriTTS dataset.
We also provide 22kHz BigVGAN models with band-limited setup (i.e., fmax=8000) for TTS applications.
Note that the checkpoints use snakebeta
activation with log scale parameterization, which have the best overall quality.
You can fine-tune the models by:
- downloading the checkpoints (both the generator weight and its discriminator/optimizer states)
- resuming training using your audio dataset by specifying
--checkpoint_path
that includes the checkpoints when launchingtrain.py
Training Details of BigVGAN-v2
Comapred to the original BigVGAN, the pretrained checkpoints of BigVGAN-v2 used batch_size=32
with a longer segment_size=65536
and are trained using 8 A100 GPUs.
Note that the BigVGAN-v2 json
config files in ./configs
use batch_size=4
as default to fit in a single A100 GPU for training. You can fine-tune the models adjusting batch_size
depending on your GPUs.
When training BigVGAN-v2 from scratch with small batch size, it can potentially encounter the early divergence problem mentioned in the paper. In such case, we recommend lowering the clip_grad_norm
value (e.g. 100
) for the early training iterations (e.g. 20000 steps) and increase the value to the default 500
.
Evaluation Results of BigVGAN-v2
Below are the objective results of the 24kHz model (bigvgan_v2_24khz_100band_256x
) obtained from the LibriTTS dev
sets. BigVGAN-v2 shows noticeable improvements of the metrics. The model also exhibits reduced perceptual artifacts, especially for non-speech audio.
Model | Dataset | Steps | PESQ(β) | M-STFT(β) | MCD(β) | Periodicity(β) | V/UV F1(β) |
---|---|---|---|---|---|---|---|
BigVGAN | LibriTTS | 1M | 4.027 | 0.7997 | 0.3745 | 0.1018 | 0.9598 |
BigVGAN | LibriTTS | 5M | 4.256 | 0.7409 | 0.2988 | 0.0809 | 0.9698 |
BigVGAN-v2 | Large-scale Compilation | 3M | 4.359 | 0.7134 | 0.3060 | 0.0621 | 0.9777 |
Speed Benchmark
Below are the speed and VRAM usage benchmark results of BigVGAN from tests/test_cuda_vs_torch_model.py
, using bigvgan_v2_24khz_100band_256x
as a reference model.
GPU | num_mel_frame | use_cuda_kernel | Speed (kHz) | Real-time Factor | VRAM (GB) |
---|---|---|---|---|---|
NVIDIA A100 | 256 | False | 1672.1 | 69.7x | 1.3 |
True | 3916.5 | 163.2x | 1.3 | ||
2048 | False | 1899.6 | 79.2x | 1.7 | |
True | 5330.1 | 222.1x | 1.7 | ||
16384 | False | 1973.8 | 82.2x | 5.0 | |
True | 5761.7 | 240.1x | 4.4 | ||
NVIDIA GeForce RTX 3080 | 256 | False | 841.1 | 35.0x | 1.3 |
True | 1598.1 | 66.6x | 1.3 | ||
2048 | False | 929.9 | 38.7x | 1.7 | |
True | 1971.3 | 82.1x | 1.6 | ||
16384 | False | 943.4 | 39.3x | 5.0 | |
True | 2026.5 | 84.4x | 3.9 | ||
NVIDIA GeForce RTX 2080 Ti | 256 | False | 515.6 | 21.5x | 1.3 |
True | 811.3 | 33.8x | 1.3 | ||
2048 | False | 576.5 | 24.0x | 1.7 | |
True | 1023.0 | 42.6x | 1.5 | ||
16384 | False | 589.4 | 24.6x | 5.0 | |
True | 1068.1 | 44.5x | 3.2 |
Acknowledgements
We thank Vijay Anand Korthikanti and Kevin J. Shih for their generous support in implementing the CUDA kernel for inference.
References
- HiFi-GAN (for generator and multi-period discriminator)
- Snake (for periodic activation)
- Alias-free-torch (for anti-aliasing)
- Julius (for low-pass filter)
- UnivNet (for multi-resolution discriminator)
- descript-audio-codec and vocos (for multi-band multi-scale STFT discriminator and multi-scale mel spectrogram loss)
- Amphion (for multi-scale sub-band CQT discriminator)