hubert-base-ch-speech-emotion-recognition
This model uses [TencentGameMate/chinese-hubert-base](TencentGameMate/chinese-hubert-base Β· Hugging Face) as the pre-training model for training on the CASIA dataset.
The CASIA dataset provides 1200 samples of recordings from actor performing on 6 different emotions in Chinese(The official website provides a total of 9600 pieces of data, and the data set I used may not be complete), which are:
emotions = ['anger', 'fear', 'happy', 'neutral', 'sad', 'surprise']
Usage
import os
import random
import librosa
import torch
import torch.nn as nn
import torch.nn.functional as F
from transformers import AutoConfig, Wav2Vec2FeatureExtractor, HubertPreTrainedModel, HubertModel
model_name_or_path = "xmj2002/hubert-base-ch-speech-emotion-recognition"
duration = 6
sample_rate = 16000
config = AutoConfig.from_pretrained(
pretrained_model_name_or_path=model_name_or_path,
)
def id2class(id):
if id == 0:
return "angry"
elif id == 1:
return "fear"
elif id == 2:
return "happy"
elif id == 3:
return "neutral"
elif id == 4:
return "sad"
else:
return "surprise"
def predict(path, processor, model):
speech, sr = librosa.load(path=path, sr=sample_rate)
speech = processor(speech, padding="max_length", truncation=True, max_length=duration * sr,
return_tensors="pt", sampling_rate=sr).input_values
with torch.no_grad():
logit = model(speech)
score = F.softmax(logit, dim=1).detach().cpu().numpy()[0]
id = torch.argmax(logit).cpu().numpy()
print(f"file path: {path} \t predict: {id2class(id)} \t score:{score[id]} ")
class HubertClassificationHead(nn.Module):
def __init__(self, config):
super().__init__()
self.dense = nn.Linear(config.hidden_size, config.hidden_size)
self.dropout = nn.Dropout(config.classifier_dropout)
self.out_proj = nn.Linear(config.hidden_size, config.num_class)
def forward(self, x):
x = self.dense(x)
x = torch.tanh(x)
x = self.dropout(x)
x = self.out_proj(x)
return x
class HubertForSpeechClassification(HubertPreTrainedModel):
def __init__(self, config):
super().__init__(config)
self.hubert = HubertModel(config)
self.classifier = HubertClassificationHead(config)
self.init_weights()
def forward(self, x):
outputs = self.hubert(x)
hidden_states = outputs[0]
x = torch.mean(hidden_states, dim=1)
x = self.classifier(x)
return x
processor = Wav2Vec2FeatureExtractor.from_pretrained(model_name_or_path)
model = HubertForSpeechClassification.from_pretrained(
model_name_or_path,
config=config,
)
model.eval()
file_path = [f"test_data/{path}" for path in os.listdir("test_data")]
path = random.sample(file_path, 1)[0]
predict(path, processor, model)
Training setting
Data set segmentation ratio: training set: verification set: test set = 0.6:0.2:0.2
seed: 34
batch_size: 36
lr: 2e-4
optimizer: AdamW(betas=(0.93,0.98), weight_decay=0.2)
scheduler: Step_LR(step_size=10, gamma=0.3)
classifier dropout: 0.1
optimizer parameter:
for name, param in model.named_parameters(): if "hubert" in name: parameter.append({'params': param, 'lr': 0.2 * lr}) else: parameter.append({'params': param, "lr": lr})
Metric
Loss(test set): 0.1165
Accuracy(test set): 0.972
Accuracy curve of training set and verification set
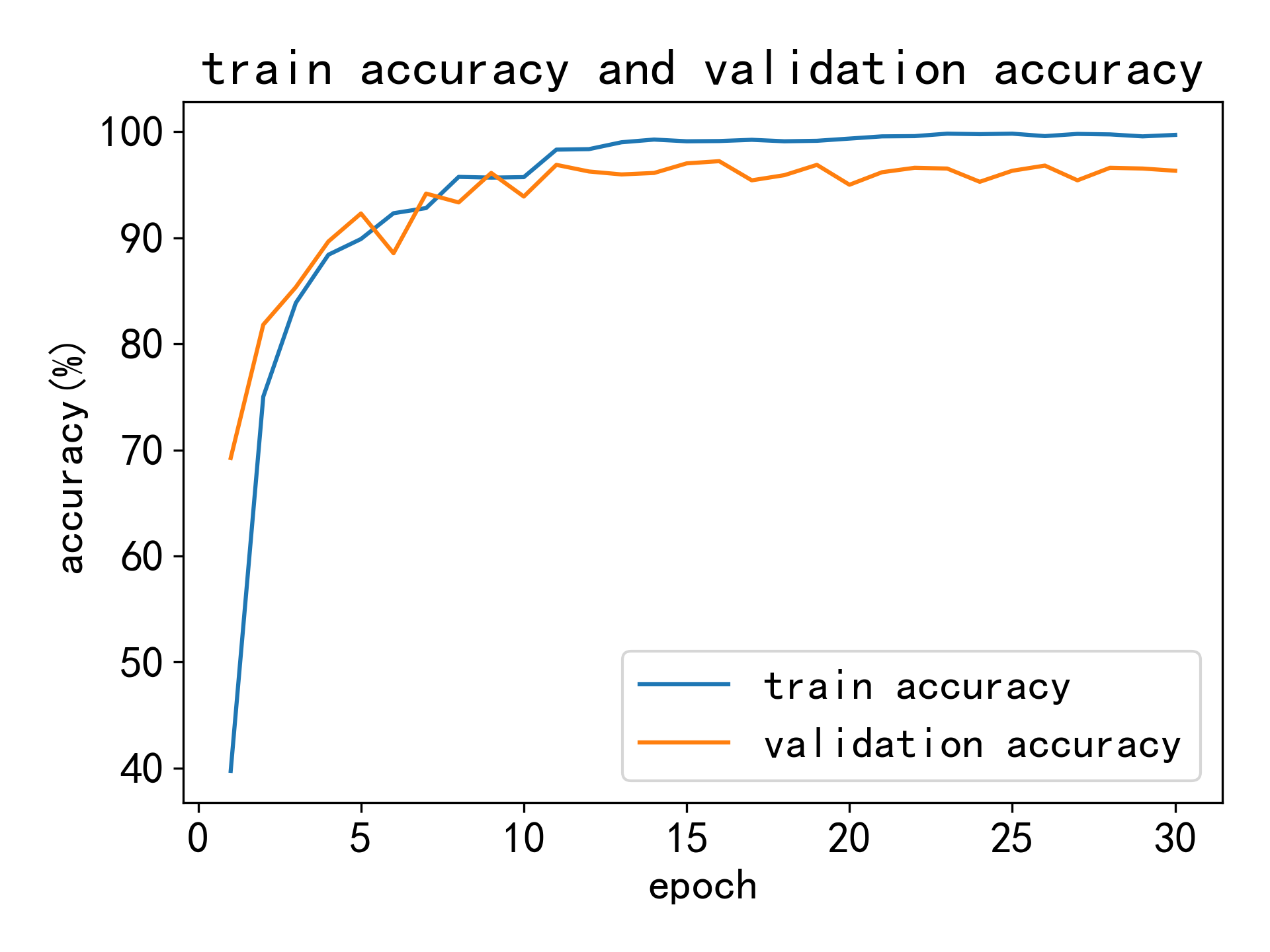
Loss curve of training set and verification set
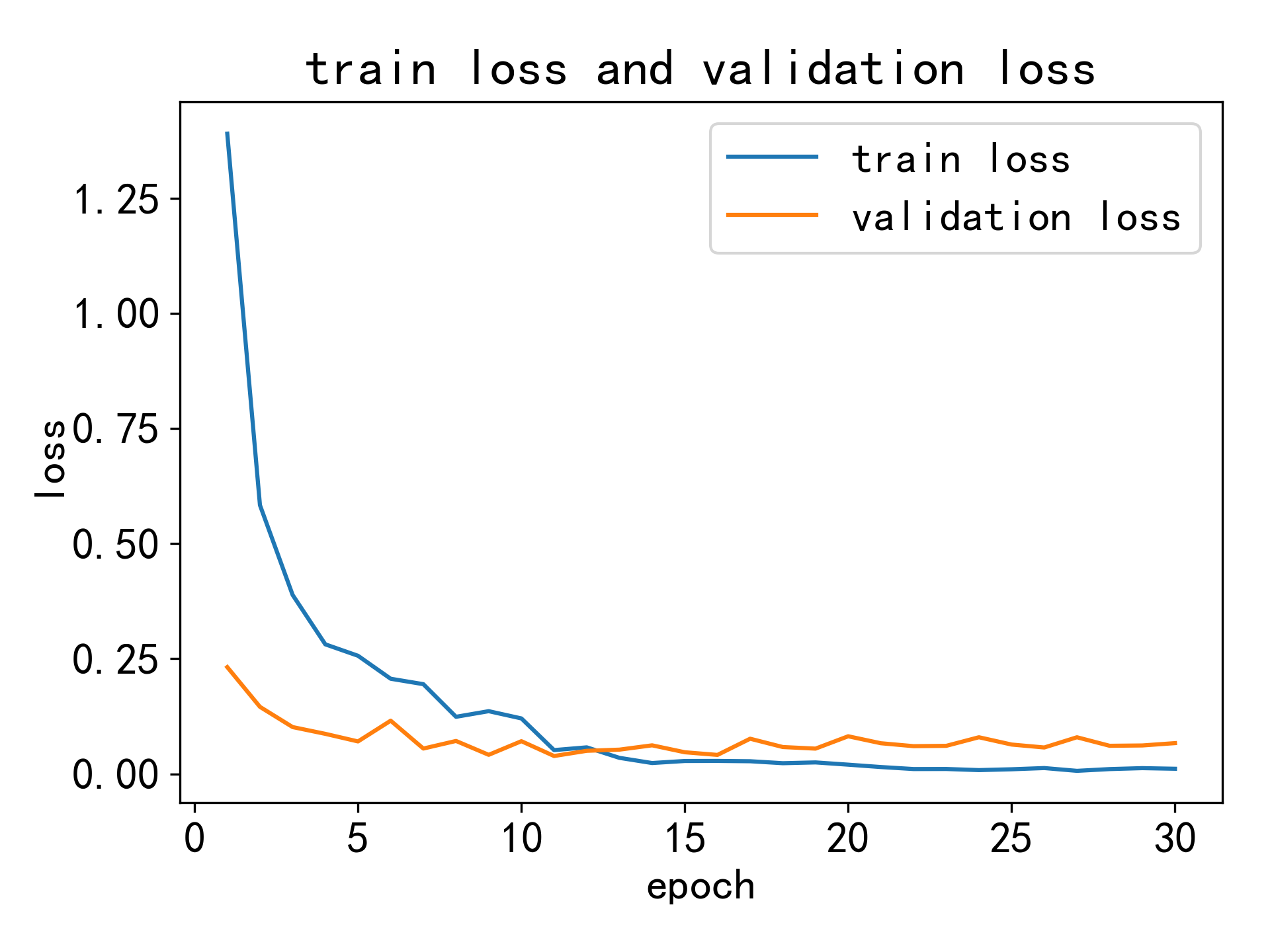
- Downloads last month
- 444